Advanced Cluster Based Spectrum Sensing in Broadband Cognitive Radio Network
T.Jothi 1 , P. Panimalar2
Section:Research Paper, Product Type: Journal Paper
Volume-07 ,
Issue-04 , Page no. 81-86, Feb-2019
Online published on Feb 28, 2019
Copyright © T.Jothi, P. Panimalar . This is an open access article distributed under the Creative Commons Attribution License, which permits unrestricted use, distribution, and reproduction in any medium, provided the original work is properly cited.
View this paper at Google Scholar | DPI Digital Library
How to Cite this Paper
- IEEE Citation
- MLA Citation
- APA Citation
- BibTex Citation
- RIS Citation
IEEE Citation
IEEE Style Citation: T.Jothi, P. Panimalar, “Advanced Cluster Based Spectrum Sensing in Broadband Cognitive Radio Network,” International Journal of Computer Sciences and Engineering, Vol.07, Issue.04, pp.81-86, 2019.
MLA Citation
MLA Style Citation: T.Jothi, P. Panimalar "Advanced Cluster Based Spectrum Sensing in Broadband Cognitive Radio Network." International Journal of Computer Sciences and Engineering 07.04 (2019): 81-86.
APA Citation
APA Style Citation: T.Jothi, P. Panimalar, (2019). Advanced Cluster Based Spectrum Sensing in Broadband Cognitive Radio Network. International Journal of Computer Sciences and Engineering, 07(04), 81-86.
BibTex Citation
BibTex Style Citation:
@article{Panimalar_2019,
author = {T.Jothi, P. Panimalar},
title = {Advanced Cluster Based Spectrum Sensing in Broadband Cognitive Radio Network},
journal = {International Journal of Computer Sciences and Engineering},
issue_date = {2 2019},
volume = {07},
Issue = {04},
month = {2},
year = {2019},
issn = {2347-2693},
pages = {81-86},
url = {https://www.ijcseonline.org/full_spl_paper_view.php?paper_id=726},
publisher = {IJCSE, Indore, INDIA},
}
RIS Citation
RIS Style Citation:
TY - JOUR
UR - https://www.ijcseonline.org/full_spl_paper_view.php?paper_id=726
TI - Advanced Cluster Based Spectrum Sensing in Broadband Cognitive Radio Network
T2 - International Journal of Computer Sciences and Engineering
AU - T.Jothi, P. Panimalar
PY - 2019
DA - 2019/02/28
PB - IJCSE, Indore, INDIA
SP - 81-86
IS - 04
VL - 07
SN - 2347-2693
ER -
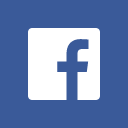
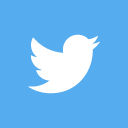
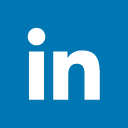
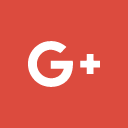
Abstract
A radio spectrum higher data rates is a challenging task that requires inventive advances prepared for giving better methodologies for using the open radio spectrum. The issue of applying the Cognitive radio technique successfully is how to sense exactly and quickly whether or not the Primary User (PU) exists, and searching for the spectrum holes to provide the Secondary User (SU). The proposed system focus on Advanced Cluster Based Spectrum Sensing (ACBSS) algorithm which combines hierarchical data-fusion idea with jointly compressive reconstruction technology. To validate the efficiency and effectiveness, we compare the ACBBSS with Independent Compressive Sensing (ICS) and Joint Compressive Sensing (JCS) in the detection probability, false-alarm probability and algorithm execution time under the situation of unusual SNR and compression ratio. The majority of existing work has focused on Single band Cognitive Radio, multiband cognitive radio represents great promises toward implementing efficient cognitive networks compared to single-based networks. This has primarily motivated the introduction of Multiband Cognitive Radio (MB-CR) paradigm, which is also referred to wideband CR. In addition, it helps contribute seamless handoff from band to band, which get better the link maintenance and reduces data transmission interruptions.
Key-Words / Index Term
Cluster, ICS, JCS, Cognitive Radio Technique, Single and Multiband, ACBSS
References
[1] Li, C. M., & Lu, S. H. (2016). Energy-based maximum likelihood spectrum sensing method for the cognitive radio. Wireless Personal Communications, 89(1), 289-302.
[2] Salahdine, F., Kaabouch, N., & Ghazi, H. E. (2018). One-Bit Compressive Sensing Vs. Multi-Bit Compressive Sensing for Cognitive Radio Networks. In IEEE Int. Conf. Industrial Techno(pp. 1-6).
[3] Manesh, M. R., Quadri, A., Subramaniam, S., & Kaabouch, N. (2017, January). An optimized SNR estimation technique using particle swarm optimization algorithm. In Computing and Communication Workshop and Conference (CCWC), 2017 IEEE 7th Annual (pp. 1-6). IEEE.
[4] Salahdine, F., & El Ghazi, H. (2017, October). A real time spectrum scanning technique based on compressive sensing for cognitive radio networks. In Ubiquitous Computing, Electronics and Mobile Communication Conference (UEMCON), 2017 IEEE 8th Annual (pp. 506-511). IEEE.
[5] Salahdine, F., Kaabouch, N., & El Ghazi, H. (2016, October). Bayesian compressive sensing with circulant matrix for spectrum sensing in cognitive radio networks. In Ubiquitous Computing, Electronics & Mobile Communication Conference (UEMCON), IEEE Annual (pp. 1-6). IEEE.
[6] Yarkan, S. (2015). A generic measurement setup for implementation and performance evaluation of spectrum sensing techniques: Indoor environments. IEEE Transactions on Instrumentation and Measurement, 64(3), 606-614.
[7] Sun, H., Nallanathan, A., Wang, C. X., & Chen, Y. (2013). Wideband spectrum sensing for cognitive radio networks: a survey. IEEE Wireless Communications, 20(2), 74-81.
[8] Sharma, S. K., Lagunas, E., Chatzinotas, S., & Ottersten, B. (2016). Application of compressive sensing in cognitive radio communications: A survey. IEEE Communication Surveys & Tutorials.
[9] Tian, Z., Tafesse, Y., & Sadler, B. M. (2012). Cyclic feature detection with sub-Nyquist sampling for wideband spectrum sensing. IEEE Journal of Selected topics in signal processing, 6(1), 58-69.
[10] Shahrasbi, B., & Rahnavard, N. (2013, December). A clustering-based coordinated spectrum sensing in wideband large-scale cognitive radio networks. In Global Communications Conference (GLOBECOM), 2013 IEEE (pp. 1101-1106). IEEE.
[11] Yadav, N., & Rathi, S. (2011). Spectrum Sensing Techniques: Research, Challenge and Limitations 1.
[12] E. Axell, G. Leus, E. Larsson, and H. Poor, “Spectrum sensing for cognitive radio : state-of-the-art and recent advances,” IEEE Signal Process. Mag., vol. 29, no. 3, p. 101-116, 2012.
[13] F. Salahdine, N. Kaabouch, and H. El Ghazi, “Techniques for dealing with uncertainty in cognitive radio networks,” IEEE Ann. Comput. Commun. Workshop Conf., p. 1-6, 2017.
[14] W. Jinlong et al., “Hierarchical cognition cycle for cognitive radio networks,” Commun., .108-121,2015.
[15] R. Tandra and A. Sahai, “SNR walls for signal detection,” Sel. Top. Signal Process. vol. 2,p. 4-17, 2008.