Document Clustering with Omni-Directional Data Similarity Process
K. Lakshmi1
Section:Research Paper, Product Type: Journal Paper
Volume-07 ,
Issue-04 , Page no. 87-90, Feb-2019
Online published on Feb 28, 2019
Copyright © K. Lakshmi . This is an open access article distributed under the Creative Commons Attribution License, which permits unrestricted use, distribution, and reproduction in any medium, provided the original work is properly cited.
View this paper at Google Scholar | DPI Digital Library
How to Cite this Paper
- IEEE Citation
- MLA Citation
- APA Citation
- BibTex Citation
- RIS Citation
IEEE Style Citation: K. Lakshmi, “Document Clustering with Omni-Directional Data Similarity Process,” International Journal of Computer Sciences and Engineering, Vol.07, Issue.04, pp.87-90, 2019.
MLA Style Citation: K. Lakshmi "Document Clustering with Omni-Directional Data Similarity Process." International Journal of Computer Sciences and Engineering 07.04 (2019): 87-90.
APA Style Citation: K. Lakshmi, (2019). Document Clustering with Omni-Directional Data Similarity Process. International Journal of Computer Sciences and Engineering, 07(04), 87-90.
BibTex Style Citation:
@article{Lakshmi_2019,
author = {K. Lakshmi},
title = {Document Clustering with Omni-Directional Data Similarity Process},
journal = {International Journal of Computer Sciences and Engineering},
issue_date = {2 2019},
volume = {07},
Issue = {04},
month = {2},
year = {2019},
issn = {2347-2693},
pages = {87-90},
url = {https://www.ijcseonline.org/full_spl_paper_view.php?paper_id=727},
publisher = {IJCSE, Indore, INDIA},
}
RIS Style Citation:
TY - JOUR
UR - https://www.ijcseonline.org/full_spl_paper_view.php?paper_id=727
TI - Document Clustering with Omni-Directional Data Similarity Process
T2 - International Journal of Computer Sciences and Engineering
AU - K. Lakshmi
PY - 2019
DA - 2019/02/28
PB - IJCSE, Indore, INDIA
SP - 87-90
IS - 04
VL - 07
SN - 2347-2693
ER -
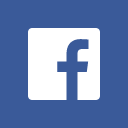
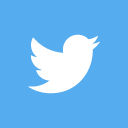
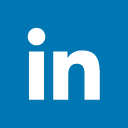
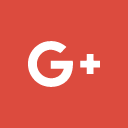
Abstract
Most of the clustering techniques must presume some cluster relationship relating to the data thing. Similarity among some items is usually defined clearly or sometimes absolutely. In this paper, is an introduction to some novel reference centered similarity gauge and two related clustering approaches. The significant difference between an old-fashioned dissimilarity/similarity gauge and the approach considered in this paper is how the former uses simple single standpoint. In the existing approach it considers the origin, while the latter utilizes a number of reference details, which are objects assumed not to ever be inside the same cluster while using two things being scored. Using several reference details, more useful assessment of similarity could be possibly achieved. In document clustering two qualification functions are proposed and is determined by the fresh measure. The above functions are being examined along with frequently used clustering based algorithms which use other well known similarity measures in various document collections in order to verify the approach under consideration in this paper.
Key-Words / Index Term
Document Clustering, Correlation Measure, Similarity Measure, Data Mining
References
[1] X. Wu, V. Kumar, J. Ross Quinlan, J. Ghosh, Q. Yang, H. Motoda, G. J. McLachlan, A. Ng, B. Liu, P. S. Yu, Z.-H. Zhou, M.steinbach,D.J.Hand,andD.Steinberg,―Top10algorithmsindata mining,‖Knowl. Inf. Syst., vol. 14, no. 1, pp. 1–37, 2007.
[2] HChimand X. Deng, ―Efficient phrase-based document similarity for clustering,‖ IEEE Trans. on Knowl. and Data Eng., vol. 20, no. 9, pp. 1217–1229,2008.
[3] D. Lee andJ. Lee, ―Dynamic dissimilarity measure for support based clustering,‖ IEEE Trans. on Knowl. and Data Eng., vol. 22, no. 6, pp. 900–905,2010.
[4] P. Lakkaraju, S. Gauch, andM. Speretta, ―Document similarity based on concept tree distance,‖ in Proc. of the 19th ACM conf. on Hypertext and hypermedia, 2008, pp.127–132.
[5] D. Ienco, R. G. Pensa, andR. Meo, ―Context-based distance learning for categorical data clustering,‖ in Proc. of the 8th Int. Symp. IDA, 2009, pp.83–94.
[6] I. Guyon, U.von Luxburg, and R. C. Williamson, ―Clustering: Science or Art?‖ NIPS‘09 Workshop on Clustering Theory,2009.
[7] E. Pekalska, A. Harol, R. P. W. Duin, B. Spillmann, and H. Bunke,―Non-Euclideanornon-metricmeasurescanbeinformative,‖ in Structural, Syntactic, and Statistical Pattern Recognition, ser. LNCS, vol. 4109, 2006, pp.871–880.
[8] M.Pelillo, ―What is a cluster?Perspectivesfromgametheory,‖in Proc. of the NIPS Workshop on Clustering Theory,2009.
[9] I. Dhillon and D. Modha, ―Concept decompositionsfor large sparse text data using clustering,‖ Mach. Learn., vol. 42, no. 1-2, pp. 143–175, Jan2001.
[10] S. Zhong, ―Efficient online spherical K-means clustering,‖in
IEEE IJCNN, 2005, pp. 3180–3185.