Bilevel Feature Extraction-Based Text Mining for Fault Diagnosis of Railway Systems
D.Keerthanaa 1 , C. Premila Rosy2
Section:Research Paper, Product Type: Journal Paper
Volume-07 ,
Issue-04 , Page no. 137-139, Feb-2019
Online published on Feb 28, 2019
Copyright © D.Keerthanaa, C. Premila Rosy . This is an open access article distributed under the Creative Commons Attribution License, which permits unrestricted use, distribution, and reproduction in any medium, provided the original work is properly cited.
View this paper at Google Scholar | DPI Digital Library
How to Cite this Paper
- IEEE Citation
- MLA Citation
- APA Citation
- BibTex Citation
- RIS Citation
IEEE Style Citation: D.Keerthanaa, C. Premila Rosy, “Bilevel Feature Extraction-Based Text Mining for Fault Diagnosis of Railway Systems,” International Journal of Computer Sciences and Engineering, Vol.07, Issue.04, pp.137-139, 2019.
MLA Style Citation: D.Keerthanaa, C. Premila Rosy "Bilevel Feature Extraction-Based Text Mining for Fault Diagnosis of Railway Systems." International Journal of Computer Sciences and Engineering 07.04 (2019): 137-139.
APA Style Citation: D.Keerthanaa, C. Premila Rosy, (2019). Bilevel Feature Extraction-Based Text Mining for Fault Diagnosis of Railway Systems. International Journal of Computer Sciences and Engineering, 07(04), 137-139.
BibTex Style Citation:
@article{Rosy_2019,
author = {D.Keerthanaa, C. Premila Rosy},
title = {Bilevel Feature Extraction-Based Text Mining for Fault Diagnosis of Railway Systems},
journal = {International Journal of Computer Sciences and Engineering},
issue_date = {2 2019},
volume = {07},
Issue = {04},
month = {2},
year = {2019},
issn = {2347-2693},
pages = {137-139},
url = {https://www.ijcseonline.org/full_spl_paper_view.php?paper_id=737},
publisher = {IJCSE, Indore, INDIA},
}
RIS Style Citation:
TY - JOUR
UR - https://www.ijcseonline.org/full_spl_paper_view.php?paper_id=737
TI - Bilevel Feature Extraction-Based Text Mining for Fault Diagnosis of Railway Systems
T2 - International Journal of Computer Sciences and Engineering
AU - D.Keerthanaa, C. Premila Rosy
PY - 2019
DA - 2019/02/28
PB - IJCSE, Indore, INDIA
SP - 137-139
IS - 04
VL - 07
SN - 2347-2693
ER -
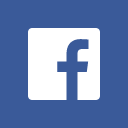
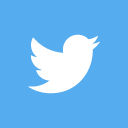
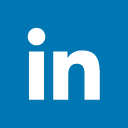
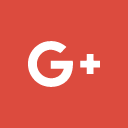
Abstract
A vast amount of text data is recorded in the forms of repair verbatim in railway maintenance sectors. Efficient text mining of such maintenance data plays an important role in detecting anomalies and improving fault diagnosis efficiency. However, unstructured verbatim, high-dimensional data, and imbalanced fault class distribution pose challenges for feature selections and fault diagnosis. We propose a bilevel feature extraction-based text mining that integrates features extracted at both syntax and semantic levels with the aim to improve the fault classification performance. We first perform an improved χ2 statistics-based feature selection at the syntax level to overcome the learning difficulty caused by an imbalanced data set. Then, we perform a prior latent Dirichlet allocation-based feature selection at the semantic level to reduce the data set into a low-dimensional topic space. Finally, we fuse fault features derived from both syntax and semantic levels via serial fusion. The proposed method uses fault features at different levels and enhances the precision of fault diagnosis for all fault classes, particularly minority ones. Its performance has been validated by using a railway maintenance data set collected from 2008 to 2014 by a railway corporation. It out performs traditional approaches.
Key-Words / Index Term
Bilevel, Feature Selection, Feature Extraction, Railway, Text Mining
References
[1] D. G. Rajpathak, “An ontology based text mining system for knowledge discovery from the diagnosis data in the automotive domain,” Comput.Ind., vol. 64, no. 5, pp. 565–580, Jun. 2013.
[2] W. Wang, H. Xu, and X. Huang, “Implicit feature detection via aconstrained topic model and SVM,” in Proc. Conf. Empirical Methods Natural Lang. Process., Seattle, WA, USA, 2013, pp. 903–907.
[3] L. Yin, Y. Ge, K. Xiao, X. Wang, and X. Quan, “Feature selection for high-dimensional imbalanced data,” Neuro computing, vol. 105, pp. 3–11,Apr. 2013.
[4] Z. Zhai, B. Liu, H. Xu, and P. Jia, “Constrained LDA for grouping productfeatures in opinion mining,” in Proc. 15th Pacific-Asia Conf. Adv. Knowl.Discov. Data Mining, Shenzhen, China, 2011, vol. 1, pp. 448–459.
[5] X. Ding, Q. He, and N. Luo, “A fusion feature and its improvementbased on locality preserving projections for rolling element bearingfault classification,” J. Sound Vibration, vol. 335, pp. 367–383,Jan. 2015.
[6] L. Huang and Y. L. Murphey, “Text mining with application to engineeringdiagnostics,” in Proc. 19th Int. Conf. IEA/AIE, Annecy, France, 2006,pp. 1309–1317.
[7] J. Silmon and C. Roberts, “Improving switch reliability with innovativecondition monitoring techniques,” Proc. IMechE, F C J. Rail RapidTransit, vol. 224, no. 4, pp. 293–302, 2010.
[8] D. Blei, A. Ng, and M. Jordan, “Latent Dirichlet allocation,” J. Mach.Learn. Res., vol. 3, pp. 993–1022, Jan. 2003.
[9] J. Chang, J. Boyd-Graber, C.Wang, S. Gerrish, and D. Blei, “Reading tealeaves: How humans interpret topic models,” Neural Inf. Process. Syst.,vol. 22, pp. 288–296, 2009.
[10] D. A. Cieslak and N. V. Chawla, “Learning decision trees for unbalanceddata,” in Proceedings of the 2008 European Conference on MachineLearning and Knowledge Discovery in Databases-Part I. Berlin,Germany: Springer-Verlag, 2008, pp. 241–256.
[11] T. Kailath, “The divergence and Bhattacharyya distance measures in signalselection,” IEEE Trans. Commun. Technol., vol. 15, no. 1, pp. 52–60,Feb. 1967.
[12] J. Yang, J. Yang, D. Zhang, and J. Lu, “Feature fusion: Parallel strategyvs. serial strategy,” Pattern Recognit., vol. 36, no. 6, pp. 1369–1381,Jun. 2003.
[13] C. Drummond and R. C. Holte, “C4. 5, class imbalanced, and cost sensitivity:Why under-sampling beats over-sampling,” in Proc. WorkshopLearn. Imbalanced Datasets II, ICML, Washington, DC, USA, 2003,pp. 1–8.
[14] X. Liu, J. Wu, and Z. Zhou, “Exploratory undersampling for classimbalancelearning,” IEEE Trans. Syst., Man Cybern., B, vol. 39, no. 2,pp. 539–550, Apr. 2009.
[15] D. Margineantu and T. G. Dietterich, “Learning decision trees for lossminimization in multi-class problems,” Dept. Comput. Sci., Oregon StateUniv., Corvallis, OR, USA, Tech. Rep., 1999.
[16] M. V. Joshi, R. Agarwal, and V. Kumar, “Predicting rare classes: Canboosting make any week learner strong?” in Proc. 8th ACM SIGKDDInt. Conf. Knowl. Discov. Data Mining, Edmonton, AB, Canada, 2002,pp. 297–306.
[17] Y. Tang, Y. Zhang, and N. V. Chawla, “SVMs modeling for highly imbalancedclassification,” IEEE Trans. Syst., Man Cybern., B, vol. 39, no. 1,pp. 281–288, Feb. 2009.
[18] G. Weiss, “Mining with rarity: A unifying framework,” ACM SIGKDDExplorations Newslett.—Spec. Issue Learn. Imbalanced Datasets, vol. 6,no. 1, pp. 7–19, Jun. 2004.
[19] D. Mladenic and M. Grobelnik, “Feature selection for unbalanced classdistribution and naive Bayes,” in Proc. 16th Int. Conf. Mach. Learn., Bled,Slovenia, 1999, pp. 258–267.
[20] Z. Zheng, X.Wu, and R. Srihari, “Feature selection for text categorizationon imbalanced data,” ACM SIGKDD Explorations Newslett.—Spec. IssueLearn. Imbalanced Datasets, vol. 6, no. 1, pp. 80–89, Jun. 2004.