A Survey on Earth Quakes Prediction Techniques with Clustering Methods
A. Mary Subashini1
Section:Survey Paper, Product Type: Journal Paper
Volume-07 ,
Issue-04 , Page no. 162-166, Feb-2019
Online published on Feb 28, 2019
Copyright © A. Mary Subashini . This is an open access article distributed under the Creative Commons Attribution License, which permits unrestricted use, distribution, and reproduction in any medium, provided the original work is properly cited.
View this paper at Google Scholar | DPI Digital Library
How to Cite this Paper
- IEEE Citation
- MLA Citation
- APA Citation
- BibTex Citation
- RIS Citation
IEEE Style Citation: A. Mary Subashini, “A Survey on Earth Quakes Prediction Techniques with Clustering Methods,” International Journal of Computer Sciences and Engineering, Vol.07, Issue.04, pp.162-166, 2019.
MLA Style Citation: A. Mary Subashini "A Survey on Earth Quakes Prediction Techniques with Clustering Methods." International Journal of Computer Sciences and Engineering 07.04 (2019): 162-166.
APA Style Citation: A. Mary Subashini, (2019). A Survey on Earth Quakes Prediction Techniques with Clustering Methods. International Journal of Computer Sciences and Engineering, 07(04), 162-166.
BibTex Style Citation:
@article{Subashini_2019,
author = {A. Mary Subashini},
title = {A Survey on Earth Quakes Prediction Techniques with Clustering Methods},
journal = {International Journal of Computer Sciences and Engineering},
issue_date = {2 2019},
volume = {07},
Issue = {04},
month = {2},
year = {2019},
issn = {2347-2693},
pages = {162-166},
url = {https://www.ijcseonline.org/full_spl_paper_view.php?paper_id=743},
publisher = {IJCSE, Indore, INDIA},
}
RIS Style Citation:
TY - JOUR
UR - https://www.ijcseonline.org/full_spl_paper_view.php?paper_id=743
TI - A Survey on Earth Quakes Prediction Techniques with Clustering Methods
T2 - International Journal of Computer Sciences and Engineering
AU - A. Mary Subashini
PY - 2019
DA - 2019/02/28
PB - IJCSE, Indore, INDIA
SP - 162-166
IS - 04
VL - 07
SN - 2347-2693
ER -
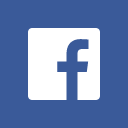
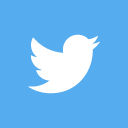
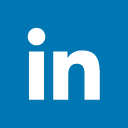
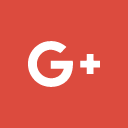
Abstract
The field of data mining has evolved from its roots in databases, statistics, artificial intelligence, information theory and algorithms into a core set of techniques that have been applied to a range of problems. Computational simulation and data acquisition in scientific and engineering domains have made tremendous progress over the past two decades. A mix of advanced algorithms, exponentially increasing computing power and accurate sensing and measurement devices have resulted in more data repositories. Advanced technologies in networks have enabled the communication of large volumes of data across the world.This paper aims at further data mining study on scientific data. This paper highlights the data mining techniques applied to mine for surface changes over time (e.g. Earthquake rupture). The data mining techniques help researchers to predict the changes in the intensity of volcanos. This paper uses predictive statistical models that can be applied to areas such as seismic activity , the spreading of fire. The basic problem in this class of systems is unobservable dynamics with respect to earthquakes. The space-time patterns associated with time, location and magnitude of the sudden events from the force threshold are observable. This paper highlights the observable space time earthquake patterns from unobservable dynamics using data mining techniques, pattern recognition and ensemble forecasting. Thus this paper gives insight on how data mining can be applied in finding the consequences of earthquakes and hence alerting thepublic.
Key-Words / Index Term
Earthquake, data mining techniques, space-time patterns
References
[1]. W.Dzwinel et al Non multidimensional scaling and visualization of earth quake cluster over space and feature space, nonlinear processes in geophysics 12[2005]pp1-12.
[2]. C.Lomnitz.FundamentalsofEarthquakeprediction[1994].
[3]. B.Gutenberg& C.H. Richtro, Earthquake magnitude, intensity, energy & acceleration bulseismsoc. Am 36, 105-145 [1996].
[4]. C.Brunk, J.Kelly&Rkohai “MinesetAn integrate system for Visual Data Mining” 1997.
[5]. MAdFraud: Investigating Ad Fraud in Android Applications.
[6]. Mining Personal Context-Aware Preferences for Mobile Users.
[7]. A Flexible Generative Model for Preference Aggregation.
[8]. D. M. Blei, A. Y. Ng, and M. I. Jordan. Lantentdirichlet allocation.Journal of Machine Learning Research, pages 993– 1022, 2003.
[9]. Y. Ge, H. Xiong, C. Liu, and Z.-H. Zhou. A taxi driving fraud detection system. In Proceedings of the 2011 IEEE 11th International Conference Neural Networks, ICNN ’11, pages 181–190, 2011.
[10]. N. Spirin and J. Han, “Survey on webspam detection: Principles and algorithms,” SIGKDD Explor. Newslett., vol. 13, no. 2, pp. 50–64,May2012.
[11]. E.-P. Lim, V.-A. Nguyen, N. Jindal, B. Liu, and H. W. Lauw, “Detecting product review spammers using rating behaviors,” in Proc.19thACMInt. Conf. Inform. Knowl.Manage., 2010, pp. 939–948.
[12]. G. Ateniese, R. Di Pietro, L. V. Mancini, and G. Tsudik,
[13]. “Scalable and efficient provable data possession,” in Proc.SecureComm, 2008, Art. ID 9.C. C. Erway, A. Küpçü, C. Papamanthou, and R.Tamassia, “Dynamic provable data possession,” in Proc. CCS,2009, pp. 213–222.
[14]. E. Esiner, A. Küpçü, and Ö. Özkasap, “Analysis and optimization on FlexDPDP: A practical solution for dynamic provable data possession,” Intelligent Cloud Computing(Lecture Notes in Computer Science), vol. 8993. Berlin, Germany: Springer-Verlag, 2014, pp. 65–83.
[15]. E. Zhou and Z. Li, “An improved remote data possession checking protocol in cloud storage,” in Algorithms andArchitectures for Parallel Processing (Lecture Notes ),105- 110.
[16] Opinion spam and analysis. In Proceedings of the 2008 International Conference on Web Search and Networking, WSDM ’08, pages 219–230, 2008.