User Web Access Record Mining for Business Intelligence
D. Kamalavadhanai1 , N. Aarthi2
Section:Research Paper, Product Type: Journal Paper
Volume-07 ,
Issue-04 , Page no. 187-189, Feb-2019
Online published on Feb 28, 2019
Copyright © D. Kamalavadhanai, N. Aarthi . This is an open access article distributed under the Creative Commons Attribution License, which permits unrestricted use, distribution, and reproduction in any medium, provided the original work is properly cited.
View this paper at Google Scholar | DPI Digital Library
How to Cite this Paper
- IEEE Citation
- MLA Citation
- APA Citation
- BibTex Citation
- RIS Citation
IEEE Citation
IEEE Style Citation: D. Kamalavadhanai, N. Aarthi, “User Web Access Record Mining for Business Intelligence,” International Journal of Computer Sciences and Engineering, Vol.07, Issue.04, pp.187-189, 2019.
MLA Citation
MLA Style Citation: D. Kamalavadhanai, N. Aarthi "User Web Access Record Mining for Business Intelligence." International Journal of Computer Sciences and Engineering 07.04 (2019): 187-189.
APA Citation
APA Style Citation: D. Kamalavadhanai, N. Aarthi, (2019). User Web Access Record Mining for Business Intelligence. International Journal of Computer Sciences and Engineering, 07(04), 187-189.
BibTex Citation
BibTex Style Citation:
@article{Kamalavadhanai_2019,
author = {D. Kamalavadhanai, N. Aarthi},
title = {User Web Access Record Mining for Business Intelligence},
journal = {International Journal of Computer Sciences and Engineering},
issue_date = {2 2019},
volume = {07},
Issue = {04},
month = {2},
year = {2019},
issn = {2347-2693},
pages = {187-189},
url = {https://www.ijcseonline.org/full_spl_paper_view.php?paper_id=749},
publisher = {IJCSE, Indore, INDIA},
}
RIS Citation
RIS Style Citation:
TY - JOUR
UR - https://www.ijcseonline.org/full_spl_paper_view.php?paper_id=749
TI - User Web Access Record Mining for Business Intelligence
T2 - International Journal of Computer Sciences and Engineering
AU - D. Kamalavadhanai, N. Aarthi
PY - 2019
DA - 2019/02/28
PB - IJCSE, Indore, INDIA
SP - 187-189
IS - 04
VL - 07
SN - 2347-2693
ER -
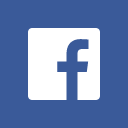
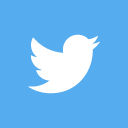
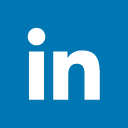
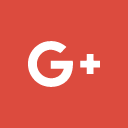
Abstract
User’s access records are captured by implementing a data mining algorithm on the website. User mostly browses those products in which he is interested. This system will capture user’s browsing pattern using data mining algorithm. This system is a web application where user can view various resources on the website. User will register their profile in an exchange of a password. User will get user ID and password in order to access the system. Once the user login’s to the system user will gain access to certain resources on the website. The links to the resources on the website have been modified such that a record of information about the access would be recorded in the database when clicked. This way, data mining can be performed on a relatively clean set of access records about the users. When user clicks on certain resources on the website his access records will be captured by the system this can be achieved with the help of data mining algorithm used in this system. By using this application, product based organization will get to know the demand for certain products. This system will help organization to target right consumers. This system will help product based firms to maintain good customer relationship. Hence, a good deal of business intelligence about the users’ behavior’s, preferences and about the popularities of the resources (products) on the website can be gained.
Key-Words / Index Term
User Access Record, Good Customer Relationship, Web Mining, User Behavior, Web Logs
References
[1]. Abraham, A (2001). Neuro-fuzzy systems: State-of-the- art modeling techniques, connectionist models of neurons, learning processes, and arti_cial intelligence. In Lecture Notes in Computer Science 2084, J Mira and APrieto (eds.), Germany, Spain: Springer-Verlag, pp. 269-276.
[2]. Cord_on, O, F Herrera, F Ho_mann and L Magdalena (2001). Genetic Fuzzy Systems: Evolutionary Tuning and Learning of Fuzzy Knowledge Bases. Singapore: World Scienti_c Publishing Company.
[3]. Hall, LO, IB Ozyurt and JC Bezdek (1999). Clustering with a genetically optimized approach. IEEE Transactions on Evolutionary Computation, 3(2), 103-112.
[4]. Pal, SK, V Talwar and P Mitra (2002). Web mining in soft computing framework: Relevance, state of the art and future directions. IEEE Transactions on Neural Networks, Vol. 13, No. 5, pp. 1163-1177.
[5]. Paliouras, G, C Papatheodorou, V Karkaletsisi and CD Spyropoulous (2000). Clustering the users of large websites into communities. In Proc. of the 17th International Conference on Machine Learning (ICML`00), pp. 719-726. USA: Morgan Kaufmann.
[6]. Pazzani, M and D Billsus (1997). Learning and revising user pro_les: The identi_cation of interesting web sites. Machine Learning, 27, 313-331.
[7]. Spiliopoulou, M and LC Faulstich (1999). WUM: A web utilization miner. In Proc. of EDBT Workshop on the Web and Data Bases (WebDB`98), pp. 109-115. Springer Verlag.
[8]. Srivastava, J, R Cooley,MDeshpande and PN Tan (2000). Web usage mining: Discovery and applications of usage patterns from web data SIGKDD Explorations, 1(2), 12-23.
[9]. Wang, X, A Abraham and KA Smith (2002). Soft computing paradigms for web access pattern analysis. In Proc.of the 1st International Conference on Fuzzy Systems and Knowledge Discovery, pp. 631-635.
[10]. Yang, Q and HH Zhang (2003). Web-log mining for predictive web caching. IEEE Transactions on Knowledge and Data Engineering, Vol. 15, No. 4, pp. 1050-1053.