Predicting Ticket Sales Using Web-Based External Factors and Box-Office Data
M. Keerthana1 , J. Rabacca Cinthiya2
Section:Research Paper, Product Type: Journal Paper
Volume-07 ,
Issue-04 , Page no. 310-312, Feb-2019
Online published on Feb 28, 2019
Copyright © M. Keerthana, J. Rabacca Cinthiya . This is an open access article distributed under the Creative Commons Attribution License, which permits unrestricted use, distribution, and reproduction in any medium, provided the original work is properly cited.
View this paper at Google Scholar | DPI Digital Library
How to Cite this Paper
- IEEE Citation
- MLA Citation
- APA Citation
- BibTex Citation
- RIS Citation
IEEE Style Citation: M. Keerthana, J. Rabacca Cinthiya, “Predicting Ticket Sales Using Web-Based External Factors and Box-Office Data,” International Journal of Computer Sciences and Engineering, Vol.07, Issue.04, pp.310-312, 2019.
MLA Style Citation: M. Keerthana, J. Rabacca Cinthiya "Predicting Ticket Sales Using Web-Based External Factors and Box-Office Data." International Journal of Computer Sciences and Engineering 07.04 (2019): 310-312.
APA Style Citation: M. Keerthana, J. Rabacca Cinthiya, (2019). Predicting Ticket Sales Using Web-Based External Factors and Box-Office Data. International Journal of Computer Sciences and Engineering, 07(04), 310-312.
BibTex Style Citation:
@article{Keerthana_2019,
author = {M. Keerthana, J. Rabacca Cinthiya},
title = {Predicting Ticket Sales Using Web-Based External Factors and Box-Office Data},
journal = {International Journal of Computer Sciences and Engineering},
issue_date = {2 2019},
volume = {07},
Issue = {04},
month = {2},
year = {2019},
issn = {2347-2693},
pages = {310-312},
url = {https://www.ijcseonline.org/full_spl_paper_view.php?paper_id=778},
publisher = {IJCSE, Indore, INDIA},
}
RIS Style Citation:
TY - JOUR
UR - https://www.ijcseonline.org/full_spl_paper_view.php?paper_id=778
TI - Predicting Ticket Sales Using Web-Based External Factors and Box-Office Data
T2 - International Journal of Computer Sciences and Engineering
AU - M. Keerthana, J. Rabacca Cinthiya
PY - 2019
DA - 2019/02/28
PB - IJCSE, Indore, INDIA
SP - 310-312
IS - 04
VL - 07
SN - 2347-2693
ER -
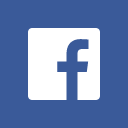
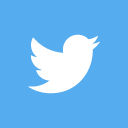
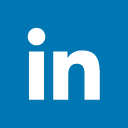
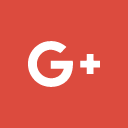
Abstract
Posting online reviews and rating their satisfaction purchased products has become an increasingly popular way to share the information for anonymous candidates who has interest in purchasing the product. In addition, people leave their interests and near-future purchasing plan on the web such as search history and search query volume. From this phenomenon, the prediction of sales performance is possible in many products by mining the data sets which are left on the web by consumers’ online activities. In this paper, we focused on the movie ticket sales which word-of-mouth effect is prominent, and our goal is to forecast the sales performance of the near-weekend using box-office data and external factors such as online reviews, star ratings and search volume. For this work, we gather 1.7 million online reviews and movie ratings, and we also gather the daily search volume of movies’ title for past three years. Using machine learning techniques and linear modeling, we develop a model for high-accuracy predicting of ticket sales on near-future. We also analyze a relationship between ticket sales performance on weekends and box-office data, online reviews, star ratings, and search volume. Through this work, we support to decide the ideal number of screens for a given weekend, thus it contributes to a substantial increase in the rate of profit on movie markets.
Key-Words / Index Term
Box-Office Data, Online Reviews, Star Ratings, Ticket Sales
References
[1] W. Duan, B. Gu, and A. B. Whinston, “Do online reviews matter ?An empirical investigation of panel data,” vol. 45, pp. 1007–1016, 2008.
[2] S. Goel, J. M. Hofman, S. Lahaie, D. M. Pennock, and D. J. Watts, “What Can Search Predict ?”WWW ’10, 2010.
[3] X. Yu, Y. Liu, X. Huang, and A. An, “Mining Online Reviews for Predicting Sales Performance: A Case Study in the Movie Domain,” IEEE Transactions on Knowledge and Data Engineering, vol. 24, no. 4, pp. 720–734, Apr. 2012. [Online]. Available: http: //ieeexplore.ieee.org/lpdocs/epic03/wrapper.htm?arnumber=5677530
[4] G. Kulkarni, P. K. Kannan, and W. Moe, “Using online search data to forecast new product sales,” Decision Support Systems, vol. 52, no. 3, pp. 604–611, 2012.[Online]. Available: http: //dx.doi.org/10.1016/j.dss.2011.10.017
[5] L. Zhang, J. Luo, and S. Yang, “Forecasting box office revenue of movies with BP neural network,” Expert Systems with Applications, vol. 36, no. 3, pp. 6580–6587, Apr. 2009. [Online]. Available: http://linkinghub.elsevier.com/retrieve/pii/S095741740800496X
[6] K. J. Lee and W. Chang, “Bayesian belief network for box-office performance: A case study on Korean movies,” Expert Systems withApplications, vol. 36, no. 1, pp. 280–291, Jan. 2009. [Online]. Available: http://linkinghub.elsevier.com/retrieve/pii/S0957417407004228
[7] MPAA. 2012 theatrical statistics summary.[Online]. Available: http:// www.mpaa.org/resources/3037b7a4-58a2-4109-8012-58fca3abdf1b.pdf
[8] R. Sharda and D. Delen, “Predicting box-office success of motion pictures with neural networks,” Expert Systems with Applications, vol. 30, no. 2, pp. 243–254, Feb. 2006. [Online]. Available: http://linkinghub.elsevier.com/retrieve/pii/S0957417405001399
[9] E. V. Karniouchina, “Impact of star and movie buzz on motion picture distribution and box office revenue,” International Journal of Researchin Marketing, vol. 28, no. 1, pp. 62–74, Mar. 2011. [Online]. Available: http://linkinghub.elsevier.com/retrieve/pii/S0167811610000881
[10] H. Rui, Y. Liu, and A. Whinston, “Whose and what chatter matters? The effect of tweets on movie sales,” Decision SupportSystems, vol. 55, no. 4, pp. 863–870, Nov. 2013.[Online]. Available: http://linkinghub.elsevier.com/retrieve/pii/S0167923612003880
[11] J. Du, H. Xu, and X. Huang, “Box office prediction based on microblog,” Expert Systems with Applications, vol. 41, no. 4, pp. 1680–1689, Mar. 2014. [Online]. Available: http://linkinghub.elsevier.com/retrieve/pii/S0957417413006866
[12] S. Moon, P. K. Bergey, and D. Iacobucci, “Dynamic effects among movie ratings, movie revenues, and viewer satisfaction,” Journal ofMarketing, vol. 74, no. 1, pp. 108–121, 2010.
[13] H. Drucker, C. J. Burges, L. Kaufman, A. Smola, and V. Vapnik, “Support vector regression machines,” Advances in neural informationprocessing systems, vol. 9, pp. 155–161, 1997.
[14] I. Basheer and M. Hajmeer, “Artificial neural networks: fundamentals, computing, design, and application,” Journal of microbiological methods, vol. 43, no. 1, pp. 3–31, 2000.