Appraisal Evaluation Tool for College Staff
N. Brindha1 , P. Dhivya2
Section:Survey Paper, Product Type: Journal Paper
Volume-07 ,
Issue-04 , Page no. 316-319, Feb-2019
Online published on Feb 28, 2019
Copyright © N. Brindha, P. Dhivya . This is an open access article distributed under the Creative Commons Attribution License, which permits unrestricted use, distribution, and reproduction in any medium, provided the original work is properly cited.
View this paper at Google Scholar | DPI Digital Library
How to Cite this Paper
- IEEE Citation
- MLA Citation
- APA Citation
- BibTex Citation
- RIS Citation
IEEE Citation
IEEE Style Citation: N. Brindha, P. Dhivya, “Appraisal Evaluation Tool for College Staff,” International Journal of Computer Sciences and Engineering, Vol.07, Issue.04, pp.316-319, 2019.
MLA Citation
MLA Style Citation: N. Brindha, P. Dhivya "Appraisal Evaluation Tool for College Staff." International Journal of Computer Sciences and Engineering 07.04 (2019): 316-319.
APA Citation
APA Style Citation: N. Brindha, P. Dhivya, (2019). Appraisal Evaluation Tool for College Staff. International Journal of Computer Sciences and Engineering, 07(04), 316-319.
BibTex Citation
BibTex Style Citation:
@article{Brindha_2019,
author = {N. Brindha, P. Dhivya},
title = {Appraisal Evaluation Tool for College Staff},
journal = {International Journal of Computer Sciences and Engineering},
issue_date = {2 2019},
volume = {07},
Issue = {04},
month = {2},
year = {2019},
issn = {2347-2693},
pages = {316-319},
url = {https://www.ijcseonline.org/full_spl_paper_view.php?paper_id=780},
publisher = {IJCSE, Indore, INDIA},
}
RIS Citation
RIS Style Citation:
TY - JOUR
UR - https://www.ijcseonline.org/full_spl_paper_view.php?paper_id=780
TI - Appraisal Evaluation Tool for College Staff
T2 - International Journal of Computer Sciences and Engineering
AU - N. Brindha, P. Dhivya
PY - 2019
DA - 2019/02/28
PB - IJCSE, Indore, INDIA
SP - 316-319
IS - 04
VL - 07
SN - 2347-2693
ER -
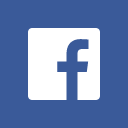
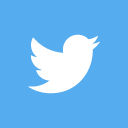
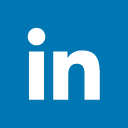
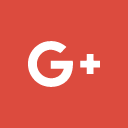
Abstract
Appraisal is an expert estimate of the value of something. Staff appraisal can be tricky. If you get this wrong, you will negatively impact your staff. This intranet web application will provide the feasible solution to boost the production of each of your staffs, regardless of their current achievement level. An effective staff appraisal system ought to validate by the students of the department. This aspect of the performance appraisal process will begin anew unless the employee is scheduled to participate in the Supervisory Performance Appraisal process or the process is warranted as a result of the Performance Growth Plan, or the staff’s job description has changed significantly. The evaluation cycle outlined below provides the employer with the opportunity to assess and evaluate the performance of the teacher on the district-adopted teacher performance evaluation criteria. Throughout the course of the evaluation cycle, strengths and areas of growth will be identified and communicated to staff. The assessment tool used for staff contains criteria like specific behaviours, knowledge, presentation skills that pertain to all staff. It is assumed that all staffs of the college are professional and, as such, will perform duties with integrity, and maintain a positive, vigilant attitude toward student physical safety and emotional well being.
Key-Words / Index Term
Appraisal System, Supervisory Performance, Svm, College Staff, Evaluation Tool
References
[1] H. Vogt, “On the mechanism of the anode effect in aluminium electrolysis,” Metallurgical & Materials Transactions B, vol. 31, pp 1225–1230, December 2000.
[2] K. Zhou, Z. Lin, D. Yu, B. Cao, Z. Wang, et al., “Cell resistance slope combined with LVQ neural network for prediction of a nodeeffect,” Sixth International Conference on Intelligent Control and Information Processing (ICICIP), pp 47-51, November 2015.
[3] N.A.A. Majid, M.P. Taylor, J.J.J. Chen and B.R. Young, “Multivariate statistical monitoring of the aluminium smelting process,” Computers & Chemical Engineering, vol. 35, pp 2457-2468, November 2011.
[4] S. Zeng, L. Ding, “Frequency characteristic and its application of the cell resistance in aluminum electrolysis,” Seventh International Conference on Computer Science &Education(ICCSE), pp 165-168, July 2012.
[5] J. Li, H. Wu, J. Pian, “The application of the equipment fault diagnosis based on modified Elman neural network,” International Conference on Electronic and Mechanical Engineering and Information Technology, vol. 8, pp 4135-4137, August 2011.
[6] J. Li, F. Qiao, T. Guo, “Neural network fault prediction and its application,” 8th World Congress on Intelligent Control and Automation (WCICA), pp 740-743, July 2010.
[7] A. Meghlaoui, J. Thibault, R.T. Buia, L. Tikasza, R. Santerre, “Neural networks for the identification of the aluminium electrolysis process,” Computers & Chemical Engineering, vol. 22, pp 1419-1428, September 1998.
[8] K. Zhou, D. Yu, Z. Lin, S. Guo, “Anode effect prediction of aluminium electrolysis using GRNN,” Chinese Automation Congress (CAC), pp 853–858, November 2015.
[9] J. Yi, D. Huang, S. Fu, H. He, T. Li, “Optimized Relative Transformation Matrix Using Bacterial Foraging Algorithm for Process Fault Detection,“ IEEE Transactions on Industrial Electronics, vol. 63, pp 2595-2605, 2016.
[10] N.A.A. Majid, M.P. Taylor, J.J.J. Chen, Y. Wei, B.R. Young, “Diagnosing faults in aluminium processing by using multivariate statistical approaches, Journal of Materials Science,” vol. 47, pp 1268- 1279, February 2012.
[11] N.A.A. Majid, M.P. Taylor, J.J.J. Chen, M.A. Stam, A. Mulder, B.R. Young, “Aluminium process fault detection by Multiway Principal Component Analysis,” Control Engineering Practice, vol. 19, pp 367- 379, April 2011.
[12] L. Qu, H. Zhou, “The Multi-class SVM Is Applied in Transformer Fault Diagnosis,” 14th International Symposium on Distributed Computing and Applications for Business Engineering and Science, pp 477-480, 2015.
[13] C. Zanchettin, B.L.D. Bezerra, W.W. Azevedo, “A KNN-SVM hybrid model for cursive handwriting recognition,” International Joint Conference on Neural Networks (IJCNN), pp 1-8, June 2012.
[14] S. Shang, M. Shi, W. Shang, Z. Hong, “Improved Feature Weight Algorithm and Its Application to Text Classification,“ Mathematical Problems in Engineering, March 2016.
[15] D. You, X. Gao, S. Katayama, “WPD-PCA-based laser welding process monitoring and defects diagnosis by using FNN and SVM,” IEEE Transactions on Industrial Electronics, vol. 62, pp 628-636, January 2015.
[16] J. Li, Q. Zhang, K. Wang, J. Wang, T. Zhou, et al., “Optimal dissolved gas ratios selected by genetic algorithm for power transformer fault diagnosis based on support vector machine,” IEEE Transactions on Dielectrics & Electrical Insulation, vol. 23, pp 1198-1206, 2016.
[17] L. Ren, W. Lv, S.W. Jiang, Y. Xiao, “Fault Diagnosis Using a Joint Model Based on Sparse Representation and SVM,” IEEE Transactions on Instrumentation & Measurement, vol. 65, pp 2313-2320, October 2016.