Survey on Machine Learning Algorithms for Classification and Prediction of Land Use Changes Using GIS
N. Bharanikumar1 , P. Dhanalakshmi2
Section:Survey Paper, Product Type: Journal Paper
Volume-07 ,
Issue-04 , Page no. 351-354, Feb-2019
Online published on Feb 28, 2019
Copyright © N. Bharanikumar, P. Dhanalakshmi . This is an open access article distributed under the Creative Commons Attribution License, which permits unrestricted use, distribution, and reproduction in any medium, provided the original work is properly cited.
View this paper at Google Scholar | DPI Digital Library
How to Cite this Paper
- IEEE Citation
- MLA Citation
- APA Citation
- BibTex Citation
- RIS Citation
IEEE Citation
IEEE Style Citation: N. Bharanikumar, P. Dhanalakshmi, “Survey on Machine Learning Algorithms for Classification and Prediction of Land Use Changes Using GIS,” International Journal of Computer Sciences and Engineering, Vol.07, Issue.04, pp.351-354, 2019.
MLA Citation
MLA Style Citation: N. Bharanikumar, P. Dhanalakshmi "Survey on Machine Learning Algorithms for Classification and Prediction of Land Use Changes Using GIS." International Journal of Computer Sciences and Engineering 07.04 (2019): 351-354.
APA Citation
APA Style Citation: N. Bharanikumar, P. Dhanalakshmi, (2019). Survey on Machine Learning Algorithms for Classification and Prediction of Land Use Changes Using GIS. International Journal of Computer Sciences and Engineering, 07(04), 351-354.
BibTex Citation
BibTex Style Citation:
@article{Bharanikumar_2019,
author = {N. Bharanikumar, P. Dhanalakshmi},
title = {Survey on Machine Learning Algorithms for Classification and Prediction of Land Use Changes Using GIS},
journal = {International Journal of Computer Sciences and Engineering},
issue_date = {2 2019},
volume = {07},
Issue = {04},
month = {2},
year = {2019},
issn = {2347-2693},
pages = {351-354},
url = {https://www.ijcseonline.org/full_spl_paper_view.php?paper_id=788},
publisher = {IJCSE, Indore, INDIA},
}
RIS Citation
RIS Style Citation:
TY - JOUR
UR - https://www.ijcseonline.org/full_spl_paper_view.php?paper_id=788
TI - Survey on Machine Learning Algorithms for Classification and Prediction of Land Use Changes Using GIS
T2 - International Journal of Computer Sciences and Engineering
AU - N. Bharanikumar, P. Dhanalakshmi
PY - 2019
DA - 2019/02/28
PB - IJCSE, Indore, INDIA
SP - 351-354
IS - 04
VL - 07
SN - 2347-2693
ER -
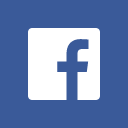
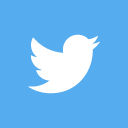
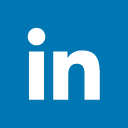
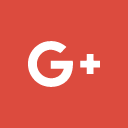
Abstract
Large area land-cover monitoring scenarios, involving large volumes of data, are becoming more prevalent in remote sensing applications. Thus, there is a pressing need for increased automation in the change mapping process the land transformation Model (LTM), which couples geographic information systems (GIS) with artificial neural networks. The objective of this research presents the survey report based on compare the performance of three machine learning algorithms (MLAs) and prediction of land use changes in GIS. The change map generated using ARTMAP has similar accuracies to a human-interpreted map produced by the U.S. Forest Service in the southern study area (John Rogan et al 2007). ARTMAP appears to be robust and accurate for automated, large area change monitoring as it performed equally well across the diverse study areas with minimal human intervention in the classification process. GIS is used to develop the spatial, predictor drivers and perform spatial analysis on the results. The predictive ability of the model improved at larger scales when assessed using a moving scalable window metric. the individual contribution of each predictor variable was examined and shown to vary across spatial scales.At the smallest scales, quality views were the strongest predictor variable. We interpreted the multi-scale influences of land use change, illustrating the relative influences of site (e.g. quality of views, residential streets) and situation (e.g. highways and county roads) variables at different scales.
Key-Words / Index Term
Component, Formatting, Style, Styling, Insert (key words)
References
[1] Alig, R. J. (1986). Econometric analysis of the factors influencing forest acreage trends in the southeast. Forest Science,32, 119-114.
[2] Alig, R. J., & Healy, R. G. (1987). Urban and built-up land area changes in the United States: an empirical investigation of determinants. Land Economics, 63(3), 216–226.
[3] Anderson, J. R., Hardy, E. E., Roach, J. T., & Witmer, R. E. 1976). A land use and land cover classification system for use with remote sensor data. US Geological Survey, Professional Paper 964, p. 28, Reston, VA.
[4] Atkinson, P., & Tatnall, A. (1997). Neural networks in remote sensing. International Journal of RemoteSensing, 18(4), 699–709.
[5] Babaian, R., Miyashita, H., Evans, R., Eshenbach, A., & Ramimrez, E. (1997). Early detection program for prostate cancer: results and identification of high-risk patient population. Urology, 37(3), 193–197.
[6] Batty, M., & Longley, (1994). Urban modeling in computer-graphic and geographic information system environments. Environment and Planning B, 19, 663–688.
[7] Bingham, G., Bishop, R., Brody, M., Bromley, D., Clark, E., Cooper, W., Costanza, R., Hale, T., Hayden, DeFries, R. S., & Chan, J. (2000).
[8] Multiple criteria for evaluating machine learning algorithms for land-cover classification from satellite data. Remote Sensing of Environment, 74, 503−515.
[9] Dobson, J., & Bright, E. (1994). Largearea change analysis: The Coast-watch Change analysis Project (C-CAP). Proceedings of Pecora 12, 24–26 August 1993, Sioux Falls, South Dakota (pp. 73−81).
[10] Foody, G. M., & Arora, M. K. (1997). Evaluation of some factors affecting the accuracy of classification by an artificial neural network. International Journal of Remote Sensing, 18, 799−810.
[11] Foody, G.M. (2003). Uncertainty, knowledge discovery and data mining in GIS. Progress in Physical Geography, 27(1), 113−121.
[12] Franklin, J. (1998). Predicting the distribution of shrub species in southern California from climate and terrainderived variables. Journal of Vegetation Science, 9, 733−748.
[13] G., Kellert, S., Norgaard, R., Norton, B., Payne, J., Russell, C., & Suter II, G.(1995). Issues in ecosystem valuation: improving information for decision making. Ecological Economics, 14, 73– 90.
[14] Bockstael, N., Costanza, R., Strand, I., Boyton, W., Bell, K., & Wagner, L. (1995). Ecological economic modeling and valuation of ecosystems. Ecological Economics, 14, 143–159.
[15] Boutt, D. F., Hyndman, D. W., Pijanowski, B. C., & Long, D. T. (2001). Identifying potential land usederived solute sources to stream baseflow using ground water models and GIS. Groundwater, 39(1),24–34.
[16] Brown, D. G., Duh, J. D., & Drzyzga, S. (2000). Estimating error in an analysis of forest fragmentation change using North American Landscape Characterization (NALC) Data. Remote Sensing of Environment, 71, 106–117.
[17] Gopal, S., & Woodcock, C. E. (1996). Remote sensing of forest change using artificial neural networks. IEEE Transactions on Geoscience and Remote Sensing, 34, 398−404.