Survey on Feature Selection Techniques towards Text Mining in Cloud
Balavinothini 1 , Gnanambigai 2
Section:Survey Paper, Product Type: Journal Paper
Volume-07 ,
Issue-05 , Page no. 101-105, Mar-2019
CrossRef-DOI: https://doi.org/10.26438/ijcse/v7si5.101105
Online published on Mar 10, 2019
Copyright © Balavinothini, Gnanambigai . This is an open access article distributed under the Creative Commons Attribution License, which permits unrestricted use, distribution, and reproduction in any medium, provided the original work is properly cited.
View this paper at Google Scholar | DPI Digital Library
How to Cite this Paper
- IEEE Citation
- MLA Citation
- APA Citation
- BibTex Citation
- RIS Citation
IEEE Style Citation: Balavinothini, Gnanambigai, “Survey on Feature Selection Techniques towards Text Mining in Cloud,” International Journal of Computer Sciences and Engineering, Vol.07, Issue.05, pp.101-105, 2019.
MLA Style Citation: Balavinothini, Gnanambigai "Survey on Feature Selection Techniques towards Text Mining in Cloud." International Journal of Computer Sciences and Engineering 07.05 (2019): 101-105.
APA Style Citation: Balavinothini, Gnanambigai, (2019). Survey on Feature Selection Techniques towards Text Mining in Cloud. International Journal of Computer Sciences and Engineering, 07(05), 101-105.
BibTex Style Citation:
@article{_2019,
author = {Balavinothini, Gnanambigai},
title = {Survey on Feature Selection Techniques towards Text Mining in Cloud},
journal = {International Journal of Computer Sciences and Engineering},
issue_date = {3 2019},
volume = {07},
Issue = {05},
month = {3},
year = {2019},
issn = {2347-2693},
pages = {101-105},
url = {https://www.ijcseonline.org/full_spl_paper_view.php?paper_id=814},
doi = {https://doi.org/10.26438/ijcse/v7i5.101105}
publisher = {IJCSE, Indore, INDIA},
}
RIS Style Citation:
TY - JOUR
DO = {https://doi.org/10.26438/ijcse/v7i5.101105}
UR - https://www.ijcseonline.org/full_spl_paper_view.php?paper_id=814
TI - Survey on Feature Selection Techniques towards Text Mining in Cloud
T2 - International Journal of Computer Sciences and Engineering
AU - Balavinothini, Gnanambigai
PY - 2019
DA - 2019/03/10
PB - IJCSE, Indore, INDIA
SP - 101-105
IS - 05
VL - 07
SN - 2347-2693
ER -
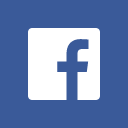
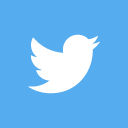
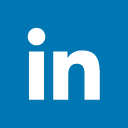
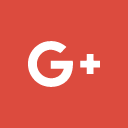
Abstract
Cloud computing is a technology that provides efficient services to the users over internet. Users stores volumes of data in cloud which is rendered as data as a service (DaaS) on demand and charged as per usage. Text mining is a technology that is used to retrieve data from a massive set of database. Cloud uses Text mining to retrieve data efficiently from various cloud data centres. Text classification is a technique used for discovering classes of indefinite data. Prior to applying any mining technique, trivial features should be filtered. Feature selection is capable of improving learning process, lesser computational complexity, organizes better general models, and decreasing required storage. We analyses towards effectiveness of the clustering based feature selection method. This paper is to analysis on different techniques used for feature selection. Further survey on Feature selection and Feature extraction technique has been extract the features from the documents, which results in single and multi-label document classification. Based on the extracted features the survey is done on multiple-feature based projective nonnegative matrix factorization technique to cluster the documents.
Key-Words / Index Term
Data mining, Feature selection, Text mining, Filtering, factorization
References
[1] Lin Yue, Wanli Zuo , TaoPeng , YingWang, Xuming Han A fuzzy document clustering approach based on domain-specified ontology, “ Data & Knowledge Engineering”, 100 (2015) 148-166.
[2] Malik Tahir Hassana, Asim Karim, Jeong-Bae Kim, Moongu Jeon CDIM: Document Clustering by Discrimination Information Maximization, ”Information Sciences”, 316 (2015) 87–106.
[3] Charlotte Laclau, Mohamed Nadif "Hard and fuzzy diagonal co-clustering for document-term partitioning "Neurocomputing”, 193 (2016) 133–147
[4] Vıctor Mijangos, Gerardo Sierra, Azucena Montes Sentence level matrix representation for document spectral clustering "Pattern Recognition Letters, Elsevier”, 20 November 2016.
[5] Mei Lua, Xiang-Jun Zhao, Li Zhang, Fan-Zhang Li, Semi-supervised concept factorization for document clustering, “Information Sciences”, 331 (2016) 86–98.
[6] Tingting Wei, Yonghe Lu, Huiyou Chang, Qiang Zhou, Xianyu Bao, A semantic approach for text clustering using WordNet and lexical chains, “Expert Systems with Applications”, 42 (2015) 2264–2275.
[7] Sourav Dutta, Gerhard Weikum, Cross-Document Co-Reference Resolution using Sample-Based Clustering with Knowledge Enrichment, “Transactions of the Association for Computational Linguistics”, 3(2015)15–28.
[8] Yong-Il Kim, Yoo-Kang Jiand Sun Park, Big Text Data Clustering using Class Labels and Semantic Feature Based on Hadoop of Cloud Computing, “International Journal of Software Engineering and Its Applications”, 8(2014),1-10.