Type 2 diabetes mellitus prediction model based on ensemble boosting method with Principal Component Analysis
M.Sornam 1 , M.Meharunnisa 2
Section:Research Paper, Product Type: Journal Paper
Volume-07 ,
Issue-05 , Page no. 124-130, Mar-2019
CrossRef-DOI: https://doi.org/10.26438/ijcse/v7si5.124130
Online published on Mar 10, 2019
Copyright © M.Sornam, M.Meharunnisa . This is an open access article distributed under the Creative Commons Attribution License, which permits unrestricted use, distribution, and reproduction in any medium, provided the original work is properly cited.
View this paper at Google Scholar | DPI Digital Library
How to Cite this Paper
- IEEE Citation
- MLA Citation
- APA Citation
- BibTex Citation
- RIS Citation
IEEE Style Citation: M.Sornam, M.Meharunnisa, “Type 2 diabetes mellitus prediction model based on ensemble boosting method with Principal Component Analysis,” International Journal of Computer Sciences and Engineering, Vol.07, Issue.05, pp.124-130, 2019.
MLA Style Citation: M.Sornam, M.Meharunnisa "Type 2 diabetes mellitus prediction model based on ensemble boosting method with Principal Component Analysis." International Journal of Computer Sciences and Engineering 07.05 (2019): 124-130.
APA Style Citation: M.Sornam, M.Meharunnisa, (2019). Type 2 diabetes mellitus prediction model based on ensemble boosting method with Principal Component Analysis. International Journal of Computer Sciences and Engineering, 07(05), 124-130.
BibTex Style Citation:
@article{_2019,
author = {M.Sornam, M.Meharunnisa},
title = {Type 2 diabetes mellitus prediction model based on ensemble boosting method with Principal Component Analysis},
journal = {International Journal of Computer Sciences and Engineering},
issue_date = {3 2019},
volume = {07},
Issue = {05},
month = {3},
year = {2019},
issn = {2347-2693},
pages = {124-130},
url = {https://www.ijcseonline.org/full_spl_paper_view.php?paper_id=818},
doi = {https://doi.org/10.26438/ijcse/v7i5.124130}
publisher = {IJCSE, Indore, INDIA},
}
RIS Style Citation:
TY - JOUR
DO = {https://doi.org/10.26438/ijcse/v7i5.124130}
UR - https://www.ijcseonline.org/full_spl_paper_view.php?paper_id=818
TI - Type 2 diabetes mellitus prediction model based on ensemble boosting method with Principal Component Analysis
T2 - International Journal of Computer Sciences and Engineering
AU - M.Sornam, M.Meharunnisa
PY - 2019
DA - 2019/03/10
PB - IJCSE, Indore, INDIA
SP - 124-130
IS - 05
VL - 07
SN - 2347-2693
ER -
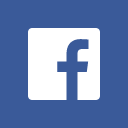
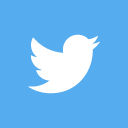
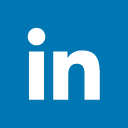
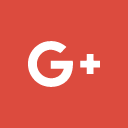
Abstract
In the recent years, data mining has been employed in the medical field for extracting and manipulating information, and aids within the higher process. There is a growing want for the medical establishments to be extra suggested and knowledgeable concerning the diseases and to understand the risk factors before diagnosis. Predicting the results of a process with a high level of accuracy is a difficult task. In this study we took the advantage of the data mining models to predict the Type – 2 Diabetes mellitus. The benchmark dataset, “Pima Indian Diabetes” dataset is used for this study. The main objective of this study is to propose the extensive data pre-processing such as imputation of missing values and a feature engineering technique namely ‘Principal Component Analysis’ are used to transform the dataset into a compressed form. Ensemble or classifier combination method called boosting method such as Gradient boosting machine and Random Forest are used. The most downside that’s attempting to be resolved isn’t solely to extend the accuracy however additionally to retain all the information in the data set while not removing the missing data. The missing data are imputed by a method called ‘predictive mean matching’. The results show that the ensemble learners, once used alongside PCA attained 100% accuracy of prediction. Moreover, it ensures that no missing information must be removed and might be imputed to confirm the data quality is enough. As a result, the model is shown to be helpful for the real time prediction of Diabetes Mellitus.
Key-Words / Index Term
Ensembles,Gradient boosting machine,Random Forest,Principal Component Analysis
References
[1] Ndisang, Joseph Fomusi, Alfredo Vannacci, and Sharad Rastogi. "Insulin Resistance, Type 1 and Type 2 Diabetes, and Related Complications 2017." Journal of diabetes research2017 ,2017
[2] Anjana, R.M., Deepa, M., Pradeepa, R., Mahanta, J., Narain, K., Das, H.K., Adhikari, P., Rao, P.V., Saboo, B., Kumar, A. and Bhansali, A., 2017. Prevalence of diabetes and prediabetes in 15 states of India: results from the ICMR–INDIAB population-based cross-sectional study. The Lancet Diabetes & Endocrinology,Vol. 5, Issue . 8, pp.585-596.
[3] Zimmet, P.Z., Magliano, D.J., Herman, W.H. and Shaw, J.E., 2014. Diabetes: a 21st century challenge. The lancet Diabetes & endocrinology,Vol. 2, Issue 1, pp.56-64.
[4] Ahmad, A., Mustapha, A., Zahadi, E.D., Masah, N. and Yahaya, N.Y., 2011. Comparison between Neural Networks against Decision Tree in Improving Prediction Accuracy for Diabetes Mellitus. In Digital Information Processing and Communications ,Springer, Berlin, Heidelberg. pp. 537-545
[5] Chen, W., Chen, S., Zhang, H. and Wu, T., November. A hybrid prediction model for type 2 diabetes using K-means and decision tree. 8th IEEE International Conference on Software Engineering and Service Science, (ICSESS). pp. 386-390,2017
[6] Wu, H., Yang, S., Huang, Z., He, J. and Wang, X., 2018. Type 2 diabetes mellitus prediction model based on data mining. Informatics in Medicine Unlocked,Vol. 10, pp.100-107.
[7] Kavakiotis, I., Tsave, O., Salifoglou, A., Maglaveras, N., Vlahavas, I. and Chouvarda, I., Machine learning and data mining methods in diabetes research. Computational and structural biotechnology journal,Vol. 15, pp.104-116,2017
[8] Choubey, D.K., Paul, S., Kumar, S. and Kumar, S., 2017, February. Classification of Pima indian diabetes dataset using naive bayes with genetic algorithm as an attribute selection. In Communication and Computing Systems: Proceedings of the International Conference on Communication and Computing System (ICCCS 2016) pp. 451-455, 2017
[9] kumar Dewangan, A. and Agrawal, P., Classification of diabetes mellitus using machine learning techniques. International Journal of Engineering Appled Science, Vol.2, Issue.5, pp.145-148, 2015.
[10] Amatul, Z., Asmawaty, T., Kadir, A. and MAM, A., A Comparative Study on the Pre-Processing and Mining of Pima Indian Diabetes Dataset, 2013.
[11] Vijayan, V.V. and Anjali, C.,Decision support systems for predicting diabetes mellitus—A Review. Global Conference in Communication Technologies, IEEE, pp. 98-103,April 2015 .
[12] Sowjanya, K., Singhal, A. and Choudhary, C., 2015, June. MobDBTest: A machine learning based system for predicting diabetes risk using mobile devices. IEEE International In Advance Computing Conference (IACC), pp. 397-402, 2015
[13] Gang, S., Shanshan, L. and Ding, Y., 2015, November. Design and Implementation of Diabetes Risk Assessment Model Based on Mobile Things. In,7th International Conference on Information Technology in Medicine and Education (ITME) pp.425-428, 2015.
[14] Choudhary, D., Suthar, O. P., Bhatia, P. K., & Biyani, G. “Zero” diastolic blood pressure. In The Indian Anaesthetists’ Forum . Medknow Publications and Media Pvt. Ltd, Vol. 17, No. 1, pp. 32-32, January 2016.
[15] Vitral, G. L. N., Aguiar, R. A. P. L., de Souza, I. M. F., Rego, M. A. S., Guimarães, R. N., & Reis, Z. S. N. (2018). Skin thickness as a potential marker of gestational age at birth despite different fetal growth profiles: A feasibility study. PloS one, Vol. 13, Issue 4, e0196542, 2018.
[16] Gisela Wilcox, Insulin and Insulin Resistance , Clinic Biochem Rev ,Vol 26, pp 19-39, 2005.
[17] Buuren, S. van, and Karin Groothuis-Oudshoorn. "mice: Multivariate imputation by chained equations in R." Journal of statistical software pp. 1-68, 2015.
[18] Breiman, Leo. "Bagging predictors." Machine learning 24.2 (1996): 123-140.
[19] Valentini, G. and Masulli, F.,. Ensembles of learning machines,In Italian Workshop on Neural Nets Springer, Berlin, Heidelberg, pp. 3-20, May 2002.
[20] Freund, Y., & Schapire, R. E. A decision-theoretic generalization of on-line learning and an application to boosting. Journal of computer and system sciences, Vol. 55,Issue 1,pp. 119-139,1997
[21] Breiman, L. Random forests. Machine learning, Vol.45,Issue 1, pp. 5-32,2001.
[22] Chaurasia, V., Pal, S., & Tiwari, B. B. (2018). Prediction of benign and malignant breast cancer using data mining techniques. Journal of Algorithms & Computational Technology, Vol.12, Issue 2, pp. 119-126.
[23] Singh, K., Lilhore, U. K., & Agrawal, N. An Efficient Supervised Learning Technique for Tumour Detection and Analysis from MR Image Data Set,2018
[24] Zhu, X. F., Zhu, B. S., Wu, F. M., & Hu, H. B. DNA methylation biomarkers for the occurrence of lung adenocarcinoma from TCGA data mining. Journal of cellular physiology, 2018.