Automatic Renal Defect Classification Using Inception
R. Vasanthselvakumar1 , M. Balasubramanian2 , S. Sathiya3
Section:Research Paper, Product Type: Journal Paper
Volume-07 ,
Issue-05 , Page no. 136-139, Mar-2019
CrossRef-DOI: https://doi.org/10.26438/ijcse/v7si5.136139
Online published on Mar 10, 2019
Copyright © R. Vasanthselvakumar, M. Balasubramanian, S. Sathiya . This is an open access article distributed under the Creative Commons Attribution License, which permits unrestricted use, distribution, and reproduction in any medium, provided the original work is properly cited.
View this paper at Google Scholar | DPI Digital Library
How to Cite this Paper
- IEEE Citation
- MLA Citation
- APA Citation
- BibTex Citation
- RIS Citation
IEEE Style Citation: R. Vasanthselvakumar, M. Balasubramanian, S. Sathiya, “Automatic Renal Defect Classification Using Inception,” International Journal of Computer Sciences and Engineering, Vol.07, Issue.05, pp.136-139, 2019.
MLA Style Citation: R. Vasanthselvakumar, M. Balasubramanian, S. Sathiya "Automatic Renal Defect Classification Using Inception." International Journal of Computer Sciences and Engineering 07.05 (2019): 136-139.
APA Style Citation: R. Vasanthselvakumar, M. Balasubramanian, S. Sathiya, (2019). Automatic Renal Defect Classification Using Inception. International Journal of Computer Sciences and Engineering, 07(05), 136-139.
BibTex Style Citation:
@article{Vasanthselvakumar_2019,
author = {R. Vasanthselvakumar, M. Balasubramanian, S. Sathiya},
title = {Automatic Renal Defect Classification Using Inception},
journal = {International Journal of Computer Sciences and Engineering},
issue_date = {3 2019},
volume = {07},
Issue = {05},
month = {3},
year = {2019},
issn = {2347-2693},
pages = {136-139},
url = {https://www.ijcseonline.org/full_spl_paper_view.php?paper_id=820},
doi = {https://doi.org/10.26438/ijcse/v7i5.136139}
publisher = {IJCSE, Indore, INDIA},
}
RIS Style Citation:
TY - JOUR
DO = {https://doi.org/10.26438/ijcse/v7i5.136139}
UR - https://www.ijcseonline.org/full_spl_paper_view.php?paper_id=820
TI - Automatic Renal Defect Classification Using Inception
T2 - International Journal of Computer Sciences and Engineering
AU - R. Vasanthselvakumar, M. Balasubramanian, S. Sathiya
PY - 2019
DA - 2019/03/10
PB - IJCSE, Indore, INDIA
SP - 136-139
IS - 05
VL - 07
SN - 2347-2693
ER -
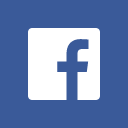
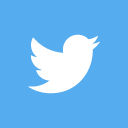
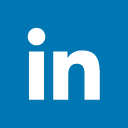
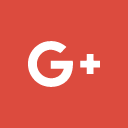
Abstract
Deep feature representation is more effective to perform classification of renal ultrasound images. Increases in distance of the features would suppresses the classification accuracy, conventional methods for categorization of renal diseases using medical ultrasound have the lack of accuracy due to restricted way of feature extraction. The main objective of this work is to classify the different renal diseases using ultrasound brightness mode images. Inception is derived with multiple convolutions and down sampling of input image elements in order to produce the deep features for classification. The projection of average pooling with convolution layer makes exacts reduction of unwanted invariants on the input image. The activation function rectified linear units are used for fast computation of the network architecture. The performance metrics for the classification of renal diseases have analyzed using confusion matrix. Inception produces better results than traditional convolution networks. The performance accuracy for the classification of renal diseases are given by 87.43%.
Key-Words / Index Term
Confusion matrix, Deep learning, Inception, Rectified linear units, Renal diseases, Ultrasound B-mode
References
[1] John D. Peipert, Peter M. Bentler, Kristi Klicko, and Ron D. Hays, “Psychometric Properties of the Kidney Disease Quality of Life 36-Item Short-Form Survey (KDQOL-36) in the United States”Elsevier transaction on American Journal of Kidney Diseases vol 71 issue 4 pp 1-9. April 2018.
[2] Tariq Shafi Eliseo Guallar, “Mapping Progress in Reducing Cardiovascular Risk with Kidney Disease”, American society of Nephrology vol 13. Issue 1. pp.1-3. jul, 2018.
[3] Rahul Roy, Susmitha gosh, Ashis gosh, “Speckle Denoising of Clinical Ultrasound Images Based on Fuzzy Spel Conformity in its Adjacency”|, Elsevier transaction on journal of applied soft computing, vol 73, pp. 394-417, dec 2018
[4] Prabha Sathees, Sujatha C. Manoharan “Proposal of index to estimate breast similarities in thermograms using fuzzy C means and anisotropic diffusion filter based fuzzy C means clustering”, Elsevier transaction on Infrared Physics & Technology vol 93. 316-325, Sep 2018.
[5] Morgan P. McBee, MD, Omer A. Awan, MD, MPH, CIIP, Andrew T. Colucci, MD, Comeron W. Ghobadi, MD, Nadja Kadom, MD, Akash P. Kansagr’, MD, MS, Srini Tridandapani, MD, PhD, MSEE, MSCR, MBA, William F. Auffermann, MD, PhD, “Deep Learning in Radiology” vol 25 issue 11 pp 12-14 Jan 2016.
[6] R. Vasanthselvakumar, M. Balasubramanian, S. Palanivel, “Pattern Analysis of Kidney Diseases for Detection and Classification Using Ultrasound B - Mode Images”, International Journal of Pure and Applied Mathematics, Volume 117 No. 15, pp. 635-653, 2017.
[7] Ladislav Rampasek1,2 and Anna Goldenberg, ” TensorFlow: Biology’s Gateway to Deep Learning?”, Elsevier transaction on cell system, vol 2 issue 1 pp. 12-14, Jan 2016.
[8] Christian Szegedy , Vincent Vanhoucke, Sergey Ioffe, Jon Shlens “Rethinking the Inception Architecture for Computer Vision”, In the proceedings IEEE Inetrnational Conference on Computer Vision and Foundation (ICVF 2016). pp. 2818-2826, 2016.
[9] Pietro Perona, Jitendra Malik, “Scale-Space and Edge Detection Using AnisotropicDiffusion”, IEEE Transactions on Pattern Analysis and Machine Intelligence, vol. 12. no. 7, pp 629-639, July 1990.
[10] Yin Zhu , Yuqiang Chen , Zhongqi Lu , Sinno Jialin Pan, Gui-Rong Xue, Yong Yu , and Qiang Yang, “Heterogeneous Transfer Learning for Image Classification, In the proceedings AAAI Conference on Artificial Intelligence (2011)”
[11] Xiaoling Xia, Cui Xu”inception-v3 for Flower Classifcation” In proc Image, Vision and Computing, pp 783-787. Jan 2017.