Classification of Network Traffic Based on Zero-Length Packets: A Review
S.Kalpana 1 , T. Raghu Trivedi2
Section:Review Paper, Product Type: Journal Paper
Volume-07 ,
Issue-06 , Page no. 103-105, Mar-2019
CrossRef-DOI: https://doi.org/10.26438/ijcse/v7si6.103105
Online published on Mar 20, 2019
Copyright © S.Kalpana, T. Raghu Trivedi . This is an open access article distributed under the Creative Commons Attribution License, which permits unrestricted use, distribution, and reproduction in any medium, provided the original work is properly cited.
View this paper at Google Scholar | DPI Digital Library
How to Cite this Paper
- IEEE Citation
- MLA Citation
- APA Citation
- BibTex Citation
- RIS Citation
IEEE Style Citation: S.Kalpana, T. Raghu Trivedi, “Classification of Network Traffic Based on Zero-Length Packets: A Review,” International Journal of Computer Sciences and Engineering, Vol.07, Issue.06, pp.103-105, 2019.
MLA Style Citation: S.Kalpana, T. Raghu Trivedi "Classification of Network Traffic Based on Zero-Length Packets: A Review." International Journal of Computer Sciences and Engineering 07.06 (2019): 103-105.
APA Style Citation: S.Kalpana, T. Raghu Trivedi, (2019). Classification of Network Traffic Based on Zero-Length Packets: A Review. International Journal of Computer Sciences and Engineering, 07(06), 103-105.
BibTex Style Citation:
@article{Trivedi_2019,
author = {S.Kalpana, T. Raghu Trivedi},
title = {Classification of Network Traffic Based on Zero-Length Packets: A Review},
journal = {International Journal of Computer Sciences and Engineering},
issue_date = {3 2019},
volume = {07},
Issue = {06},
month = {3},
year = {2019},
issn = {2347-2693},
pages = {103-105},
url = {https://www.ijcseonline.org/full_spl_paper_view.php?paper_id=877},
doi = {https://doi.org/10.26438/ijcse/v7i6.103105}
publisher = {IJCSE, Indore, INDIA},
}
RIS Style Citation:
TY - JOUR
DO = {https://doi.org/10.26438/ijcse/v7i6.103105}
UR - https://www.ijcseonline.org/full_spl_paper_view.php?paper_id=877
TI - Classification of Network Traffic Based on Zero-Length Packets: A Review
T2 - International Journal of Computer Sciences and Engineering
AU - S.Kalpana, T. Raghu Trivedi
PY - 2019
DA - 2019/03/20
PB - IJCSE, Indore, INDIA
SP - 103-105
IS - 06
VL - 07
SN - 2347-2693
ER -
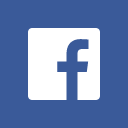
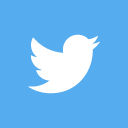
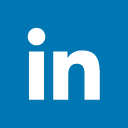
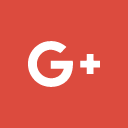
Abstract
Network traffic visitor’s classification is fundamental to network management and its performance. However, traditional traffic classifications, which were designed to work on a devoted hardware at very high line rates, may not feature well in digital software-primarily based surroundings. The advised fingerprinting scheme is strong to community conditions which include congestion, fragmentation, put off, retransmissions, duplications, and losses and to various processing abilities. Hence, its overall performance is largely independent of placement and migration problems, and consequently yields an appealing answer for virtualized software program-primarily based environments. We recommend an identical fingerprinting scheme for consumer datagram protocol traffic, which advantages from the equal blessings as the TCP one and attains very excessive accuracy as properly. Results show that our scheme effectively labeled about 97% of the flows on the dataset examined, even on encrypted facts.
Key-Words / Index Term
Network traffic classification, Network monitoring and measurements, Machine learning, Network function virtualization, Software-defined networking
References
[1] B. Pfaff, J. Pettit, T. Koponen, E. J. Jackson, A. Zhou, J. Rajahalme, J. Gross, A. Wang, J. Stringer, P. Shelar et al., “The design and implementation of open vswitch.” in NSDI, 2015, pp. 117–130.
[2] M. Rifai, N. Huin, C. Caillouet, F. Giroire, D. Lopez-Pacheco, J. Moulierac, and G. Urvoy-Keller, “Too many sdn rules? compress them with minnie,” in Global Communications Conference (GLOBECOM), 2015 IEEE. IEEE, 2015, pp. 1–7.
[3] C. Estan, K. Keys, D. Moore, and G. Varghese, “Building a better netflow,” in ACM SIGCOMM Computer Communication Review, vol. 34, no. 4. ACM, 2004, pp. 245–256.
[4] N. Hohn and D. Veitch, “Inverting sampled traffic,” in Proceedings of the 3rd ACM SIGCOMM conference on Internet measurement. ACM, 2003, pp. 222–233.
[5] N. Duffield, C. Lund, and M. Thorup, “Learn more, sample less: control of volume and variance in network measurement,” Information Theory, IEEE Transactions on, vol. 51, no. 5, pp. 1756–1775, 2005. [6] S. Fernandes, C. Kamienski, J. Kelner, D. Mariz, and D. Sadok, “A stratified traffic sampling methodology for seeing the big picture,” Computer Networks, vol. 52, no. 14, pp. 2677–2689, 2008.
[7] S. Zander, T. Nguyen, and G. Armitage, “Sub-flow packet sampling for scalable ml classification of interactive traffic,” in Local Computer Networks (LCN), 2012 IEEE 37th Conference on. IEEE, 2012.
[8] N. Katta, O. Alipourfard, J. Rexford, and D. Walker, “Rule-caching algorithms for software-defined networks,” Technical report, 2014.
[9] A. Vishnoi, R. Poddar, V. Mann, and S. Bhattacharya, “Effective switch memory management in openflow networks,” in Proceedings of the 8th ACM International Conference on Distributed Event-Based Systems. ACM, 2014, pp. 177–188.
[10] M. Kuzniar, P. Pere ´ sˇ´ıni, and D. Kostic, “What you need to know about ´ sdn flow tables,” in International Conference on Passive and Active Network Measurement. Springer, 2015, pp. 347–359. [11] Q. Maqbool, S. Ayub, J. Zulfiqar, and A. Shafi, “Virtual TCAM for data center switches,” in Network Function Virtualization and Software Defined Network (NFV-SDN), 2015 IEEE Conference on. IEEE, 2015, pp. 61–66.
[12] R. McGeer and P. Yalagandula, “Minimizing rulesets for TCAM implementation,” in IEEE INFOCOM, 2009, pp. 1314–1322.
[13] H. Zhu, S. Chen, L. Zhu, H. Li, and X. Chen, “Rangetree: A feature selection algorithm for c4. 5 decision tree,” in IEEE 5th International Conference on Intelligent Networking and Collaborative Systems (INCoS), 2013, pp. 17–22.
[14] T. Karagiannis, K. Papagiannaki, and M. Faloutsos, “Blinc: multilevel traffic classification in the dark,” in ACM SIGCOMM Computer Communication Review, vol. 35, no. 4. ACM, 2005, pp. 229–240.
[15] M. Tavallaee, W. Lu, and A. A. Ghorbani, “Online classification of network flows,” in Communication Networks and Services Research Conference, 2009. CNSR’09. Seventh Annual. IEEE, 2009.
[16] L. Grimaudo, M. Mellia, and E. Baralis, “Hierarchical learning for fine grained internet traffic classification,” in Wireless Communications and Mobile Computing Conference (IWCMC), 2012 8th International. IEEE, 2012, pp. 463–468.
[17] Y. Wang, Y. Xiang, and S. Yu, “Internet traffic classification using machine learning: A token-based approach,” in Computational Science and Engineering (CSE), 2011 IEEE 14th International Conference on. IEEE, 2011, pp. 285–289.
[18] S. Zander, T. Nguyen, and G. Armitage, “Automated traffic classification and application identification using machine learning,” in Local Computer Networks, 2005. 30th Anniversary. The IEEE Conference on. IEEE, 2005, pp. 250–257.
[19] A. Dainotti, W. De Donato, A. Pescape, and P. S. Rossi, “Classification of network traffic via packet-level hidden markov models,” in Global Telecommunications Conference, 2008. IEEE GLOBECOM 2008. IEEE. IEEE, 2008, pp. 1–5.
[20] Y.-D. Lin, C.-N. Lu, Y.-C. Lai, W.-H. Peng, and P.-C. Lin, “Application classification using packet size distribution and port association,” Journal of Network and Computer Applications, vol. 32, no. 5, pp. 1023–1030, 2009.