Prediction of Groundwater Level In District Level By Implementing Machine Learning And Advanced Softcomputing Techniques
Mooramreddy Sree Devi1 , Vempalli Rahamathulla2
Section:Survey Paper, Product Type: Journal Paper
Volume-07 ,
Issue-06 , Page no. 122-124, Mar-2019
CrossRef-DOI: https://doi.org/10.26438/ijcse/v7si6.122124
Online published on Mar 20, 2019
Copyright © Mooramreddy Sree Devi, Vempalli Rahamathulla . This is an open access article distributed under the Creative Commons Attribution License, which permits unrestricted use, distribution, and reproduction in any medium, provided the original work is properly cited.
View this paper at Google Scholar | DPI Digital Library
How to Cite this Paper
- IEEE Citation
- MLA Citation
- APA Citation
- BibTex Citation
- RIS Citation
IEEE Style Citation: Mooramreddy Sree Devi, Vempalli Rahamathulla, “Prediction of Groundwater Level In District Level By Implementing Machine Learning And Advanced Softcomputing Techniques,” International Journal of Computer Sciences and Engineering, Vol.07, Issue.06, pp.122-124, 2019.
MLA Style Citation: Mooramreddy Sree Devi, Vempalli Rahamathulla "Prediction of Groundwater Level In District Level By Implementing Machine Learning And Advanced Softcomputing Techniques." International Journal of Computer Sciences and Engineering 07.06 (2019): 122-124.
APA Style Citation: Mooramreddy Sree Devi, Vempalli Rahamathulla, (2019). Prediction of Groundwater Level In District Level By Implementing Machine Learning And Advanced Softcomputing Techniques. International Journal of Computer Sciences and Engineering, 07(06), 122-124.
BibTex Style Citation:
@article{Devi_2019,
author = {Mooramreddy Sree Devi, Vempalli Rahamathulla},
title = {Prediction of Groundwater Level In District Level By Implementing Machine Learning And Advanced Softcomputing Techniques},
journal = {International Journal of Computer Sciences and Engineering},
issue_date = {3 2019},
volume = {07},
Issue = {06},
month = {3},
year = {2019},
issn = {2347-2693},
pages = {122-124},
url = {https://www.ijcseonline.org/full_spl_paper_view.php?paper_id=881},
doi = {https://doi.org/10.26438/ijcse/v7i6.122124}
publisher = {IJCSE, Indore, INDIA},
}
RIS Style Citation:
TY - JOUR
DO = {https://doi.org/10.26438/ijcse/v7i6.122124}
UR - https://www.ijcseonline.org/full_spl_paper_view.php?paper_id=881
TI - Prediction of Groundwater Level In District Level By Implementing Machine Learning And Advanced Softcomputing Techniques
T2 - International Journal of Computer Sciences and Engineering
AU - Mooramreddy Sree Devi, Vempalli Rahamathulla
PY - 2019
DA - 2019/03/20
PB - IJCSE, Indore, INDIA
SP - 122-124
IS - 06
VL - 07
SN - 2347-2693
ER -
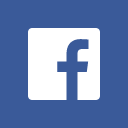
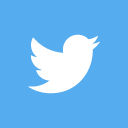
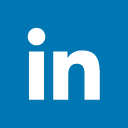
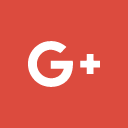
Abstract
Groundwater plays a major role in human life. Now-a-days, the Groundwater levels are gradually decreasing due to pollution and over usage of water and lack of rains. The air pollution caused by industries and human wastage reducess the Groundwater. The increased Groundwater threat is a threat to human life. There is no proper planning and infrastructure to preserve the Groundwater. The bore wells and tube wells pump the Groundwater from a very deep source. Over usage of sand also causes the decrement of Groundwater level. Now-a-days, due to the the scarcity of Groundwater, the farmer is unable to decide the kind crop to be grown in his/her land. This is a complex task. The food bowl of India is day by day becoming weak due to a the scarcity of Groundwater. “Atmospherical science is the best source of study for analyzing and predicting the weather phenomenon and suggests ways and means overcome the problem”[1].
Key-Words / Index Term
Rainfall, Geographical Parameters,an aquifer
References
[1]. Aziz,A.R.A. and Wong, K.F.V. (1992) Neural network approach the determination of aquifer parameter. Groundwater, v.30(2), pp.164-166.
[2]. ESMAILI VARAKI, M. (2003) Presenting an intelligent model for estimating the fluctuation of alluvial aquifer groundwater level by using artificial neural network. the collection of first two yearly conference of managing water resources of Iran.
[3]. GIDSON, S (2009). Application of Artificial Neural Networks in the Field of Geohydrology. Thesis for Msc of University of the Free State.
[4]. KAASTRA, I. and BOYD, M. (1996). Designing a neural network for forecasting financial and economic time series. Neurocomputing, v.10(3), pp.215-236.
[5]. MAIER, H.R. and DANDY, G.C. (2000) Neural networks for the prediction and forecasting of water esources variables: a review of modeling issues and applications. Environ. Modell. Software, v.15, pp.101–124.
[6]. TASLLOTI, B. (2004) Estimating groundwater level by using MODFLOW and artificial neural network, Msc thesis of irrigation and drainage engineering, Tarbiat Modares University of Tehran, Iran.
[7]. VEMURI, V.R. (1992) Artificial Neural Networks, Concepts and ControlApplications, IEEE Computer Soc. Pr., Los Alamos, California.
[8]. V.Rahamathulla, S.Ramakrishna.(2017) Prediction of rainfall through Data Science using Time Series analysis and Prediction Analysit, IJETTCS, Volume 7, Issue 1, January – February 2018.
[9]. LALLAHEM, S., MANINA, J., HANI,A. and NAJJAR,Y. (2005)on the use of neural networks to evaluate groundwater levels in fractured porous media. Jour. Math. Computer Modell., v.37, pp.1047-1061.
[10]. NAZARI, J. and ERSOY, O. (1992). Implementation of Back Propagation Neural Networks with Matlab, School of Electrical Engineering – Purdue University, West Lafayette, Indiana,