A Review on Traffic classification Based on Zero-Length Packets
M.G. Divya1
Section:Review Paper, Product Type: Journal Paper
Volume-07 ,
Issue-06 , Page no. 132-134, Mar-2019
CrossRef-DOI: https://doi.org/10.26438/ijcse/v7si6.132134
Online published on Mar 20, 2019
Copyright © M.G. Divya . This is an open access article distributed under the Creative Commons Attribution License, which permits unrestricted use, distribution, and reproduction in any medium, provided the original work is properly cited.
View this paper at Google Scholar | DPI Digital Library
How to Cite this Paper
- IEEE Citation
- MLA Citation
- APA Citation
- BibTex Citation
- RIS Citation
IEEE Style Citation: M.G. Divya, “A Review on Traffic classification Based on Zero-Length Packets,” International Journal of Computer Sciences and Engineering, Vol.07, Issue.06, pp.132-134, 2019.
MLA Style Citation: M.G. Divya "A Review on Traffic classification Based on Zero-Length Packets." International Journal of Computer Sciences and Engineering 07.06 (2019): 132-134.
APA Style Citation: M.G. Divya, (2019). A Review on Traffic classification Based on Zero-Length Packets. International Journal of Computer Sciences and Engineering, 07(06), 132-134.
BibTex Style Citation:
@article{Divya_2019,
author = {M.G. Divya},
title = {A Review on Traffic classification Based on Zero-Length Packets},
journal = {International Journal of Computer Sciences and Engineering},
issue_date = {3 2019},
volume = {07},
Issue = {06},
month = {3},
year = {2019},
issn = {2347-2693},
pages = {132-134},
url = {https://www.ijcseonline.org/full_spl_paper_view.php?paper_id=884},
doi = {https://doi.org/10.26438/ijcse/v7i6.132134}
publisher = {IJCSE, Indore, INDIA},
}
RIS Style Citation:
TY - JOUR
DO = {https://doi.org/10.26438/ijcse/v7i6.132134}
UR - https://www.ijcseonline.org/full_spl_paper_view.php?paper_id=884
TI - A Review on Traffic classification Based on Zero-Length Packets
T2 - International Journal of Computer Sciences and Engineering
AU - M.G. Divya
PY - 2019
DA - 2019/03/20
PB - IJCSE, Indore, INDIA
SP - 132-134
IS - 06
VL - 07
SN - 2347-2693
ER -
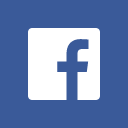
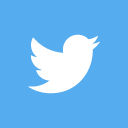
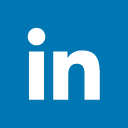
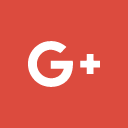
Abstract
A system, or information arrange, is a computerized media communications organize which enables hubs to share assets. In PC systems, registering gadgets trade information with one another utilizing associations between hubs. In this paper, we devise a novel fingerprinting method that can be used as a product based arrangement which empowers machine-learning based characterization of progressing streams. The proposed plan is extremely easy to actualize and requires negligible assets, yet accomplishes high exactness. In particular, for TCP streams, we propose a unique finger impression that depends on zero-length parcels, subsequently empowers an exceedingly proficient inspecting technique which can be embraced with a solitary CAM rule. The proposed fingerprinting plan is vigorous to organize conditions, for example, clog, fracture, delay, retransmissions, duplications and misfortunes and to changing preparing abilities. Consequently, its execution is basically free of position and relocation issues, and in this way yields an appealing answer for virtualized programming based conditions. We recommend a practically equivalent to fingerprinting plan for UDP traffic, which profits by indistinguishable favorable circumstances from the TCP one and achieves high precision also. Results demonstrate that our plan effectively ordered about 97% of the streams on the dataset tried, even on scrambled information.
Key-Words / Index Term
Machine Learning, Software-defined networking, Network traffic classification
References
[1] Cao, J., Fang, Z., Qu, G., Sun, H., Zhang, D.: An accurate traffic classification model based on support vector machines. Int. J. Netw. Manag. 27, e1962 (2017)
[2] Ertam, F., Avc, E.: A new approach for internet traffic classification: GA-WK-ELM. Measurement 95, 135–142 (2017)
[3] Munther, A., Othman, R.R., Alsaadi, A.S., Anbar, M.: A performance study of hidden Markov model and random forest in internet traffic classification. In: Kim, K., Joukov, N. (eds.) Information Science and Applications (ICISA) 2016. LNEE, vol. 376, pp. 319–329. Springer, Singapore (2016).
[4] Rizzi, A., Iacovazzi, A., Baiocchi, A., Colabrese, S.: A low complexity real-time internet traffic flows neuro-fuzzy classifier. Comput. Netw. 91, 752–771 (2015)
[5] Velan, P., Čermák, M., Čeleda, P., Drašar, M.: A survey of methods for encrypted traffic classification and analysis. Int. J. Netw. Manag. 25(5), 355–374 (2015)
[6] Peng, L., Yang, B., Chen, Y.: Hierarchical RBF neural network using for early stage internet traffic identification. In: 2014 IEEE 17th International Conference on Computational Science and Engineering. Institute of Electrical and Electronics Engineers (IEEE) (2014)
[7] P. Mehta and R. Shah, “A survey of network based traffic classification methods,” Database Systems Journal, vol. 7, no. 4, pp. 24–31, 2017.
[8] De Donato, Walter, Antonio Pescapé, and Alberto Dainotti. "Traffic identification engine: an open platform for traffic classification ." IEEE Network 28.2. 2014. 56-64.
[9] Finsterbusch, Michael. "A survey of payload-based traffic classification approaches." IEEE Communications Surveys & Tutorials 16.2. 2014. 1135- 1156.
[10] Xue, Yibo, Dawei Wang, and Luoshi Zhang. "Traffic classification: Issues and challenges." Computing, Networking and Communications (ICNC) International Conference on IEEE. 2013.
[11] Valenti, Silvio. "Reviewing traffic classification - Data Traffic Monitoring and Analysis." Springer Berlin Heidelberg, 2013. 123-147.
[12] Zhang, Jun. "An effective network traffic classification method with unknown flow detection." IEEE Transactions on Network and Service Management 10.2. 2013. 133-147.
[13] Abijith Sankar, P. Divya Bharathi, M. Midhun, K. Vijay, T. Senthil Kumar, "A Coniectural study on Machine Learning Algorithms", Advances in Intelligent Systems and Computing, pp. 105-116, Dec 2015.
[14] Yu Wang, Yang Xiang, Jun Zhang, Wanlei Zhou, Guiyi Wei, Laurence T. Yang, "Internet Traffic Classification Using Constrained Clustering", IEEE Transactions On Parallel And Distributed Systems, vol. 25, no. 11, November 2014.
[15] M. Shafiq, X. Yu, A. A. Laghari, L. Yao, N. K. Karn, and F. Abdessamia, “Network traffic classification techniques and comparative analysis using machine learning algorithms,” in Computer and Communications (ICCC), 2016 2nd IEEE International Conference on. IEEE, 2016, pp. 2451–2455.
[16] Jie Cao et al., "Network Traffic Classification Using Feature Selection and Parameter Optimization", Journal of Communications, vol. 10.10, 2015.
[17] Pawel Foremski, On different ways to classify Internet traffic: a short review of selected publications Theoretical and Applied Informatics, 2013.
[18] B. Pfaff, J. Pettit, T. Koponen, E. J. Jackson, A. Zhou, J. Rajahalme, J. Gross, A. Wang, J. Stringer, P. Shelar et al., “The design and implementation of open vswitch.” in NSDI, 2015, pp. 117–130.
[19] J. Hwang, K. K. Ramakrishnan, and T. Wood. NetVM: High Performance and Flexible Networking Using Virtualization on Commodity Platforms. In Proc. of NSDI, Apr. 2014.
[20] ] N. Katta, O. Alipourfard, J. Rexford, and D. Walker. Infinite CacheFlow in Software-Defined Networks. In Proc. of HotSDN, 2014.
[21] K. Kogan, S. Nikolenko, O. Rottenstreich, W. Culhane, and P. Eugster. SAX-PAC (Scalable And eXpressive PAcket Classification). In Proc. of SIGCOMM, 2014.