Big Healthcare Data Privacy Preservation –A Technological Perspective
Suneetha V1 , Srivatsala V2 , Kumara wamy Y S3
Section:Research Paper, Product Type: Journal Paper
Volume-07 ,
Issue-09 , Page no. 69-75, Apr-2019
CrossRef-DOI: https://doi.org/10.26438/ijcse/v7si9.6975
Online published on Apr 30, 2019
Copyright © Suneetha V, Srivatsala V, Kumara Swamy Y S . This is an open access article distributed under the Creative Commons Attribution License, which permits unrestricted use, distribution, and reproduction in any medium, provided the original work is properly cited.
View this paper at Google Scholar | DPI Digital Library
How to Cite this Paper
- IEEE Citation
- MLA Citation
- APA Citation
- BibTex Citation
- RIS Citation
IEEE Citation
IEEE Style Citation: Suneetha V, Srivatsala V, Kumara Swamy Y S, “Big Healthcare Data Privacy Preservation –A Technological Perspective,” International Journal of Computer Sciences and Engineering, Vol.07, Issue.09, pp.69-75, 2019.
MLA Citation
MLA Style Citation: Suneetha V, Srivatsala V, Kumara Swamy Y S "Big Healthcare Data Privacy Preservation –A Technological Perspective." International Journal of Computer Sciences and Engineering 07.09 (2019): 69-75.
APA Citation
APA Style Citation: Suneetha V, Srivatsala V, Kumara Swamy Y S, (2019). Big Healthcare Data Privacy Preservation –A Technological Perspective. International Journal of Computer Sciences and Engineering, 07(09), 69-75.
BibTex Citation
BibTex Style Citation:
@article{V_2019,
author = {Suneetha V, Srivatsala V, Kumara Swamy Y S},
title = {Big Healthcare Data Privacy Preservation –A Technological Perspective},
journal = {International Journal of Computer Sciences and Engineering},
issue_date = {4 2019},
volume = {07},
Issue = {09},
month = {4},
year = {2019},
issn = {2347-2693},
pages = {69-75},
url = {https://www.ijcseonline.org/full_spl_paper_view.php?paper_id=957},
doi = {https://doi.org/10.26438/ijcse/v7i9.6975}
publisher = {IJCSE, Indore, INDIA},
}
RIS Citation
RIS Style Citation:
TY - JOUR
DO = {https://doi.org/10.26438/ijcse/v7i9.6975}
UR - https://www.ijcseonline.org/full_spl_paper_view.php?paper_id=957
TI - Big Healthcare Data Privacy Preservation –A Technological Perspective
T2 - International Journal of Computer Sciences and Engineering
AU - Suneetha V, Srivatsala V, Kumara Swamy Y S
PY - 2019
DA - 2019/04/30
PB - IJCSE, Indore, INDIA
SP - 69-75
IS - 09
VL - 07
SN - 2347-2693
ER -
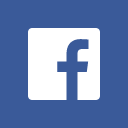
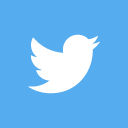
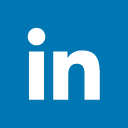
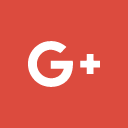
Abstract
In this digital world, by virtue of highly diversified data generating technologies – huge amount of data is being churned out by organizations like hospices, banks, e-commerce, retail and supply chain, etc.,. Heaps and loads of big data is being generated every minute, by humans and machines. Because of onset of big data the industries have fundamentally changes their way of handling data. The volume and velocity big data generated from the various sources can be managed and analyzed to take appropriate decisions to benefit the organization. One of the most promising fields where big data analytics can be applied is healthcare. Big healthcare data and its analytics has considerable potential to improve quality of patients’ life, gain valuable insights, prevent diseases, make healthcare more affordable. Securing data of patients and ensuring its security is major concern of data analytics. Unless the privacy and security issues of Big Data are addressed in healthcare industry it cannot be too useful. Invasion of patient privacy is a growing concern in big data analytics as emerging threats and vulnerabilities continue to grow. It is necessary to ensure a secure and sound environment for big data for better future in research by repairing the available solutions. In this paper, we present the security and privacy issues in big data applicable to healthcare industry. Also, we discuss the various Anonymization and Encryption techniques to preserve the privacy of the data, comparing their strengths and limitations.
Key-Words / Index Term
Healthcare; Healthcare privacy; Big data security; Big data Privacy; Data Anonymization, K-anonymity, T-closeness, L-diversity
References
[1]. Karim Abouelmehdi, Abderrahim Beni-Hssane, Hayat Khaloufi, Mostafa Saadi. "Big data security and privacy in healthcare: A Review", Procedia Computer Science, 2017
[2]. Aggarwal CC, Philip SY. A general survey of privacy-preserving data mining models and algorithms. Privacy-preserving data mining. Springer: US; 2008. p. 11–52.
[3]. Jiang R, Lu R, Choo KK. Achieving high performance and privacy-preserving query over encrypted multidimensional big metering data. Future Gen Comput Syst. 2018;78:392–401.
[4]. Hettig M, Kiss E, Kassel J-F, Weber S, Harbach M. Visualizing risk by example: demonstrating threats arising from android apps. In: Smith M, editor. Symposium on usable privacy and security (SOUPS), Newcastle, UK, July 24–26, 2013.
[5]. Bayardo RJ, Agrawal A. Data privacy through optimal k-anonymization. In: Proceedings 21st international conference on data engineering, 2005 (ICDE 2005). Piscataway: IEEE; 2005.
[6]. Iyengar S. Transforming data to satisfy privacy constraints. In: Proceedings of the eighth ACM SIGKDD international conference on knowledge discovery and data mining. New York: ACM; 2002.
[7]. LeFevre K, DeWitt DJ, Ramakrishnan R. Incognito: efficient full-domain k-anonymity. In: Proceedings of the 2005 ACM SIGMOD international conference on management of data. New York: ACM; 2005.
[8]. LeFevre K, DeWitt DJ, Ramakrishnan R. Mondrian multidimensional k-anonymity. In: Proceedings of the 22nd international conference (ICDE’06) on data engineering, 2006. New York: ACM; 2006.
[9]. Samarati, Pierangela, and Latanya Sweeney. In: Protecting privacy when disclosing information: k-anonymity and its enforcement through generalization and suppression. Technical report, SRI International, 1998.
[10]. Sweeney Latanya. Achieving k-anonymity privacy protection using generalization and suppression. In J Uncertain Fuzziness Knowl Based Syst. 2002;10(05):571–88.
[11]. Sweeney Latanya. k-Anonymity: a model for protecting privacy. Int J Uncertain, Fuzziness Knowl Based Syst. 2002;10(05):557–70.
[12]. Williams R. On the complexity of optimal k-anonymity. In: Proc. 23rd ACM SIGMOD-SIGACT-SIGART symp. principles of database systems (PODS). New York: ACM; 2004.
[13]. Machanavajjhala A et al. L-diversity: privacy beyond k-anonymity. In: Proceedings of the 22nd international conference on data engineering (ICDE’06), 2006. Piscataway: IEEE; 2006.
[14]. Xiao X, Yufei T. Personalized privacy preservation. In: Proceedings of the 2006 ACM SIGMOD international conference on Management of data. New York: ACM; 2006.
[15]. Rubner Y, Tomasi T, Guibas LJ. The earth mover’s distance as a metric for image retrieval. Int J Comput Vision. 2000;40(2):99–121.
[16]. Priyank Jain* , Manasi Gyanchandani and Nilay Khare; Big data privacy: a technological perspective and review; Journal of Big data.
[17]. Karim Abouelmehdi*, Abderrahim Beni‑Hessane and Hayat Khaloufi; Big healthcare data: preserving security and privacy; Journal of Big data.
[18]. P. Ram Mohan Rao, S. Murali Krishna, A. P.Siva Kumar. "Privacy preservation techniques in big data analytics: a survey", Journal of Big Data, 2018 journalofbigdata.springeropen.com
[19]. Kajol Patel, G. B. Jethava. "Privacy Preserving Techniques for Big Data: A Survey", 2018 Second International Conference on Inventive Communication and Computational Technologies (ICICCT), 2018.
[20]. OECD Health Care Quality Framework (OECD Health Working Paper No. 23, March 2006). https://eugdprcompliant.com/