Data Mining Techniques for Customer Relationship Management
AR. PonPeriasamy1 , G. Vijayasree2
Section:Review Paper, Product Type: Journal Paper
Volume-5 ,
Issue-4 , Page no. 120-126, Apr-2017
Online published on Apr 30, 2017
Copyright © AR. PonPeriasamy, G. Vijayasree . This is an open access article distributed under the Creative Commons Attribution License, which permits unrestricted use, distribution, and reproduction in any medium, provided the original work is properly cited.
View this paper at Google Scholar | DPI Digital Library
How to Cite this Paper
- IEEE Citation
- MLA Citation
- APA Citation
- BibTex Citation
- RIS Citation
IEEE Style Citation: AR. PonPeriasamy, G. Vijayasree, “Data Mining Techniques for Customer Relationship Management,” International Journal of Computer Sciences and Engineering, Vol.5, Issue.4, pp.120-126, 2017.
MLA Style Citation: AR. PonPeriasamy, G. Vijayasree "Data Mining Techniques for Customer Relationship Management." International Journal of Computer Sciences and Engineering 5.4 (2017): 120-126.
APA Style Citation: AR. PonPeriasamy, G. Vijayasree, (2017). Data Mining Techniques for Customer Relationship Management. International Journal of Computer Sciences and Engineering, 5(4), 120-126.
BibTex Style Citation:
@article{PonPeriasamy_2017,
author = { AR. PonPeriasamy, G. Vijayasree},
title = {Data Mining Techniques for Customer Relationship Management},
journal = {International Journal of Computer Sciences and Engineering},
issue_date = {4 2017},
volume = {5},
Issue = {4},
month = {4},
year = {2017},
issn = {2347-2693},
pages = {120-126},
url = {https://www.ijcseonline.org/full_paper_view.php?paper_id=1254},
publisher = {IJCSE, Indore, INDIA},
}
RIS Style Citation:
TY - JOUR
UR - https://www.ijcseonline.org/full_paper_view.php?paper_id=1254
TI - Data Mining Techniques for Customer Relationship Management
T2 - International Journal of Computer Sciences and Engineering
AU - AR. PonPeriasamy, G. Vijayasree
PY - 2017
DA - 2017/04/30
PB - IJCSE, Indore, INDIA
SP - 120-126
IS - 4
VL - 5
SN - 2347-2693
ER -
![]() |
![]() |
![]() |
545 | 406 downloads | 468 downloads |
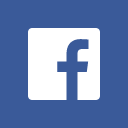
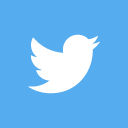
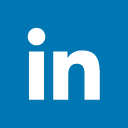
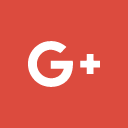
Abstract
Advancements in technology have made relationship marketing a reality in recent years. Technologies such as data warehousing, data mining, and campaign management software have made customer relationship management a new area where firms can gain a competitive advantage. Particularly through data mining—the extraction of hidden predictive information from large databases—organizations can identify valuable customers, predict future behaviors, and enable firms to make proactive, knowledge-driven decisions. The automated, future-oriented analyses made possible by data mining move beyond the analyses of past events typically provided by history-oriented tools such as decision support systems. Data mining tools answer business questions that in the past were too time-consuming to pursue. Yet, it is the answers to these questions make customer relationship management possible. Various techniques exit among data mining software, each with their own advantages and challenges for different types of applications. A particular dichotomy exists between neural networks and chi-square automated interaction detection (CHAID). While differing approaches abound in the realm of data mining, the use of some type of data mining is necessary to accomplish the goals of today’s customer relationship management philosophy.
Key-Words / Index Term
Customer relationship management (CRM),Relationship, CHAID, Decision support system and Datamining
References
[1] EK Clemons, WC. Row, “Information technology and industrial cooperation: the changing economics of coordination and ownership”, Journal of Management Information Systems, Vol.9, Issue.2, pp.9-28, 1992.
[2] GH. Nguyen, A. Bouzerdoum, S.L. Phung, “A supervised learning approach for imbalanced data sets”, 2008 19th International Conference on Pattern Recognition, Tampa, pp.1-4, 2008.
[3] H. R. Qodmanan, “Multi objective association rule mining with genetic algorithm without specifying minimum support and minimum confidence”, Expert Systems with Applications, vol. 38, Issue.1, pp. 288-298, 2011.
[4] Dun Bradstreet, “Data Intelligence Group Pilot Software. An overview of data mining”, DIG White Paper, USA, pp.1-13, 2011
[5] Gang Wang, Jian Ma, “A hybrid ensemble approach for enterprise credit risk assessment based on Support Vector Machine”, Expert System with Applications, Vol.39, Issue.5, pp.5325-5331, 2012.
[7] H. Jin, “Personality mining method in web based education system using data mining”, IEEE International Conference on Grey Systems and Intelligent Services, Chin, pp.155-158, 2007.
[8]H. R. Qodmanan, “Multi objective association rule mining with genetic algorithm without specifying minimum support and minimum confidence”, Expert Systems with Applications, vol. 38, Issue.1, pp. 288-298, 2011.
[9] J. Gao, B. Ding, F. Wei, H. Jiawei, PS. Yu, “Classifying data streams with skewed class distributions and concept drifts”, IEEE Internet Computer, vol. 12, no. 6, pp. 37-49, 2008.
[10]KS. Priya, AV. Senthil Kumar, “Improving the Students Performance Using Educational Data Mining”, Int. J. Advanced Networking and Applications, Vol.4 Issue.4, pp.1680-1685, 2013.
[11] Jayshree Jha, Leena Ragha , “Educational Data Mining using Improved Apriori Algorithm”, International Journal of Information and Computation Technology, Vol.3, Issue.5, pp.411-418, 2013.
[12] K Shymala. and S.P Rajgopalan., “Data Mining Model for a Better Higher Educational System”, Information Technology Journal, Vol.5, Issue.3, pp.560-564, 2006.
[13] B. Bakariya, G.S. Thakur, “Effectuation of Web Log Preprocessing and Page Access Frequency using Web Usage Mining”, International Journal of Computer Sciences and Engineering, Vol.1, Issue.1, pp.1-5, 2013.
[14] C. Rygielski, JC. Wang, DC. Yen, “Data mining techniques for customer relationship management. Technology in society”, Vol.24, Issue.4, pp.483-502, 2002.
[15] L. I. Kuncheva, “Classifier ensembles for detecting concept change in streaming data: Overview and perspectives”, in Proc. Eur. Conf. Artificial Intelligence, USA, pp.5-10, 2008.
[16] Nidhi Sethi and Pradeep Sharma, “Mining Frequent Pattern from Large Dynamic Database Using Compacting Data Sets”, International Journal of Scientific Research in Computer Science and Engineering, Vol.1, Issue.3, pp.31-34, 2013.
[17] S.Praveen Kumar and R.V.Krishnaiah, “Efficient Computation of Range Aggregates Against Uncertain Location Based Queries using Filtering-and-Verification Algorithm”, International Journal of Scientific Research in Computer Science and Engineering, Vol.1, Issue.3, pp.56-61, 2013.
[18]A. M. Rajee and F. Sagayaraj Francis, “A Probabilistic Estimation of Cluster Region Prone to Inter Cluster Data Movement”, International Journal of Computer Sciences and Engineering, Vol.2, Issue.11, pp.138-141, 2014.