Mining Based Design and Analysis of Social Spam Detection in Micro-blogging
R. Chugga1 , P. Dashore2
- Dept. of CSE, Sanghvi Innovative Academy, Indore, India.
- Dept. of CSE, Sanghvi Innovative Academy, Indore, India.
Correspondence should be addressed to: rimpalchugga@gmail.com.
Section:Research Paper, Product Type: Journal Paper
Volume-5 ,
Issue-7 , Page no. 101-109, Jul-2017
CrossRef-DOI: https://doi.org/10.26438/ijcse/v5i7.101109
Online published on Jul 30, 2017
Copyright © R. Chugga, P. Dashore . This is an open access article distributed under the Creative Commons Attribution License, which permits unrestricted use, distribution, and reproduction in any medium, provided the original work is properly cited.
View this paper at Google Scholar | DPI Digital Library
How to Cite this Paper
- IEEE Citation
- MLA Citation
- APA Citation
- BibTex Citation
- RIS Citation
IEEE Style Citation: R. Chugga, P. Dashore , “Mining Based Design and Analysis of Social Spam Detection in Micro-blogging,” International Journal of Computer Sciences and Engineering, Vol.5, Issue.7, pp.101-109, 2017.
MLA Style Citation: R. Chugga, P. Dashore "Mining Based Design and Analysis of Social Spam Detection in Micro-blogging." International Journal of Computer Sciences and Engineering 5.7 (2017): 101-109.
APA Style Citation: R. Chugga, P. Dashore , (2017). Mining Based Design and Analysis of Social Spam Detection in Micro-blogging. International Journal of Computer Sciences and Engineering, 5(7), 101-109.
BibTex Style Citation:
@article{Chugga_2017,
author = {R. Chugga, P. Dashore },
title = {Mining Based Design and Analysis of Social Spam Detection in Micro-blogging},
journal = {International Journal of Computer Sciences and Engineering},
issue_date = {7 2017},
volume = {5},
Issue = {7},
month = {7},
year = {2017},
issn = {2347-2693},
pages = {101-109},
url = {https://www.ijcseonline.org/full_paper_view.php?paper_id=1373},
doi = {https://doi.org/10.26438/ijcse/v5i7.101109}
publisher = {IJCSE, Indore, INDIA},
}
RIS Style Citation:
TY - JOUR
DO = {https://doi.org/10.26438/ijcse/v5i7.101109}
UR - https://www.ijcseonline.org/full_paper_view.php?paper_id=1373
TI - Mining Based Design and Analysis of Social Spam Detection in Micro-blogging
T2 - International Journal of Computer Sciences and Engineering
AU - R. Chugga, P. Dashore
PY - 2017
DA - 2017/07/30
PB - IJCSE, Indore, INDIA
SP - 101-109
IS - 7
VL - 5
SN - 2347-2693
ER -
![]() |
![]() |
![]() |
669 | 391 downloads | 450 downloads |
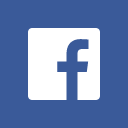
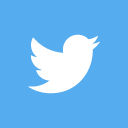
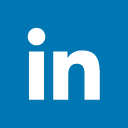
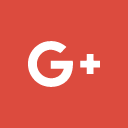
Abstract
The web-based social networking becomes a valuable part of over life. Young clients can pay a significant amount of time on this social platform. The primary reason behind the time expense on the social media is to check the updates on the different area of interest i.e. politics, movies, and others. The updates on these domains are obtained on the basis of the trending topics. But sometimes the similar or duplicate topics are flooded on social media due to this un-necessary traffic, redundancy, and storage overheads increases. Keeping in mind the end goal need to identify the duplicate post on the social network applications and remove them is a better solution. By this inspiration a new data model using the big data mining is introduced in this work. The proposed data model contributes by accepting the online and offline data both. After that the three phase of pre-processing is performed on the data first the removal of stop words, removal of punctuations, and completion of abbreviations. The pre-processed data is further ranked on the basis of Jaccard similarity index. This ranked data is further used with the fuzzy c-means algorithm. The fuzzy c-means algorithm computes the different groups of the similar tweets. Thus in further for finding the similar tweets the synonyms based re-tweets are generated with the mutation methodology. Finally the hashes of all the data are computed and the similar hash value based tweets are removed. The implementation of the proposed method is finished on the idea of JAVA era and hadoop storage. Additionally after implementation of the proposed technique, the technique is compared with the similar technique on the basis of their precision and recall values. The computed results demonstrate the high degree of accurate duplicate data identification and their removal for the micro-blog data analysis.
Key-Words / Index Term
Big Data, Hadoop, FCM(fuzzy c-means), Social Spam, Clustering, Twitter
References
[1] Jiang, Meng, P. Cui, and C. Faloutsos, "Suspicious behavior detection: Current trends and future directions," IEEE Intelligent Systems, Vol.31,issue.1, pp. 31-39, 2016
[2] J.S. Rohankar, “A Study on Advanced Security Techniques to Provide Security for Social Networking as Data Mining”, International Journal of Advance Foundation and Research in Computer (IJAFRC) Vol.2, Special Issue (NCRTIT 2015), January 2015.
[3] L. Cipriani, “Goal! Detecting the most important World Cup moments”, Technical report, Twitter, 2014.
[4] Chu, Zi, I. Widjaja, and H. Wang, "Detecting social spam campaigns on twitter", International Conference on Applied Cryptography and Network Security, Springer Berlin Heidelberg, 2012.
[5] Ghosh, Saptarshi, "Understanding and combating link farming in the twitter social network", ACM, Proceedings of the 21st international conference on World Wide Web, 2012.
[6] Zhu, Yin, et al. "Discovering Spammers in Social Networks", AAAI, 2012.
[7] Ratkiewicz, Jacob, et al, "Truthy: mapping the spread of Astroturf in micro blog streams", ACM, Proceedings of the 20th international conference companion on World Wide Web, pp.249-252, 2011.
[8] Wang, De, D. Irani, and C. Pu, "A social-spam detection framework”, ACM, Proceedings of the 8th Annual Collaboration, Electronic Messaging, Anti-Abuse and Spam Conference, 2011.
[9] Theobald, Martin, J. Siddharth, and A. Paepcke, "Spotsigs: robust and efficient near duplicate detection in large web collections", ACM, Proceedings of the 31st annual international ACM SIGIR conference on Research and development in information retrieval, ACM, 2008.
[10] Chowdhury, Abdur, et al. "Collection statistics for fast duplicate document detection", ACM, Transactions on Information Systems (TOIS), Vol.20, issue.2, pp.171-191, 2002.
[11] G. Jain, Manisha, B. Agarwal, “Spam Detection on Social Media Text”, International Journal of Computer Sciences and Engineering, Vol.5, issue.5, May 2017