Social link prediction using category based location history in trajectory data
Revathy S B1 , emya R2
- College Of Engineering Perumon, APJ Abdul Kalam Technological University,kerala ,India.
- College Of Engineering Perumon, APJ Abdul Kalam Technological University,kerala ,India.
Correspondence should be addressed to: revathysinoj244@gmail.com.
Section:Research Paper, Product Type: Journal Paper
Volume-5 ,
Issue-11 , Page no. 167-170, Nov-2017
CrossRef-DOI: https://doi.org/10.26438/ijcse/v5i11.167170
Online published on Nov 30, 2017
Copyright © Revathy S B, Remya R . This is an open access article distributed under the Creative Commons Attribution License, which permits unrestricted use, distribution, and reproduction in any medium, provided the original work is properly cited.
View this paper at Google Scholar | DPI Digital Library
How to Cite this Paper
- IEEE Citation
- MLA Citation
- APA Citation
- BibTex Citation
- RIS Citation
IEEE Style Citation: Revathy S B, Remya R, “Social link prediction using category based location history in trajectory data,” International Journal of Computer Sciences and Engineering, Vol.5, Issue.11, pp.167-170, 2017.
MLA Style Citation: Revathy S B, Remya R "Social link prediction using category based location history in trajectory data." International Journal of Computer Sciences and Engineering 5.11 (2017): 167-170.
APA Style Citation: Revathy S B, Remya R, (2017). Social link prediction using category based location history in trajectory data. International Journal of Computer Sciences and Engineering, 5(11), 167-170.
BibTex Style Citation:
@article{B_2017,
author = {Revathy S B, Remya R},
title = {Social link prediction using category based location history in trajectory data},
journal = {International Journal of Computer Sciences and Engineering},
issue_date = {11 2017},
volume = {5},
Issue = {11},
month = {11},
year = {2017},
issn = {2347-2693},
pages = {167-170},
url = {https://www.ijcseonline.org/full_paper_view.php?paper_id=1560},
doi = {https://doi.org/10.26438/ijcse/v5i11.167170}
publisher = {IJCSE, Indore, INDIA},
}
RIS Style Citation:
TY - JOUR
DO = {https://doi.org/10.26438/ijcse/v5i11.167170}
UR - https://www.ijcseonline.org/full_paper_view.php?paper_id=1560
TI - Social link prediction using category based location history in trajectory data
T2 - International Journal of Computer Sciences and Engineering
AU - Revathy S B, Remya R
PY - 2017
DA - 2017/11/30
PB - IJCSE, Indore, INDIA
SP - 167-170
IS - 11
VL - 5
SN - 2347-2693
ER -
![]() |
![]() |
![]() |
527 | 1835 downloads | 252 downloads |
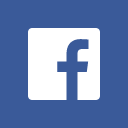
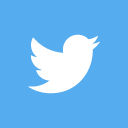
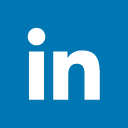
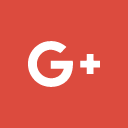
Abstract
With the rising popularity of location-based services, trajectory data mining became an important research topic. There exists many data mining algorithms for systematic processing, managing and mining of trajectory data. Trajectory data mining has many applications such as location recommandations, social link prediction, movement behaviour analysis etc. Here proposes a contextual trajectory analysis model which provides a flexible way to characterize the complex moving nature of humans. It embed multiple contextual information for efficiently modeling data. It includes user-level, trajectory-level, location-level, and temporal-level contexts. It can be used to predict the future location of a user based on the previous travelling pattern. Social link prediction aims to find out whether there exists reciprocal link between two users. Here also propose a method for social link prediction from trajectory data by analyzing the nearest neighbour. This method considers the tf-idf metrics as the baseline.
Key-Words / Index Term
Trajectory,contextual information,social link prediction
References
[1]Ningnan Zhou, Wayne Xin Zhao, Xiao Zhang, Ji-RongWen, Shan Wang, “A General Multi-Context Embedding Model for Mining Human Trajectory Data” IEEE Trans on Knowledge and Data Engineering , Vol. 28, No. 8, pp.1945-1958, 2016.
[2] H. Pham, C. Shahabi, and Y. Liu, “Ebm: An entropy-based model to infer social strength from spatiotemporal data,” in Proc. ACM SIGMOD Int. Conf. Manage. Data, pp. 265–276, 2013.
[3] Q. Li, Y. Zheng, X. Xie, Y. Chen, W. Liu, and W.-Y. Ma, “Mining user similarity based on location history,” in Proc. Annu. ACM Int. Symp. Adv. Geographic Inf. Syst., pp. 34:1–34:10, 2008.
[4] X. Xiao, Y. Zheng, Q. Luo, and X. Xie, “Finding similar users using category-based location history,” in Proc. Annu. ACM Int. Symp. Adv. Geographic Inf. Syst., pp. 442–445, 2010.
[5]E. Cho, S. A. Myers, and J. Leskovec ,” Friendship and mobility: User movement in location-based social networks”, in Proc. ACM SIGKDD Int. Conf. Knowl. Discovery Data Mining, pp. 1082– 1090, 2011.
[6] B. Perozzi, R. A.-Rfou, and S. Skiena, “Deepwalk: Online learning of social representations,” in Proc. ACM SIGKDD Int. Conf. Knowl. Discovery Data Mining, pp. 701–710, 2014.
[7] D. Wang, D. Pedreschi, C. Song, F. Giannotti, and A.-L. Barabasi, “Human mobility, social ties, and link prediction,” in Proc. ACM SIGKDD Int. Conf. Knowl. Discovery Data Mining, pp. 1100–1108, 2011.
[8] W. Mathew, R. Raposo, and B. Martins, “ Predicting future locations with hidden markov models,” in Proc. Int. Joint Conf. Pervasive Ubiquitous Comput., pp. 911–918, 2012.
[9] G.Sivaiah, P.K.Rao,”A comprehensive survey on providing efficient directions using GPS and driver’s ability”, International Journal of Computer Sciences and Engineering, Volume-2, pp. 79-82, 2014.