Intruder Detection Using Fuzzy Min-Max Neural Network and A Principal Component Analysis (PCA) in Network Data
A. F. Aldubai1 , V. H. Humbe2 , S.S. Chowhan3 , Y.F. Aldubai4
- Department of Computational Sciences and Technology, S.R.T.M University, Nanded, India.
- School of Technology, Sub-Center Latur S.R.T.M University, latur, India.
- Department of Computational Sciences and Technology, S.R.T.M University, Nanded , India.
- Faculty of Administrative Sciences, Albaydaa University, Radaa , Yemen.
Correspondence should be addressed to: Ahmed_Aldubai86@yahoo.com.
Section:Research Paper, Product Type: Journal Paper
Volume-5 ,
Issue-12 , Page no. 109-117, Dec-2017
CrossRef-DOI: https://doi.org/10.26438/ijcse/v5i12.109117
Online published on Dec 31, 2017
Copyright © A. F. Aldubai, V. H. Humbe, S.S. Chowhan, Y.F. Aldubai . This is an open access article distributed under the Creative Commons Attribution License, which permits unrestricted use, distribution, and reproduction in any medium, provided the original work is properly cited.
View this paper at Google Scholar | DPI Digital Library
How to Cite this Paper
- IEEE Citation
- MLA Citation
- APA Citation
- BibTex Citation
- RIS Citation
IEEE Style Citation: A. F. Aldubai, V. H. Humbe, S.S. Chowhan, Y.F. Aldubai, “Intruder Detection Using Fuzzy Min-Max Neural Network and A Principal Component Analysis (PCA) in Network Data,” International Journal of Computer Sciences and Engineering, Vol.5, Issue.12, pp.109-117, 2017.
MLA Style Citation: A. F. Aldubai, V. H. Humbe, S.S. Chowhan, Y.F. Aldubai "Intruder Detection Using Fuzzy Min-Max Neural Network and A Principal Component Analysis (PCA) in Network Data." International Journal of Computer Sciences and Engineering 5.12 (2017): 109-117.
APA Style Citation: A. F. Aldubai, V. H. Humbe, S.S. Chowhan, Y.F. Aldubai, (2017). Intruder Detection Using Fuzzy Min-Max Neural Network and A Principal Component Analysis (PCA) in Network Data. International Journal of Computer Sciences and Engineering, 5(12), 109-117.
BibTex Style Citation:
@article{Aldubai_2017,
author = {A. F. Aldubai, V. H. Humbe, S.S. Chowhan, Y.F. Aldubai},
title = {Intruder Detection Using Fuzzy Min-Max Neural Network and A Principal Component Analysis (PCA) in Network Data},
journal = {International Journal of Computer Sciences and Engineering},
issue_date = {12 2017},
volume = {5},
Issue = {12},
month = {12},
year = {2017},
issn = {2347-2693},
pages = {109-117},
url = {https://www.ijcseonline.org/full_paper_view.php?paper_id=1588},
doi = {https://doi.org/10.26438/ijcse/v5i12.109117}
publisher = {IJCSE, Indore, INDIA},
}
RIS Style Citation:
TY - JOUR
DO = {https://doi.org/10.26438/ijcse/v5i12.109117}
UR - https://www.ijcseonline.org/full_paper_view.php?paper_id=1588
TI - Intruder Detection Using Fuzzy Min-Max Neural Network and A Principal Component Analysis (PCA) in Network Data
T2 - International Journal of Computer Sciences and Engineering
AU - A. F. Aldubai, V. H. Humbe, S.S. Chowhan, Y.F. Aldubai
PY - 2017
DA - 2017/12/31
PB - IJCSE, Indore, INDIA
SP - 109-117
IS - 12
VL - 5
SN - 2347-2693
ER -
![]() |
![]() |
![]() |
853 | 525 downloads | 188 downloads |
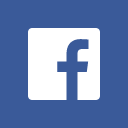
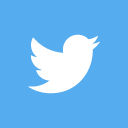
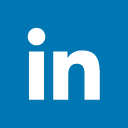
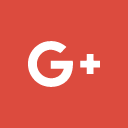
Abstract
To improve classification performance and prediction time of (IDS) system when a few number of large hyperboxes are formed in the network. We have proposed FMM NN and PCA features extraction algorithms. The process of a system consisting of the pre-processing dataset based on interquartile ranges filter and features extraction. Followed by the Fuzzy min-max neural network (FMM NN), which define as supervised learning classifier, that utilizes a fuzzy set hyberbox as pattern classes for learning and classification. Each hyberbox consists of min and mix points of opposite corners of hyberbox with corresponding to membership function. Two real-time and faster streaming datasets (KDD99 and NSL-KDD) are used to empirically evaluate the effectiveness of the proposed FMM NN system. The results are analyzed and compared with others existing systems and published results.
Key-Words / Index Term
Intrusion Detection, Fuzzy min-max neural network, Principal Component Analysis (PCA), Machine Learning
References
[1] Ahmed Fuad Mohammed, Vikas T. Humbe, Santosh S. Chowhan, "Analytical Study of Intruder Detection System in Big Data Environment " Soft Computing: Theories and Applications SoCTA 2016, Volume 2, 2016.
[2] Khattab M. Ali Alheeti, Anna Gruebler, Klaus D. McDonald-Maier, "An Intrusion Detection System against Black Hole Attacks on the Communication Network of Self-Driving Cars", Emerging Security Technologies (EST), 2015 Sixth International Conference on, Publisher: IEEE, 10 March 2016.
[3] JABEZ Ja , Dr.B.MUTHUKUMAR, "Intrusion Detection System (IDS): Anomaly Detection using Outlier Detection Approach ", International Conference on Intelligent Computing, Communication & Convergence (ICCC-2014), Procedia Computer Science 338 – 346 Publisher: Science Direct, 2015.
[4] Modi, Chirag N., Dhiren R. Patel, Avi Patel, and Rajarajan Muttukrishnan. "Bayesian Classifier and Snort based network intrusion detection system in cloud computing", 2012 Third International Conference on Computing Communication and Networking Technologies (ICCCNT 12), 2012.
[5] Ahmed Fuad Mohammed, Vikas T. Humbe, Santosh S. Chowhan, "Data Mining Based Traffic Classification Using Low-Level Features" International Journal of Computer Applications (0975 – 8887), 2017.
[6] Azad C, Jha VK , "Data mining based hybrid intrusion detection system", Indian J Sci Technol 7(6):781–789, 2014.
[7] Chen T, Zhang X, Jin S, Kim O , "Efficient classification using parallel and scalable compressed model and its application on intrusion detection", Expert Syst Appl 41(13):5972–5983, 2014.
[8] Gu B, Guo H, "The intrusion detection system based on a novel association rule", In: International conference on information science, electronics and electrical engineering (ISEEE), vol. 2, pp 1313–1316, 2014.
[9] Anas Quteishat, Chee Peng Lim. " A modified fuzzy min–max neural network with rule extraction and its application to fault detection and classification", Elsevier, Applied Soft Computing 8 (2008) 985–995.
[10] Liao S-H, Chu P-H, Hsiao P-Y, "Data mining techniques and applications–a decade review from 2000 to 2011", Expert Syst Appl 39(12):11303–11311, 2012.
[11] Julisch K, "Data mining for intrusion detection. Applications of data mining in computer security". Springer, US, Fuzzy Min-Max Neural Network-Based Intrusion Detection System pp 33–62, 2002.
[12] Wang G, Hao J, Ma J, Huang L "A new approach to intrusion detection using Artificial Neural Networks and fuzzy clustering.Expert Syst" Appl 37(9):6225–6232.
[13] Chandrashekhar Azad, Vijay Kumar Jha, "Fuzzy min–max neural network and particle swarm optimization based intrusion detection system", Springer-Verlag Berlin Heidelberg 2016.
[14] . Lei JZ, Ghorbani AA , "Improved competitive learning neural networks for network intrusion and fraud detection", Neurocomputing 75(1)135–145.
[15] Basant Subba , Santosh Biswas, Sushanta Karmakar, "A Neural Network Based System for Intrusion Detection and Attack Classification", 978-1-5090-2361-5/16/$31.00 c 2016 IEEE, 08 September 2016.
[16] Azad Chandrashekhar, "Fuzzy Min-Max Neural Network-Based Intrusion Detection System", © Springer Nature Singapore Pte Ltd. PP 191-201, 2017.
[17] L. A. Zadeh, "Fuzzy Sets", Department of Electrical Engineering and Electronics Research Laboratory, University of California, Berkeley, California.
[18] Simpson PK, "Fuzzy min-max neural networks. I. classification", IEEE Trans Neural Networks 3(5), 776–786, 1992.
[19] Anjay Krishnankutty Alonso, eMath teacher for MAMBANI`S FUZZY INFERENCE MMETHOD, Retrievedfrom,http://www.dma.fi.upm.es/recursos/aplicaciones/logica_borrosa/web/fuzzy_inferencia/funpert_en.htm, (2017, Oct, 19)
[20] Pfahringer B Winning, " the KDD99 classification cup: bagged boosting", ACM SIGKDD Explor Newsl 1(2):65–66, 2000.
[21] Levin I, KDD-99, "classifier learning contest: LLSoft’s results overview", SIGKDD Explor 1(2):67–75, 2000.