Identification of Psychological Harassment Via Digital Communication Media
Manorama Singh1 , Abhay Kumar2
- Dept. of CSE, J.B. Institute of Engineering and Technology, Hyderabad, India.
- Dept. of CSE, J.B. Institute of Engineering and Technology, Hyderabad, India.
Section:Review Paper, Product Type: Journal Paper
Volume-5 ,
Issue-12 , Page no. 147-150, Dec-2017
CrossRef-DOI: https://doi.org/10.26438/ijcse/v5i12.147150
Online published on Dec 31, 2017
Copyright © Manorama Singh, Abhay Kumar . This is an open access article distributed under the Creative Commons Attribution License, which permits unrestricted use, distribution, and reproduction in any medium, provided the original work is properly cited.
View this paper at Google Scholar | DPI Digital Library
How to Cite this Paper
- IEEE Citation
- MLA Citation
- APA Citation
- BibTex Citation
- RIS Citation
IEEE Style Citation: Manorama Singh, Abhay Kumar, “Identification of Psychological Harassment Via Digital Communication Media,” International Journal of Computer Sciences and Engineering, Vol.5, Issue.12, pp.147-150, 2017.
MLA Style Citation: Manorama Singh, Abhay Kumar "Identification of Psychological Harassment Via Digital Communication Media." International Journal of Computer Sciences and Engineering 5.12 (2017): 147-150.
APA Style Citation: Manorama Singh, Abhay Kumar, (2017). Identification of Psychological Harassment Via Digital Communication Media. International Journal of Computer Sciences and Engineering, 5(12), 147-150.
BibTex Style Citation:
@article{Singh_2017,
author = {Manorama Singh, Abhay Kumar},
title = {Identification of Psychological Harassment Via Digital Communication Media},
journal = {International Journal of Computer Sciences and Engineering},
issue_date = {12 2017},
volume = {5},
Issue = {12},
month = {12},
year = {2017},
issn = {2347-2693},
pages = {147-150},
url = {https://www.ijcseonline.org/full_paper_view.php?paper_id=1594},
doi = {https://doi.org/10.26438/ijcse/v5i12.147150}
publisher = {IJCSE, Indore, INDIA},
}
RIS Style Citation:
TY - JOUR
DO = {https://doi.org/10.26438/ijcse/v5i12.147150}
UR - https://www.ijcseonline.org/full_paper_view.php?paper_id=1594
TI - Identification of Psychological Harassment Via Digital Communication Media
T2 - International Journal of Computer Sciences and Engineering
AU - Manorama Singh, Abhay Kumar
PY - 2017
DA - 2017/12/31
PB - IJCSE, Indore, INDIA
SP - 147-150
IS - 12
VL - 5
SN - 2347-2693
ER -
![]() |
![]() |
![]() |
737 | 369 downloads | 196 downloads |
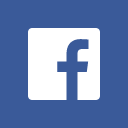
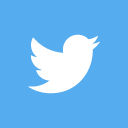
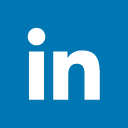
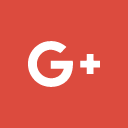
Abstract
Although the Internet has transformed the way our world operates, it has also served as a venue for cyberbullying, a serious form of misbehavior among youth. With many of today`s youth experiencing acts of cyberbullying [2], a growing body of literature has begun to document the prevalence, predictors, and outcomes of this behavior, but the literature is highly fragmented and lacks theoretical focus. Therefore, our purpose in the present article [1] is to provide a critical review of the existing cyberbullying research. This systematic review and meta-analysis [6][7] offers a synthesis of the relationship between cyber-victimization and educational outcomes of adolescents aged 12 to 17, including 25 effect sizes from 12 studies drawn from a variety of disciplines. The general aggression model is proposed as a useful theoretical framework from which to understand this phenomenon. Additionally, results from a meta-analytic review are presented to highlight the size of the relationships between cyberbullying and traditional bullying, as well as relationships between cyberbullying and other meaningful behavioral and psychological variables. A series of random-effects meta-analyses [12] using robust variance estimation revealed associations between cyber-victimization [4] and higher class presence problems (r = .20) and academic achievement problems (r = .14). Results did not differ by provided definition, publication status, reporting time frame, gender, race/ethnicity, or average age. Implications for future research are discussed within context of theoretical, critical, and applied discussions.
Key-Words / Index Term
cyber-victimization, victimization, meta-analysis, adolescents, academic achievement, school attendance, Cyberbullying Detection, Text Mining, Representation Learning
References
[1]. P. Baldi, “Autoencoders, unsupervised learning, and deep architectures,” Unsupervised and Transfer Learning Challenges in Machine Learning, Volume 7, p. 43, 2012.
[2]. M. Chen, Z. Xu, K. Weinberger, and F. Sha, “Marginalized denoising autoencoders for domain adaptation,” arXiv preprint arXiv: 1206.4683, 2012.
[3]. T. K. Landauer, P. W. Foltz, and D. Laham, “An introduction to latent semantic analysis,” Discourse processes, vol. 25, no. 2-3, pp. 259–284, 1998.
[4]. T. L. Griffiths and M. Steyvers, “Finding scientific topics,” Proceedings of the National academy of Sciences of the United States of America, vol. 101, no. Suppl 1, pp. 5228–5235, 2004.
[5]. D. M. Blei, A. Y. Ng, and M. I. Jordan, “Latent dirichlet allocation,” the Journal of machine Learning research, vol. 3, pp. 993–1022, 2003.
[6]. T. Hofmann, “Unsupervised learning by probabilistic latent semantic analysis,” Machine learning, vol. 42, no. 1-2, pp. 177–196, 2001.
[7]. Y. Bengio, A. Courville, and P. Vincent, “Representation learning: A review and new perspectives,” Pattern Analysis and Machine Intelligence, IEEE Transactions on, vol. 35, no. 8, pp. 1798–1828, 2013.
[8]. B. L. McLaughlin, A. A. Braga, C. V. Petrie, M. H. Moore et al., Deadly Lessons:: Understanding Lethal School Violence. National Academies Press, 2002.
[9]. J. Juvonen and E. F. Gross, “Extending the school grounds? bullying experiences in cyberspace,” Journal of School health, vol. 78, no. 9, pp. 496–505, 2008.
[10]. M. Fekkes, F. I. Pijpers, A. M. Fredriks, T. Vogels, and S. P. Verloove-Vanhorick, “Do bullied children get ill, or do ill children get bullied? a prospective cohort study on the relationship between bullying and health-related symptoms,” Pediatrics, vol. 117, no. 5, pp. 1568–1574, 2006.
[11]. M. Ptaszynski, F. Masui, Y. Kimura, R. Rzepka, and K. Araki, “Brute force works best against bullying,” in Proceedings of IJCAI 2015 Joint Workshop on Constraints and Preferences for Configuration and Recommendation and Intelligent Techniques for Web Personalization. ACM, 2015.
[12]. R. Tibshirani, “Regression shrinkage and selection via the lasso,” Journal of the Royal Statistical Society. Series B (Methodological), pp. 267–288, 1996.
[13]. P. Vincent, H. Larochelle, I. Lajoie, Y. Bengio, and P.-A. Manzagol, “Stacked denoising autoencoders: Learning useful representations in a deep network with a local denoising criterion,” The Journal of Machine Learning Research, vol. 11, pp. 3371–3408, 2010.
[14]. P. Baldi, “Autoencoders, unsupervised learning, and deep architectures,” Unsupervised and Transfer Learning Challenges in Machine Learning, Volume 7, p. 43, 2012.
[15]. M. Chen, Z. Xu, K. Weinberger, and F. Sha, “Marginalized denoising autoencoders for domain adaptation,” arXiv preprint arXiv: 1206.4683, 2012.
[16]. T. K. Landauer, P. W. Foltz, and D. Laham, “An introduction to latent semantic analysis,” Discourse processes, vol. 25, no. 2-3, pp. 259–284, 1998.
[17]. T. L. Griffiths and M. Steyvers, “Finding scientific topics,” Proceedings of the National academy of Sciences of the United States of America, vol. 101, no. Suppl 1, pp. 5228–5235, 2004.
[18]. D. M. Blei, A. Y. Ng, and M. I. Jordan, “Latent dirichlet allocation,” the Journal of machine Learning research, vol. 3, pp. 993–1022, 2003.
[19]. T. Hofmann, “Unsupervised learning by probabilistic latent semantic analysis,” Machine learning, vol. 42, no. 1-2, pp. 177–196, 2001.