Analysis of Multilevel-Semantic Prediction based on User Point of Sentiment Opinion (MSP-UPSO) in Social Web Mining
A. Muruganantham1 , S. P. Victor2
- Department of Computer Science, Kristu Jayanti College (Autonomous) [ Bangalore University], Bengaluru, India.
- Department of Computer Science, St. Xavier’s College (Autonomous)[Manonmaniam Sundaranar University], Tirunelveli, India.
Correspondence should be addressed to: murushr@hotmail.com.
Section:Research Paper, Product Type: Journal Paper
Volume-5 ,
Issue-12 , Page no. 151-158, Dec-2017
CrossRef-DOI: https://doi.org/10.26438/ijcse/v5i12.151158
Online published on Dec 31, 2017
Copyright © A. Muruganantham, S. P. Victor . This is an open access article distributed under the Creative Commons Attribution License, which permits unrestricted use, distribution, and reproduction in any medium, provided the original work is properly cited.
View this paper at Google Scholar | DPI Digital Library
How to Cite this Paper
- IEEE Citation
- MLA Citation
- APA Citation
- BibTex Citation
- RIS Citation
IEEE Style Citation: A. Muruganantham, S. P. Victor, “Analysis of Multilevel-Semantic Prediction based on User Point of Sentiment Opinion (MSP-UPSO) in Social Web Mining,” International Journal of Computer Sciences and Engineering, Vol.5, Issue.12, pp.151-158, 2017.
MLA Style Citation: A. Muruganantham, S. P. Victor "Analysis of Multilevel-Semantic Prediction based on User Point of Sentiment Opinion (MSP-UPSO) in Social Web Mining." International Journal of Computer Sciences and Engineering 5.12 (2017): 151-158.
APA Style Citation: A. Muruganantham, S. P. Victor, (2017). Analysis of Multilevel-Semantic Prediction based on User Point of Sentiment Opinion (MSP-UPSO) in Social Web Mining. International Journal of Computer Sciences and Engineering, 5(12), 151-158.
BibTex Style Citation:
@article{Muruganantham_2017,
author = {A. Muruganantham, S. P. Victor},
title = {Analysis of Multilevel-Semantic Prediction based on User Point of Sentiment Opinion (MSP-UPSO) in Social Web Mining},
journal = {International Journal of Computer Sciences and Engineering},
issue_date = {12 2017},
volume = {5},
Issue = {12},
month = {12},
year = {2017},
issn = {2347-2693},
pages = {151-158},
url = {https://www.ijcseonline.org/full_paper_view.php?paper_id=1595},
doi = {https://doi.org/10.26438/ijcse/v5i12.151158}
publisher = {IJCSE, Indore, INDIA},
}
RIS Style Citation:
TY - JOUR
DO = {https://doi.org/10.26438/ijcse/v5i12.151158}
UR - https://www.ijcseonline.org/full_paper_view.php?paper_id=1595
TI - Analysis of Multilevel-Semantic Prediction based on User Point of Sentiment Opinion (MSP-UPSO) in Social Web Mining
T2 - International Journal of Computer Sciences and Engineering
AU - A. Muruganantham, S. P. Victor
PY - 2017
DA - 2017/12/31
PB - IJCSE, Indore, INDIA
SP - 151-158
IS - 12
VL - 5
SN - 2347-2693
ER -
![]() |
![]() |
![]() |
1115 | 504 downloads | 283 downloads |
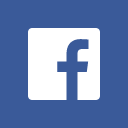
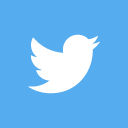
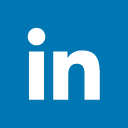
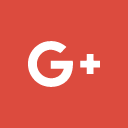
Abstract
Web mining is a tremendous growth in social networks for intent knowledge of various information’s, comments, reviews, tags about choosing concern life objects, there is an increasing demand for data prediction because of a different point of user interest i.e.,” think to best”. The users will be trusted to using these online reviews and to get information about the opinions of products from others. Due to the reviews product was successive based on threating of user point opinion regarding the given object. The social comment should the hidden sentiments of the user opinions. A most problematic challenge in web mining is to identify the sentiments and aspects opinion of the people to perform the data classification based on these features Opinion summarization. To propose a multilevel semantic pattern incremental algorithm (MSPI) with intent to divisive rank clusters which they analyze the hidden user opinion sentiments and classifies the user hidden points. Initially to preprocess the product reviews and to apply the multilevel semantic analyzer form extraction data points. The data points are ranked based on algometric rank clusters to optimized result case. The data sources to be taken for the social web from a customer review of the product list. In this paper, experiments were conducted to compare the performance of existing clustering and classification algorithm produce higher prediction rate based on hidden sentiment case reviews.
Key-Words / Index Term
web mining, opinion mining, sentiment analysis, clustering, rank prediction
References
[1] M. L. Felciah, R.Anbuselvi.,” A Study on Sentiment Analysis of Social Media Reviews” IEEE Sponsored 2nd International Conference on Innovations in Information Embedded and Communication Systems,india,2015.
[2] ZaipingTao, “An approach towards comprehensive sentimental data analysis and opinion mining,"IEEE Transactions on Learning Technologies,vol 5, Issue 30,pp,215-228,2013.
[3] Z.Zhai et al,"Clustering Product Feature for Opinion Mining", IEEE Transactions on Knowledge and Data Engineering,vol 7,Issue 28,pp.112-139 ,20ll.
[4] R.Lijun, "Research on the opinion mining system for 5 Massive Social Media Data" Internationl natural language processing,vol10,issue 40,pp.424-431,2013, Springer.
[5] Blessy Selvam,"A Survey on Opinion Mining FrameWork", International Journal of Advanced Research in computer and communication Engineering, vol 2, Issue 9, 2013.
[6] B Vijay. Raut, .D. Londhe,” Survey on Opinion Mining and Summarization of User Reviews on Web” International Journal of Computer Science and Information Technologie, vol 17,issue 5,pp.564-579,2014.
[7] R Soni1, K. J. Mathai” Effective Sentiment Analysis of a Launched Product using Clustering and Decision Trees” International Journal of Innovative Research in Computer and Communication Engineering, Vol. 4, Issue 1,2016
[8] B. Burscher, R. Vliegenthart, H. Claes, “Frames Beyond Words: Applying Cluster and Sentiment Analysis to News Coverage of the Nuclear Power,” International Social Science Computer Research,vol 2,Issue 32 pp. 1-16, 2015
[9] H Suresh, G Raj . “A Novel Cluster-Based Unsupervised Technique for Twitter Sentiment Analysis”, International Journal of Innovative Research in Computer and Communication Engineering, Vol. 6, Issue 7,pp.345,362, 2017
[10] L.Zhu, A. Galstyan, “Tripartite Graph clustering for Dynamic Sentiment Analysis on Social Media”, International Social and Information Networks,’ vol 14, pp.1531-1542, June. 2014.
[11] L. N. Ferreira, L. Zhao, “ A Time series Clustering Technique based on Community Detection in Networks”, Proceeding Computer Science, Elsevier, pp. 183-190, 2015. International Journal of Advanced Research in CS and Software Engineering, Vol. 5, Issue 8, 2015.
[12] L .F .S. Coletta, N. F. F da Silva, E. R. Hruschka, E. R. Hruschka, “ Combining Classification and Clustering for Tweet Sentiment Analysis”,Brazilian journal on Intelligent Systems,vol 2,Issue 12,pp784,797 2016.
[13] M. Unnisa, A. Ameen, S. Raziuddin, “ Opinion Mining on Twitter Data using Unsupervised Learning Technique “, International Journal of Computer Applications, Vol. 148, No.12, pp.12-19, 2016.
[14] N. Pooranam, G. Shyamala, “A Statistical Method of Knowledge Extraction on Online Stock Forum Using Subspace Clustering with Outlier Detection”, International Journal of Innovative Research in Science, Engineering and Technology, Vol.5, Issue 5, May.2016.
[15] A.Shukla, R. Misra, “Sentiment Classification and Analysis using Modified K-Means and Naïve Bayes Algorithm”, International Journal of Computer Applications, Vol. 86, No.22, pp.43-58, 2015.
[16] Xiaolong Wang, Furu Wei, Xiaohua Liu, Ming Zhou, Ming Zhang,” Topic-Sentiment Analysis in Twitter: A Graph-based Hashtag Sentiment Classification Approach”, ACM, CIKM’11, October 24–28, 2011.
[17] Glasgow, Scotland, UK, 2011.Andrius Mudinas, Dell Zhang, Mark Levene,” Combining Lexicon and Learning based Approaches for Concept-Level Sentiment Analysis”, IEEE Transactions on Affective Computing, vol 4,issue 99,pp.15-32 ,2012.
[18] L.williams ,” Idiom based features in sentiment analysis: Cutting the Gordian knot”, IEEE Trans. On knowledge and data engineering, vol. 24, no. 4, 2012.
[19] D park, S.kim“ConceptVector: Text Visual Analytics via Interactive Lexicon Building Using Word Embedding” IEEE Transactions on Visualization and Computer Graphics , Vol 24, Issue 1, 361 – 370, 2018.
[20] R.Xia , J.Jiang, and H.He, “Distantly Supervised Lifelong Learning for Large-Scale Social Media Sentiment Analysis” IEEE transactions on affective computing, vol. 8, no. 4 ,pp. 751 – 770,2017