A Survey on knowledge extraction Approaches from Big Data and Rectifying Misclassification strategies
Jyoti Arora1 , Ambica Sood2
- Computer Science Engineering, Chitkara University, Kalu Jhanda, India.
- Computer Science Engineering, Chandigarh University, Gharuan, India.
Correspondence should be addressed to: jyoti@chitkarauniversity.edu.in.
Section:Survey Paper, Product Type: Journal Paper
Volume-5 ,
Issue-12 , Page no. 187-200, Dec-2017
CrossRef-DOI: https://doi.org/10.26438/ijcse/v5i12.187200
Online published on Dec 31, 2017
Copyright © Jyoti Arora, Ambica Sood . This is an open access article distributed under the Creative Commons Attribution License, which permits unrestricted use, distribution, and reproduction in any medium, provided the original work is properly cited.
View this paper at Google Scholar | DPI Digital Library
How to Cite this Paper
- IEEE Citation
- MLA Citation
- APA Citation
- BibTex Citation
- RIS Citation
IEEE Style Citation: Jyoti Arora, Ambica Sood, “A Survey on knowledge extraction Approaches from Big Data and Rectifying Misclassification strategies,” International Journal of Computer Sciences and Engineering, Vol.5, Issue.12, pp.187-200, 2017.
MLA Style Citation: Jyoti Arora, Ambica Sood "A Survey on knowledge extraction Approaches from Big Data and Rectifying Misclassification strategies." International Journal of Computer Sciences and Engineering 5.12 (2017): 187-200.
APA Style Citation: Jyoti Arora, Ambica Sood, (2017). A Survey on knowledge extraction Approaches from Big Data and Rectifying Misclassification strategies. International Journal of Computer Sciences and Engineering, 5(12), 187-200.
BibTex Style Citation:
@article{Arora_2017,
author = {Jyoti Arora, Ambica Sood},
title = {A Survey on knowledge extraction Approaches from Big Data and Rectifying Misclassification strategies},
journal = {International Journal of Computer Sciences and Engineering},
issue_date = {12 2017},
volume = {5},
Issue = {12},
month = {12},
year = {2017},
issn = {2347-2693},
pages = {187-200},
url = {https://www.ijcseonline.org/full_paper_view.php?paper_id=1601},
doi = {https://doi.org/10.26438/ijcse/v5i12.187200}
publisher = {IJCSE, Indore, INDIA},
}
RIS Style Citation:
TY - JOUR
DO = {https://doi.org/10.26438/ijcse/v5i12.187200}
UR - https://www.ijcseonline.org/full_paper_view.php?paper_id=1601
TI - A Survey on knowledge extraction Approaches from Big Data and Rectifying Misclassification strategies
T2 - International Journal of Computer Sciences and Engineering
AU - Jyoti Arora, Ambica Sood
PY - 2017
DA - 2017/12/31
PB - IJCSE, Indore, INDIA
SP - 187-200
IS - 12
VL - 5
SN - 2347-2693
ER -
![]() |
![]() |
![]() |
1354 | 662 downloads | 274 downloads |
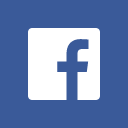
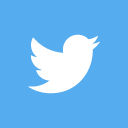
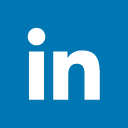
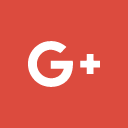
Abstract
The amount of data is increasing now a days due to usage of portable resources like smart phones, tablets and many more for accessing social sites. The requirement to analyze such big data to extract meaningful data came into existence. Traditional methods have been explored by number of researchers to analyze such data. These methods removed faulty data, uncertain data or misclassified data for better analyses. But this leads to loss of data. There is need to take into consideration the rectification of uncertainty in aspect of big datasets also. So, In this paper we survey big data, some traditional methods for data analyses, advance methods for data analyses, issues related to these methods, misclassification concept, the survey of rectification techniques for high accuracy followed by bearer future scope.
Key-Words / Index Term
Big Data, Misclassification, Machine Learning, Knowledge, Discovery, Mining
References
[1] Weiss, Sholom M., and Nitin Indurkhya. Predictive data mining: a practical guide. Morgan Kaufmann, 1998.
[2] Basu, Sugato, and Prem Melville. "Weka Tutorial." ht-tp://www. cs. utexas. edu/users/ml/tutorials/Weka-tut.
[3] Fisher, Danyel, Rob DeLine, Mary Czerwinski, and Steven Drucker. "Interactions with big data analytics." interactions 19, no. 3 (2012): 50-59.
[4] Molodtsov, Dmitriy. "Soft set theory—first results." Computers & Mathematics with Applications 37, no. 4-5 (1999): 19-31.
[5] Marr, Bernard. "Big Data: 20 Mind-Boggling Facts Everyone Must Read." Forbes Magazine (2015).
[6] https://www.modeln.com/blog/high-tech/2016/10-interesting-facts-big-data/
[7] Tole, Alexandru Adrian. "Big data challenges." Database Systems Journal 4, no. 3 (2013): 31-40.
[8] Gantz, John, and David Reinsel. "The digital universe in 2020: Big data, bigger digital shadows, and biggest growth in the far east." IDC iView: IDC Analyze the future 2007, no. 2012 (2012): 1-16.
[9] Fisher, Danyel, Rob DeLine, Mary Czerwinski, and Steven Drucker. "Interactions with big data analytics." interactions 19, no. 3 (2012): 50-59.
[10] Press, Gil. "$16.1 billion big data market: 2014 predictions from IDC and IIA." Forbes. com (2013).
[11] Big data and analytics—an IDC four pillar research area, IDC, Tech. Rep. 2013.
[12] Laney, Doug. "3D data management: Controlling data volume, velocity and variety." META Group Research Note 6 (2001): 70.
[13] Sagiroglu, Seref, and Duygu Sinanc. "Big data: A review." In Collaboration Technologies and Systems (CTS), 2013 International Conference on, pp. 42-47. IEEE, 2013.
[14] The Big Bang: How the Big Data Explosion Is Changing the World - Microsoft UK Enterprise Insights Blog - Site Home - MSDN Blogs.
[15] Landset, Sara, Taghi M. Khoshgoftaar, Aaron N. Richter, and Tawfiq Hasanin. "A survey of open source tools for machine learning with big data in the Hadoop ecosystem." Journal of Big Data 2, no. 1 (2015): 24.
[16] Beyer, Mark A., and Douglas Laney. "The Importance of “Big Data”: A Definition. Gartner." (2012).
[17] Hashem, Ibrahim Abaker Targio, Ibrar Yaqoob, Nor Badrul Anuar, Salimah Mokhtar, Abdullah Gani, and Samee Ullah Khan. "The rise of “big data” on cloud computing: Review and open research issues." Information Systems 47 (2015): 98-115.
[18] Khan, Nawsher, Ibrar Yaqoob, Ibrahim Abaker Targio Hashem, Zakira Inayat, Waleed Kamaleldin Mahmoud Ali, Muhammad Alam, Muhammad Shiraz, and Abdullah Gani. "Big data: survey, technologies, opportunities, and challenges." The Scientific World Journal 2014 (2014).
[19] Kaisler, Stephen, Frank Armour, J. Alberto Espinosa, and William Money. "Big data: Issues and challenges moving forward." In System sciences (HICSS), 2013 46th Hawaii international conference on, pp. 995-1004. IEEE, 2013.
[20] Sandryhaila, Aliaksei, and Jose MF Moura. "Big data analysis with signal processing on graphs: Representation and processing of massive data sets with irregular structure." IEEE Signal Processing Magazine 31, no. 5 (2014): 80-90.
[21] Gantz, J., and D. Reinsel. "Extracting value from chaos technical report white paper." International Data Corporation (IDC) Sponsored by EMC Corporation (2011).
[22] Gantz, John, and David Reinsel. "The digital universe decade-are you ready." IDC White Paper (2010): 1-16.
[23] Mitchell, Tom M. "Machine learning. WCB." (1997).
[24] Russell, Stuart, Peter Norvig, and Artificial Intelligence. "A modern approach." Artificial Intelligence. Prentice-Hall, Egnlewood Cliffs 25 (1995): 27.
[25] Cherkassky, Vladimir, and Filip M. Mulier. Learning from data: concepts, theory, and methods. John Wiley & Sons, 2007.
[26] Mitchell, Tom Michael. The discipline of machine learning. Vol. 9. Carnegie Mellon University, School of Computer Science, Machine Learning Department, 2006.
[27] Rudin, Cynthia, and Kiri L. Wagstaff. "Machine learning for science and society." Machine Learning 95, no. 1 (2014): 1-9.
[28] Bishop, Christopher M. "Pattern recognition." Machine Learning 128 (2006): 1-58.
[29] Adam, Bernard, and Ian F. Smith. "Reinforcement learning for structural control." Journal of Computing in Civil Engineering 22, no. 2 (2008): 133-139.
[30] Jones, Nicola. "The learning machines." Nature 505, no. 7482 (2014): 146.
[31] Langford, John. "Tutorial on practical prediction theory for classification." Journal of machine learning research 6, no. Mar (2005): 273-306.
[32] Bekkerman, Ron, Ran El-Yaniv, Naftali Tishby, and Yoad Winter. "Distributional word clusters vs. words for text categorization." Journal of Machine Learning Research 3, no. Mar (2003): 1183-1208.
[33] Burch, Carl. "A survey of machine learning." Tech. report, Pennsylvania Governor`s School for the Sciences (2001).
[34] Zheng, Jun, Furao Shen, Hongjun Fan, and Jinxi Zhao. "An online incremental learning support vector machine for large-scale data." Neural Computing and Applications 22, no. 5 (2013): 1023-1035.
[35] Cortes, Corinna, and Vladimir Vapnik. "Support-vector networks." Machine learning 20, no. 3 (1995): 273-297.
[36] Dong, Xu, Ying Li, Chun Wu, and Yueming Cai. "A learner based on neural network for cognitive radio." In Communication Technology (ICCT), 2010 12th IEEE International Conference on, pp. 893-896. IEEE, 2010.
[37] Safatly, Lise, Mario Bkassiny, Mohammed Al-Husseini, and Ali El-Hajj. "Cognitive radio transceivers: RF, spectrum sensing, and learning algorithms review." International Journal of Antennas and Propagation 2014 (2014).
[38] Galindo-Serrano, Ana, and Lorenza Giupponi. "Distributed Q-learning for aggregated interference control in cognitive radio networks." IEEE Transactions on Vehicular Technology 59, no. 4 (2010): 1823-1834.
[39] Sutton, Richard S. "Learning to predict by the methods of temporal differences." Machine learning 3, no. 1 (1988): 9-44.
[40] O. Okun, G. Valentini, (Eds.), Supervised and Unsupervised Ensemble Methods and their Applications Studies in Computational Intelligence, vol. 126, Springer, Heidelberg, 2008.
[41] Abaei, Golnoush, Ali Selamat, and Hamido Fujita. "An empirical study based on semi-supervised hybrid self-organizing map for software fault prediction." Knowledge-Based Systems 74 (2015): 28-39.
[42] Abdi, Amir, Suvi Heinonen, Christopher Juhlin, and Tuomo Karinen. "Constraints on the geometry of the Suasselkä post-glacial fault, northern Finland, based on reflection seismic imaging." Tectonophysics 649 (2015): 130-138.
[43] Audet, Patrick, Bradley D. Pinno, and Evelyne Thiffault. "Reclamation of boreal forest after oil sands mining: anticipating novel challenges in novel environments." Canadian Journal of Forest Research 45, no. 3 (2014): 364-371.
[44] Bissig, Thomas, Alan H. Clark, Amelia Rainbow, and Allan Montgomery. "Physiographic and tectonic settings of high-sulfidation epithermal gold–silver deposits of the Andes and their controls on mineralizing processes." Ore Geology Reviews 65 (2015): 327-364.
[45] Botros, N. S. "The role of the granite emplacement and structural setting on the genesis of gold mineralization in Egypt." Ore Geology Reviews 70 (2015): 173-187.
[46] Karapetrou, S., M. Manakou, D. Bindi, B. Petrovic, and K. Pitilakis. "“Time-building specific” seismic vulnerability assessment of a hospital RC building using field monitoring data." Engineering Structures 112 (2016): 114-132.
[47] Khan, Salman H., M. Ali Akbar, Farrukh Shahzad, Mudassar Farooq, and Zeashan Khan. "Secure biometric template generation for multi-factor authentication." Pattern Recognition 48, no. 2 (2015): 458-472.
[48] Moss, S., J. Melia, J. Sutton, C. Mathews, and M. Kirby. "Prostate‐specific antigen testing rates and referral patterns from general practice data in England." International journal of clinical practice 70, no. 4 (2016): 312-318.
[49] Naoi, Makoto, Masao Nakatani, Kenshiro Otsuki, Yasuo Yabe, Thabang Kgarume, Osamu Murakami, Thabang Masakale et al. "Steady activity of microfractures on geological faults loaded by mining stress." Tectonophysics 649 (2015): 100-114.
[50] Pavel, Ana B., Dmitriy Sonkin, and Anupama Reddy. "Integrative modeling of multi-omics data to identify cancer drivers and infer patient-specific gene activity." BMC systems biology 10, no. 1 (2016): 16.
[51] Sang, Jitao, Yue Gao, Bing-kun Bao, Cees Snoek, and Qionghai Dai. "Recent advances in social multimedia big data mining and applications." Multimedia Systems 22, no. 1 (2016): 1-3.
[52] Tosun, Suleyman, Vahid B. Ajabshir, Ozge Mercanoglu, and Ozcan Ozturk. "Fault-tolerant topology generation method for application-specific network-on-chips." IEEE Transactions on Computer-Aided Design of Integrated Circuits and Systems 34, no. 9 (2015): 1495-1508.
[53] Yang, Chunsheng, Yanni Zou, Pinhua Lai, and Nan Jiang. "Data mining-based methods for fault isolation with validated FMEA model ranking." Applied Intelligence 43, no. 4 (2015): 913-923.
[54] Zhang, Yongshuang, Changbao Guo, Hengxing Lan, Nengjuan Zhou, and Xin Yao. "Reactivation mechanism of ancient giant landslides in the tectonically active zone: a case study in Southwest China." Environmental Earth Sciences 74, no. 2 (2015): 1719-1729.
[55] Zimek, Arthur, and Jilles Vreeken. "The blind men and the elephant: On meeting the problem of multiple truths in data from clustering and pattern mining perspectives." Machine Learning 98, no. 1-2 (2015): 121-155.
[56] Gong, Ke, Panpan Wang, and Yi Peng. "Fault-tolerant enhanced bijective soft set with applications." Applied Soft Computing (2016).
[57] Haseena, Hassan H., Paul K. Joseph, and Abraham T. Mathew. "Classification of arrhythmia using hybrid networks." Journal of medical systems 35, no. 6 (2011): 1617-1630.
[58] Kumar, S. Udhaya, H. Hannah Inbarani, and S. Senthil Kumar. "Improved bijective-soft-set-based classification for gene expression data." In Computational Intelligence, Cyber Security and Computational Models, pp. 127-132. Springer India, 2014.
[59] Senthilkumar, S., H. Hannah Inbarani, and S. Udhayakumar. "Modified soft rough set for multiclass classification." In Computational Intelligence, Cyber Security and Computational Models, pp. 379-384. Springer India, 2014.
[60] Wei, Song, Hani Hagras, and Daniyal Alghazzawi. "A cloud computing based Big-Bang Big-Crunch fuzzy logic multi classifier system for Soccer video scenes classification." Memetic Computing 8, no. 4 (2016): 307-323.
[61] Fernández, Alberto, Sara del Río, Abdullah Bawakid, and Francisco Herrera. "Fuzzy rule based classification systems for big data with MapReduce: granularity analysis." Advances in Data Analysis and Classification (2016): 1-20.
[62] Nelles, Oliver. "Unsupervised Learning Techniques." In Nonlinear System Identification, pp. 137-155. Springer Berlin Heidelberg, 2001.
[63] Bengio, Yoshua, Aaron Courville, and Pascal Vincent. "Representation learning: A review and new perspectives." IEEE transactions on pattern analysis and machine intelligence 35, no. 8 (2013): 1798-1828.
[64] Huang, Fei, and Alexander Yates. "Biased representation learning for domain adaptation." In Proceedings of the 2012 Joint Conference on Empirical Methods in Natural Language Processing and Computational Natural Language Learning, pp. 1313-1323. Association for Computational Linguistics, 2012.
[65] Tu, Wenting, and Shiliang Sun. "Cross-domain representation-learning framework with combination of class-separate and domain-merge objectives." In Proceedings of the 1st International Workshop on Cross Domain Knowledge Discovery in Web and Social Network Mining, pp. 18-25. ACM, 2012.
[66] Li, Shou-Shan, Chu-Ren Huang, and Cheng-Qing Zong. "Multi-domain sentiment classification with classifier combination." Journal of Computer Science and Technology 26, no. 1 (2011): 25-33.
[67] F Huang, E Yates, Exploring representation-learning approaches to domain adaptation, in Proceedings of the 2010 Workshop on Domain Adaptation for Natural Language Processing (Uppsala, 2010), pp. 23–30
[68] A Bordes, X Glorot, JWAY Bengio, Joint learning of words and meaning representations for open-text semantic parsing, in Proceedings of 15th International Conference on Artificial Intelligence and Statistics (La Palma, 2012), pp. 127–135
[69] N. Boulanger-Lewandowski, Y. Bengio, P. Vincent, Modeling temporal dependencies in high-dimensional sequences: application to polyphonic music generation and transcription. arXiv preprint (2012). arXiv:1206.6392
[70] K Dwivedi, K Biswaranjan, A Sethi, Drowsy driver detection using representation learning, in Proceedings of the IEEE International Advance Computing Conference (Gurgaon, 2014), pp. 995–999
[71] D Yu, L Deng, Deep learning and its applications to signal and information processing. IEEE Signal Proc Mag 28(1), 145–154 (2011)
[72] I Arel, DC Rose, TP Karnowski, Deep machine learning-a new frontier in artificial intelligence research. IEEE Comput Intell Mag 5(4), 13–18 (2010)
[73] Y Bengio, Learning deep architectures for AI. Foundations Trends Mach Learn 2(1), 1–127 (2009)
[74] R Collobert, J Weston, L Bottou, M Karlen, K Kavukcuoglu, P Kuksa, Natural language processing (almost) from scratch. J Mach Learn Res 12, 2493–2537 (2011)
[75] P Le Callet, C Viard-Gaudin, D Barba, A convolutional neural network approach for objective video quality assessment. IEEE Trans Neural Networ 17(5), 1316–1327 (2006)
[76] GE Dahl, D Yu, L Deng, A Acero, Context-dependent pre-trained deep neural networks for large-vocabulary speech recognition. IEEE Trans Audio Speech Lang Proc 20(1), 30–42 (2012)
[77] G Hinton, L Deng, Y Dong, GE Dahl, A Mohamed, N Jaitly, A Senior, V Vanhoucke, P Nguyen, TN Sainath, B Kingsbury, Deep neural networks for acoustic modeling in speech recognition: the shared views of four research groups. IEEE Signal Proc Mag 29(6), 82–97 (2012)
[78] DC Ciresan, U Meier, LM Gambardella, J Schmidhuber, Deep, big, simple neural nets for handwritten digit recognition. Neural Comput 22(12), 3207–3220(2010)
[79] D Peteiro-Barral, B Guijarro-Berdiñas, A survey of methods for distributed machine learning. Progress in Artificial Intelligence 2(1), 1–11 (2012)
[80] H Zheng, SR Kulkarni, HV Poor, Attribute-distributed learning: models, limits,and algorithms. IEEE Trans Signal Process 59(1), 386–398 (2011)
[81] H Chen, T Li, C Luo, SJ Horng, G Wang, A rough set-based method for updating decision rules on attribute values’ coarsening and refining. IEEE Trans Knowl Data Eng 26(12), 2886–2899 (2014)
[82] J Chen, C Wang, R Wang, Using stacked generalization to combine SVMs in magnitude and shape feature spaces for classification of hyperspectral data. IEEE Trans Geosci Remote 47(7), 2193–2205 (2009)
[83] E Leyva, A González, R Pérez, A set of complexity measures designed for applying meta-learning to instance selection. IEEE Trans Knowl Data Eng 27(2), 354–367 (2014)
[84] M Sarnovsky, M Vronc, Distributed boosting algorithm for classification of text documents, in Proceedings of the 12th IEEE International Symposium on Applied Machine Intelligence and Informatics (SAMI) (Herl`any, 2014), pp. 217–220
[85] SR Upadhyaya, Parallel approaches to machine learning—a comprehensive survey. J Parallel Distr Com 73(3), 284–292 (2013)R Bekkerman, M Bilenko, J Langford, Scaling up machine learning: parallel and distributed approaches (Cambridge University Press, Oxford, 2011)
[86] EW Xiang, B Cao, DH Hu, Q Yang, Bridging domains using world wide knowledge for transfer learning. IEEE Trans Knowl Data Eng 22(6), 770–783 (2010)
[87] SJ Pan, Q Yang, A survey on transfer learning. IEEE Trans Knowl Data Eng 22(10), 1345–1359 (2010)
[88] W Fan, I Davidson, B Zadrozny, PS Yu, An improved categorization of classifier’s sensitivity on sample selection bias, in Proceedings of the 5th IEEE International Conference on Data Mining (ICDM) (Brussels, 2012), pp. 605–608
[89] J Gao, W Fan, J Jiang, J Han, Knowledge transfer via multiple model local structure mapping, in Proceedings of the 14th ACM SIGKDD International Conference on Knowledge Discovery and Data Mining (Las Vegas, 2008), pp. 283-291
[90] C Wang, S Mahadevan, Manifold alignment using procrustes analysis, in Proceedings of the 25th International Conference on Machine Learning (ICML) (Helsinki, 2008), pp. 1120–1127
[91] X Ling, W Dai, GR Xue, Q Yang, Y Yu, Spectral domain-transfer learning, in Proceedings of the 14th ACM SIGKDD International Conference on Knowledge Discovery and Data Mining (Las Vegas, 2008), pp. 488–496
[92] R Raina, AY Ng, D Koller, 2006, Constructing informative priors using transfer learning, in Proceedings of the 23rd International Conference on Machine Learning (ICML) (Pittsburgh, 2006), pp. 713–720
[93] J Zhang, Deep transfer learning via restricted Boltzmann machine for document classification, in Proceedings of the 10th International Conference on Machine Learning and Applications and Workshops (ICMLA) (Honolulu,2011), pp. 323–326
[94] Y Fu, B Li, X Zhu, C Zhang, Active learning without knowing individual instance labels: a pairwise label homogeneity query approach. IEEE Trans Knowl Data Eng 26(4), 808–822 (2014)
[95] B Settles, Active learning literature survey (University of Wisconsin, Madison, 2010) MM Crawford, D Tuia, HL Yang, Active learning: any value for classification of remotely sensed data? P IEEE 101(3), 593–608 (2013)
[96] MM Haque, LB Holder, MK Skinner, DJ Cook, Generalized query-based active learning to identify differentially methylated regions in DNA. IEEE ACM Trans Comput Bi 10(3), 632–644 (2013)
[97] D Tuia, M Volpi, L Copa, M Kanevski, J Munoz-Mari, A survey of active learning algorithms for supervised remote sensing image classification. IEEE J Sel Top Sign Proces 5(3), 606–617 (2011)
[98] G Ding, Q Wu, YD Yao, J Wang, Y Chen, Kernel-based learning for statistical signal processing in cognitive radio networks. IEEE Signal Proc Mag 30(4), 126–136 (2013)
[99] C Li, M Georgiopoulos, GC Anagnostopoulos, A unifying framework for typical multitask multiple kernel learning problems. IEEE Trans Neur Net Lear Syst 25(7), 1287–1297 (2014)
[100] G Montavon, M Braun, T Krueger, KR Muller, Analyzing local structure in kernel-based learning: explanation, complexity, and reliability assessment. IEEE Signal Proc Mag 30(4), 62–74 (2013)
[101] K Slavakis, S Theodoridis, I Yamada, Online kernel-based classification using adaptive projection algorithms. IEEE Trans Signal Process 56(7), 2781–2796 (2008)
[102] S Theodoridis, K Slavakis, I Yamada, Adaptive learning in a world of projections. IEEE Signal Proc Mag 28(1), 97–123 (2011)
[103] K Slavakis, S Theodoridis, I Yamada, Adaptive constrained learning in reproducing kernel Hilbert spaces: the robust beamforming case. IEEE Trans Signal Process 57(12), 4744–4764 (2009)
[104] K Slavakis, P Bouboulis, S Theodoridis, Adaptive multiregression in reproducing kernel Hilbert spaces: the multiaccess MIMO channel case. IEEE Trans Neural Netw Learn Syst 23(2), 260–276 (2012)
[105] KR Müller, S Mika, G Rätsch, K Tsuda, B Schölkopf, An introduction to kernel based learning algorithms. IEEE Trans Neural Networ 12(2), 181–201 (2001)
[106] Kocaguneli, Ekrem, Tim Menzies, and Emilia Mendes. "Transfer learning in effort estimation." Empirical Software Engineering 20, no. 3 (2015): 813-843.
[107] Bengio, Yoshua, Aaron Courville, and Pascal Vincent. "Representation learning: A review and new perspectives." IEEE transactions on pattern analysis and machine intelligence 35, no. 8 (2013): 1798-1828.
[108] Van Hulse J, Khoshgoftaar T. Knowledge discovery from imbalanced and noisy data. Data Knowl Eng. 2009;68(12):1513–42.
[109] Khoshgoftaar TM, Hulse JV. Imputation techniques for multivariate missingness in software measurement data.Software Quality J. 16(4):563–600; 2008.
[110] Khoshgoftaar TM, Van Hulse J, Napolitano A. Comparing boosting and bagging techniques with noisy and imbalanced data. Syst Man Cybern Part A Syst Hum IEEE Trans. 2011;41(3):552–68.
[111] Van Hulse J, Khoshgoftaar TM, Napolitano A. Experimental perspectives on learning from imbalanced data. In:Proceedings of the 24th International Conference on Machine Learning; 2007. pp. 935–42.
[112] Hogan JM, Peut T. Large Scale Read Classification for Next Generation Sequencing. Procedia Comput Sci.2014;29:2003–12.
[113] Sun K, Miao W, Zhang X, Rao R. An Improvement to Feature Selection of Random Forests on Spark. In: 2014 IEEE 17th International Conference on Computational Science and Engineering (CSE); 2014. pp. 774–9.
[114] Ooi, Beng Chin, Kian-Lee Tan, Sheng Wang, Wei Wang, Qingchao Cai, Gang Chen, Jinyang Gao et al. "SINGA: A distributed deep learning platform." In Proceedings of the 23rd ACM international conference on Multimedia, pp. 685-688. ACM, 2015.
[115] Y. Jia, E. Shelhamer, J. Donahue, S. Karayev, J. Long, R. Girshick, S. Guadarrama, and T. Darrell.Ca_e: Convolutional architecture for fast feature embedding. arXiv preprint arXiv:1408.5093, 2014.
[116] M. Lin, S. Li, X. Luo, and S. Yan. Purine: A bi-graph based deep learning framework. CoRR, abs/1412.6249,2014.
[117] N. Vasilache, J. Johnson, M. Mathieu, S. Chintala, S. Piantino, and Y. LeCun. Fast convolutional nets with fb_t: A GPU performance evaluation. CoRR, abs/1412.7580, 2014.
[118] Zaharia, Matei, Mosharaf Chowdhury, Michael J. Franklin, Scott Shenker, and Ion Stoica. "Spark: Cluster Computing with Working Sets." HotCloud 10, no. 10-10 (2010): 95.
[119] T. White, Hadoop: The Definitive Guide, O’Reilly Media, 2009.
[120] Caudill, Steven B., and Franklin G. Mixon. "Analysing misleading discrete responses: A logit model based on misclassified data." Oxford Bulletin of Economics and Statistics 67, no. 1 (2005): 105-113.
[121] Brodley, Carla E., and Mark A. Friedl. "Identifying mislabeled training data." Journal of Artificial Intelligence Research 11 (1999): 131-167.
[122] Miranda, André LB, Luís Paulo F. Garcia, André CPLF Carvalho, and Ana C. Lorena. "Use of classification algorithms in noise detection and elimination." In International Conference on Hybrid Artificial Intelligence Systems, pp. 417-424. Springer Berlin Heidelberg, 2009.
[123] Van den Hout, Ardo, and Peter GM Van der Heijden. "The analysis of multivariate misclassified data with special attention to randomized response data." Sociological Methods & Research 32, no. 3 (2004): 384-410.
[124] Bilgic, Mustafa, and Lise Getoor. "Reflect and correct: A misclassification prediction approach to active inference." ACM Transactions on Knowledge Discovery from Data (TKDD) 3, no. 4 (2009): 20.
[125] Ciraco, Michelle, Michael Rogalewski, and Gary Weiss. "Improving classifier utility by altering the misclassification cost ratio." In Proceedings of the 1st international workshop on Utility-based data mining, pp. 46-52. ACM, 2005.
[126] Healy, J. D. "The effects of misclassification error on the estimation of several population proportions." Bell System Technical Journal 60, no. 5 (1981): 697-705.
[127] Smith, Michael R., Tony Martinez, and Christophe Giraud-Carrier. "An instance level analysis of data complexity." Machine learning 95, no. 2 (2014): 225-256.
[128] Evans, Robert. "Apache storm, a hands on tutorial." In Cloud Engineering (IC2E), 2015 IEEE International Conference on, pp. 2-2. IEEE, 2015.
[129] Olston, Christopher, Benjamin Reed, Utkarsh Srivastava, Ravi Kumar, and Andrew Tomkins. "Pig latin: a not-so-foreign language for data processing." In Proceedings of the 2008 ACM SIGMOD international conference on Management of data, pp. 1099-1110. ACM, 2008.
[130] Presutti, Valentina, Francesco Draicchio, and Aldo Gangemi. "Knowledge extraction based on discourse representation theory and linguistic frames." In International Conference on Knowledge Engineering and Knowledge Management, pp. 114-129. Springer Berlin Heidelberg, 2012.
[131] Brown, Samuel DJ, Rupert A. Collins, Stephane Boyer, MARIE‐CAROLINE LEFORT, J. A. G. O. B. A. MALUMBRES‐OLARTE, Cor J. Vink, and Robert H. Cruickshank. "Spider: an R package for the analysis of species identity and evolution, with particular reference to DNA barcoding." Molecular Ecology Resources 12, no. 3 (2012): 562-565.
[132] Mika, Sebastian, Bernhard Schölkopf, Alexander J. Smola, Klaus-Robert Müller, Matthias Scholz, and Gunnar Rätsch. "Kernel PCA and De-noising in feature spaces." In NIPS, vol. 11, pp. 536-542. 1998.
[133] Zucker, David M., and Donna Spiegelman. "Corrected score estimation in the proportional hazards model with misclassified discrete covariates." Statistics in medicine 27, no. 11 (2008): 1911-1933.
[134] Yuan, Yang C. "Multiple imputation for missing data: Concepts and new development (Version 9.0)." SAS Institute Inc, Rockville, MD 49 (2010): 1-11.
[135] Zucker, David M., and Donna Spiegelman. "Corrected score estimation in the proportional hazards model with misclassified discrete covariates." Statistics in medicine 27, no. 11 (2008): 1911-1933.Akazawa K, Kinukawa N, Nakamura T. A note on the corrected score function corrected formisclassification. Journal of the Japan Statistical Society. 1998; 28:115–123.
[136] Nakamura T. Corrected score function of errors-in-variables models: methodology and application to generalized linear models. Biometrika. 1990; 77:127–137.
[137] Spiegelman, Donna, Aidan McDermott, and Bernard Rosner. "Regression calibration method for correcting measurement-error bias in nutritional epidemiology." The American journal of clinical nutrition 65, no. 4 (1997): 1179S-1186S.
[138] Gaurav Jain, Kunal Gupta, Arpit Kushwah, Abhishek Agrawal, "A Survey on Various Issues Big Data in Cloud Computing", International Journal of Computer Sciences and Engineering, Vol.5, Issue.9, pp.131-134, 2017.