Future Opportunities and Challenges in Sentiment Analysis: An Overview
Pranay Kumar B.V1 , M. Sadanandam2
- Dept of CSE, Christu Jyothi Institute of Technology and Science, Hyderabad, India.
- Dept of CSE, UCE Kakatiya University, Warangal, India.
Correspondence should be addressed to: pranaybv4u@gmail.com.
Section:Review Paper, Product Type: Journal Paper
Volume-5 ,
Issue-12 , Page no. 279-286, Dec-2017
CrossRef-DOI: https://doi.org/10.26438/ijcse/v5i12.279286
Online published on Dec 31, 2017
Copyright © Pranay Kumar B.V, M. Sadanandam . This is an open access article distributed under the Creative Commons Attribution License, which permits unrestricted use, distribution, and reproduction in any medium, provided the original work is properly cited.
View this paper at Google Scholar | DPI Digital Library
How to Cite this Paper
- IEEE Citation
- MLA Citation
- APA Citation
- BibTex Citation
- RIS Citation
IEEE Style Citation: Pranay Kumar B.V, M. Sadanandam, “Future Opportunities and Challenges in Sentiment Analysis: An Overview,” International Journal of Computer Sciences and Engineering, Vol.5, Issue.12, pp.279-286, 2017.
MLA Style Citation: Pranay Kumar B.V, M. Sadanandam "Future Opportunities and Challenges in Sentiment Analysis: An Overview." International Journal of Computer Sciences and Engineering 5.12 (2017): 279-286.
APA Style Citation: Pranay Kumar B.V, M. Sadanandam, (2017). Future Opportunities and Challenges in Sentiment Analysis: An Overview. International Journal of Computer Sciences and Engineering, 5(12), 279-286.
BibTex Style Citation:
@article{B.V_2017,
author = {Pranay Kumar B.V, M. Sadanandam},
title = {Future Opportunities and Challenges in Sentiment Analysis: An Overview},
journal = {International Journal of Computer Sciences and Engineering},
issue_date = {12 2017},
volume = {5},
Issue = {12},
month = {12},
year = {2017},
issn = {2347-2693},
pages = {279-286},
url = {https://www.ijcseonline.org/full_paper_view.php?paper_id=1616},
doi = {https://doi.org/10.26438/ijcse/v5i12.279286}
publisher = {IJCSE, Indore, INDIA},
}
RIS Style Citation:
TY - JOUR
DO = {https://doi.org/10.26438/ijcse/v5i12.279286}
UR - https://www.ijcseonline.org/full_paper_view.php?paper_id=1616
TI - Future Opportunities and Challenges in Sentiment Analysis: An Overview
T2 - International Journal of Computer Sciences and Engineering
AU - Pranay Kumar B.V, M. Sadanandam
PY - 2017
DA - 2017/12/31
PB - IJCSE, Indore, INDIA
SP - 279-286
IS - 12
VL - 5
SN - 2347-2693
ER -
![]() |
![]() |
![]() |
553 | 338 downloads | 221 downloads |
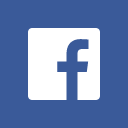
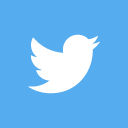
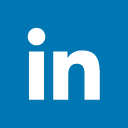
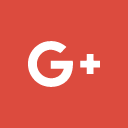
Abstract
Today the evolution of technology and fair access to the internet in countries such as India, public opinions over social media, expression of sentiment on products and services are fast and furious in present days. These opinions have value for companies to materialize profits and understand the market for their future strategic decisions. Present technology adoption energized by the healthy growth in big data framework, caused applications based on Sentiment Analysis (SA) in big data to become common for businesses. But there is wide gap and scope for SA application in big data. This paper discusses various Sentiment analysis approaches and algorithms, including sentiment polarity detection, SA features (explicit and implicit), sentiment classification techniques, applications of SA. Future opportunities and challenges of sentiment analysis is explored. Scalable, automated, accurate, sophisticated sentiment analysis is a much sought-after technology that almost no one has truly nailed yet.
Key-Words / Index Term
Sentiment Analysis Approaches, tools, techniques, machine Learning, opportunities, and challenges, supervised learning and unsupervised learning
References
[1]. Harnani Mat Zin and Samaneh Nadali, Nurfadhlina Mohd Sharef. “Overview and Future Opportunities of Sentiment Analysis Approaches for Big Data.” thescipub.com. http://thescipub.com/PDF/jcssp.2016.153.168.pdf
[2]. .Agnihotri, R., R. Dingus, M.Y. Hu and M.T. Krush, 2015. Social media: Influencing customer satisfaction in B2B sales. Industrial Market. Manage. DOI: 10.1016/j.indmarman.2015.09.003
[3]. Asur, S. and B.A. Huberman, 2010. Predicting the future with social media. Proceedings of the International Conference on Web Intelligence and Intelligent Agent Technology, Aug. 31 2010-Sept. 3, IEEE Xplore Press, Toronto, ON, pp: 492-499. DOI: 10.1109/WI-IAT.2010.63
[4]. Bagheri, A., M. Saraee and F. de Jong, 2013. Care more about customers: Unsupervised domain independent aspect detection for sentiment analysis of customer reviews. Knowledge-Based Syst., 52: 201-213. DOI: 10.1016/j.knosys.2013.08.011
[5]. Balahur, A., J.M. Hermida and A. Montoya, 2012. Detecting implicit expressions of emotion in a text: A comparative analysis. Decision Support Syst., 53: 742-753. DOI: 10.1016/j.dss.2012.05.024
[6]. Balahur, A., R. Mihalcea and A. Montoya, 2014. Computational approaches to subjectivity and sentiment analysis: Present and envisaged methods and applications. Comput. Speech Lang., 28: 1-6. DOI: 10.1016/j.csl.2013.09.003
[7]. Banea, C., R. Mihalcea and J. Wiebe, 2014. Sense-level subjectivity in a multilingual setting. Comput. Speech Lang., 28: 7-19. DOI: 10.1016/j.csl.2013.03.002
[8]. Batrinca, B. and P.C. Treleaven, 2014. Social media analytics: A survey of techniques, tools and platforms. Ai Society, 30: 89-116. DOI: 10.1007/s00146-014-0549-4
[9]. Bernabé-Moreno, J., A. Tejeda-Lorente, C. Porcel, H. Fujita and E. Herrera-Viedma, 2015. CARESOME: A system to enrich marketing customers acquisition and retention campaigns using social media information. Knowledge-Based Syst., 80: 163-179. DOI: 10.1016/j.knosys.2014.12.033
[10]. Bing, L.I. and K.C.C. Chan, 2014. A fuzzy logic approach for opinion mining on large-scale twitter data. Proceedings of the ACM 7th International Conference on Utility and Cloud Computing, 0Dec. 8-11, IEEE Xplore Press, London, pp: 652-657. DOI: 10.1109/UCC.2014.105
[11]. Bravo-Marquez, F., M. Mendoza and B. Poblete, 2014. Meta-level sentiment models for big social data analysis. Knowledge-Based Syst., 69: 86-99. DOI: 10.1016/j.knosys.2014.05.016
[12]. Cambria, E., M. Grassi, A. Hussain and C. Havasi, 2011. Sentic Computing for social media marketing. Multimedia Tools Applic., 59: 557-577. DOI: 10.1007/s11042-011-0815-0
[13]. Chaumartin, F., L. Talana and U. Paris, 2007. UPAR7: A knowledge-based system for headline sentiment tagging. Proceedings of the 4th International Workshop on Semantic Evaluations, (WSE’ 07), Stroudsburg, PA, USA, pp: 422-425. DOI: 10.3115/1621474.1621568
[14]. Cheong, M. and V.C.S. Lee, 2010. A microblogging based approach to terrorism informatics: Exploration and chronicling civilian sentiment and response to terrorism events via Twitter. Inform. Syst. Frontiers, 13: 45-59. DOI: 10.1007/s10796-010-9273-x
[15]. Conejero, J., P. Burnap, O. Rana and J. Morgan, 2013. Scaling archived social media data analysis using a Hadoop cloud. Proceedings of the IEEE 6th International Conference on Cloud Computing, Jun. 28 Jul. 3, IEEE Xplore Press, Santa Clara, CA, pp: 685-692. DOI: 10.1109/CLOUD.2013.120
[16]. Da Silva, N.F.F., E.R. Hruschka and E.R. Hruschka, 2014. Tweet sentiment analysis with classifier ensembles. Decision Support Syst., 66: 170-179. DOI: 10.1016/j.dss.2014.07.003 De Vel, O., A. Anderson, M. Corney and G. Mohay, 2001. Mining e-mail content for author identification forensics. ACM SIGMOD Record, 30: 55-64. DOI: 10.1145/604264.604272
[17]. Denecke, K., 2008. Using SentiWordNet for multilingual sentiment analysis. Proceedings of the 24th International Conference on Data Engineering Workshop, Apr. 7-12, IEEE Xplore Press, Cancun, pp: 507-512. DOI: 10.1109/ICDEW.2008.4498370
[18]. Derczynski, L. and K. Bontcheva, 2014a. PHEME: Veracity in digital social networks. Proceedings of the Workshop on UMAP Projects Synergy, (UPS’ 14), pp: 1-4.
[19]. Ding, X., B. Liu and S.M. Street, 2007. The utility of linguistic rules in opinion mining. Proceedings of the 30th Annual International ACM SIGIR Conference on Research and Development in Information Retrieval, Jul. 23-27, Amsterdam, pp: 811-812. DOI: 10.1145/1277741.1277921
[20]. Efron, M., M. Gary and Z. Julinang, 2004. Cultural orientation: Classifying subjective documents by Cociation analysis. Proceedings of the AAAI Fall Symposium Series on Style and Meaning in Language, Art, Music and Design, (AMD’ 04), pp: 41-48.
[21]. Eirinaki, M., S. Pisal and J. Singh, 2012. Feature-based opinion mining and ranking. J. Comput. Syst. Sci., 78: 1175-1184. DOI: 10.1016/j.jcss.2011.10.007
[22]. Fei, X., H. Wang and J. Zhu, 2010. Sentiment word identification using the maximum entropy model. Proceedings of the 6th International Conference on Natural Language Processing and Knowledge Engineering, Aug. 21-23, IEEE Xplore Press, Beijing, pp: 1-4. DOI: 10.1109/NLPKE.2010.5587811
[23]. Fei, Z., J. Liu and G. Wu, 2004. Sentiment classification using phrase patterns. Proceedings of the 4th IEEE International Conference on Computer Information Technology, Sept. 14-16, IEEE Xplore Press, pp: 1147-1152. DOI: 10.1109/CIT.2004.1357349
[24]. Fulse, S., R. Sugandhi and A. Mahajan, 2014. A survey on multimodal sentiment analysis. Int. J. Eng. Res. Technol., 3: 1233-1238. Gamon, M., 2004.
[25]. Sentiment classification on customer feedback data: Noisy data, large feature vectors and the role of linguistic analysis. Proceedings of the 20th International Conference on Computational Linguistics, (CCL’ 04), Stroudsburg, PA, USA, pp: 1-7. DOI: 10.3115/1220355.1220476
[26]. Ghazi, D., D. Inkpen and S. Szpakowicz, 2014. The prior and contextual emotion of words in sentential context. Comput. Speech Lang., 28: 76-92. DOI: 10.1016/j.csl.2013.04.009
[27]. Go, A., R. Bhayani and L. Huang, 2009. Twitter sentiment classification using distant supervision. Grefenstette, G., Y. Qu, J. Shanahan and D. Evans, 2004.
[28]. Guo, Y., Z. Shao and N. Hua, 2010. Automatic text categorization based on content analysis of cognitive situation models. Inform. Sci., 180: 613-630. DOI: 10.1016/j.ins.2009.11.012
[29]. Habernal, I., T. Ptáček and J. Steinberger, 2014. Supervised sentiment analysis in Czech social media. Inform. Process. Manage., 50: 693-707. DOI: 10.1016/j.ipm.2014.05.001
[30]. Hai, Z., K. Chang and J. Kim, 2011. Implicit feature identification via co-occurrence association rule mining. Proceedings of the 12th International Conference on Computational Linguistics and Intelligent Text Processing, Feb. 20-26, Springer Berlin Heidelberg, pp: 393-404. DOI: 10.1007/978-3-642-19400-9_31
[31]. Harrigan, P., G. Soutar, M.M. Choudhury and M. Lowe, 2014. Modelling CRM in a social media age. Aus. Market. J., 23: 27-37. DOI: 10.1016/j.ausmj.2014.11.001
[32]. Hatzivassiloglou, V. and K.R. McKeown, 1997. Predicting the semantic orientation of adjectives. Proceedings of the 8th Conference on European chapter of the Association for Computational Linguistics, (ACL’ 97), Stroudsburg, PA, USA, pp: 174-181. DOI: 10.3115/976909.979640
[33]. He, W., H. Wu, G. Yan, V. Akula and J. Shen, 2015. A novel social media competitive analytics framework with sentiment benchmarks. Inform. Manage., 52: 801-812. DOI: 10.1016/j.im.2015.04.006