Clustering Mutual Outline for Multi Assessment Temporal Data and cancer Data
Sathishkumar. K1 , V. Thiagarasu2 , E. Balamurugan3 , David Otto Arthur4
- Dept. of CS, Gobi Arts and Science College (Autonomus), Gobi, India.
- Dept. of CS, Gobi Arts and Science College (Autonomus), Gobi, India.
- Bluecrest College, Accra, Ghana, West Africa.
- Bluecrest College, Accra, Ghana, West Africa.
Correspondence should be addressed to: sathishmsc.vlp@gmail.com.
Section:Research Paper, Product Type: Journal Paper
Volume-6 ,
Issue-1 , Page no. 71-79, Jan-2018
CrossRef-DOI: https://doi.org/10.26438/ijcse/v6i1.7179
Online published on Jan 31, 2018
Copyright © Sathishkumar. K, V. Thiagarasu, E. Balamurugan, David Otto Arthur . This is an open access article distributed under the Creative Commons Attribution License, which permits unrestricted use, distribution, and reproduction in any medium, provided the original work is properly cited.
View this paper at Google Scholar | DPI Digital Library
How to Cite this Paper
- IEEE Citation
- MLA Citation
- APA Citation
- BibTex Citation
- RIS Citation
IEEE Style Citation: Sathishkumar. K, V. Thiagarasu, E. Balamurugan, David Otto Arthur , “Clustering Mutual Outline for Multi Assessment Temporal Data and cancer Data,” International Journal of Computer Sciences and Engineering, Vol.6, Issue.1, pp.71-79, 2018.
MLA Style Citation: Sathishkumar. K, V. Thiagarasu, E. Balamurugan, David Otto Arthur "Clustering Mutual Outline for Multi Assessment Temporal Data and cancer Data." International Journal of Computer Sciences and Engineering 6.1 (2018): 71-79.
APA Style Citation: Sathishkumar. K, V. Thiagarasu, E. Balamurugan, David Otto Arthur , (2018). Clustering Mutual Outline for Multi Assessment Temporal Data and cancer Data. International Journal of Computer Sciences and Engineering, 6(1), 71-79.
BibTex Style Citation:
@article{K_2018,
author = {Sathishkumar. K, V. Thiagarasu, E. Balamurugan, David Otto Arthur },
title = {Clustering Mutual Outline for Multi Assessment Temporal Data and cancer Data},
journal = {International Journal of Computer Sciences and Engineering},
issue_date = {1 2018},
volume = {6},
Issue = {1},
month = {1},
year = {2018},
issn = {2347-2693},
pages = {71-79},
url = {https://www.ijcseonline.org/full_paper_view.php?paper_id=1635},
doi = {https://doi.org/10.26438/ijcse/v6i1.7179}
publisher = {IJCSE, Indore, INDIA},
}
RIS Style Citation:
TY - JOUR
DO = {https://doi.org/10.26438/ijcse/v6i1.7179}
UR - https://www.ijcseonline.org/full_paper_view.php?paper_id=1635
TI - Clustering Mutual Outline for Multi Assessment Temporal Data and cancer Data
T2 - International Journal of Computer Sciences and Engineering
AU - Sathishkumar. K, V. Thiagarasu, E. Balamurugan, David Otto Arthur
PY - 2018
DA - 2018/01/31
PB - IJCSE, Indore, INDIA
SP - 71-79
IS - 1
VL - 6
SN - 2347-2693
ER -
![]() |
![]() |
![]() |
757 | 490 downloads | 281 downloads |
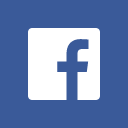
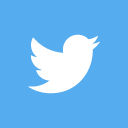
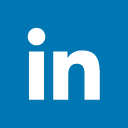
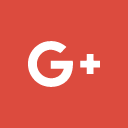
Abstract
Clustering temporal data compiled from cancer registries is a crucial problem faced by many data analyst owing to the elevated high dimensionality, weight value calculation, multi view data and multifaceted temporal correlation. This research work reveals a hypothetical effect of Temporal Clustering (TC) in various domains on cancer genome risk estimates by introducing data mining clustering algorithms. For the first time, cancer genome datasets samples were made available for the complete genome sequences consisting of point mutations and structural alternations for a huge number of cancer types which allows the variation of cancer subtypes in an exceptional excellent global analysis. In this work, TC algorithm is presented to the allocation of several time- series into a set of non-overlapping parts that fit in to k temporal clusters. The paper presents a group of clustering communal framework for multi view data, TW-K-means and an automated two-level variable clustering algorithm that can be used to calculate the weights for views and person variables. A new ATBCWCE structure is projected to improve the risk estimates in cancer genome.
Key-Words / Index Term
Temporal data clustering, weighted consensus function, multi view learning, k-means
References
[1]. Arthur D., S. Vassilvitskii , K-means++: the advantages of careful seeding, in: Proceedings of the Eighteenth Annual ACM-SIAM Symposium on Discrete Algorithms, SODA ’07, Society for Industrial and Applied Mathematics, Philadelphia, PA, USA, 2007, pp. 1027–1035 .
[2]. Cuturi M., Fast global alignment kernels, in: Proceedings of the 28th International Conference on Machine Learning (ICML-11), 2011, pp. 929–936 .
[3]. Cuturi M., J.-P. Vert , Ø. Birkenes , T. Matsui , A kernel for time series based on global alignments, in: Proceedings of the International Conference on Acoustics, Speech and Signal Processing, 11, 2007, pp. 413–416.
[4]. Petitjean F., A. Ketterlin , P. Gançarski , A global averaging method for dynamic time warping, with applications to clustering, Pattern Recognit. 44 (3) (2011) 678–693 .
[5]. Dhillon I.S., Y. Guan , B. Kulis , Kernel k-means: spectral clustering and normalized cuts, in: Proceedings of the Tenth ACM SIGKDD International Conference on Knowledge Discovery and Data Mining, ACM, 2004, pp. 551–556 .
[6]. Liao W., Clustering of time series data – a survey., Pattern Recognit. 38 (2005) 1857–1874 .
[7]. Frambourg C., A. Douzal-Chouakria , E. Gaussier , Learning multiple temporal matching for time series classification, in: A. Tucker, F. Höppner, A. Siebes, S. Swift (Eds.), Intelligent Data Analysis, Springer Berlin Heidelberg, London, 2013, pp. 198–209 .
[8]. Liao T.W., Mining of vector time series by clustering, Working paper, 2005.
[9]. Dacheng N.; F. Yan; Z. Junlin; F. Yuke; X. Hu; , "Time series analysis based on enhanced NLCS," Information Sciences and Interaction Sciences (ICIS), 2010 3rd International Conference on, vol., no., pp.292-295, 23-25 June 2010.
[10]. Wu X.; D. Huang;, "Data stream clustering for stock data analysis," Industrial and Information Systems (IIS), 2010 2nd International Conference on , vol.2, no., pp.168-171, 10-11 July 2010.
[11]. Huang X., H.-l. LI,” Research on Predicting Agricultural Drought Based on Fuzzy Set and RlS Analysis Model”, 2010 3rd International Conference on Advanced Computer Theory and Engineering (ICACTE),pp 186-189.
[12]. Lin Y.; Y. Yang;, "Stock markets forecasting based on fuzzy time series model," Intelligent Computing and Intelligent Systems, 2009. ICIS 2009. IEEE International Conference on, vol.1, no., pp.782-786, 20-22 Nov. 2009.
[13]. Gao, S., He, Y., & Chen, H. (2009). Wind speed forecast for wind farms based on ARMA-ARCH model. International Conference on Sustainable Power Generation and Supply, 2009. SUPERGEN`09, pp. 1-4.
[14]. Jixue D.; , "Data Mining of Time Series Based on Wave Cluster," Information Technology and Applications, 2009. IFITA `09. International Forum on , vol.1, no., pp.697-699, 15-17 May 2009
[15]. Powell N.; S.Y. Foo; M. Weatherspoon; "Supervised and Unsupervised Methods for Stock Trend Forecasting," System Theory, 2008. SSST 2008. 40th Southeastern Symposium on , vol., no., pp.203-205, 16-18 March 2008.
[16]. Wu J. X., J. L. Wei, “Combining ICA with SVR for prediction of finance time series”, Proceedings of the IEEE International Conference on Automation and Logistics August 18 - 21, 2007, Jinan, China, pp 95-100.
[17]. Verdoolaege G. and Y. Rosseel,” Activation Detection In Event-Related FMRI Through Clustering Of wavelet Distributions”, Proceedings of 2010 IEEE 17th International Conference on Image Processing, September 26-29, 2010, Hong Kong, pp 4393-4395.
[18]. Fern X. and C. Brodley, “Solving Cluster Ensemble Problem by Bipartite Graph Partitioning,” Proc. Int’l Conf. Machine Learning, pp. 36-43, 2004
[19]. Gionis A., H. Mannila, and P. Tsaparas, “Clustering Aggregation,” ACM Trans. Knowledge Discovery from Data, vol. 1, no. 1, article no. 4, Mar. 2007.
[20]. Singh V., L. Mukerjee, J. Peng, and J. Xu, “Ensemble Clustering Using Semidefinite Programming,” Advances in Neural Information Processing Systems, pp. 1353-1360, 2007.
[21]. Topchy A., M. Law, A. Jain, and A. Fred, “Analysis of Consensus Partition in Cluster Ensemble,” Proc. IEEE Int’l Conf. Data Mining, pp. 225-232, 2004.
[22]. Chaudhuri K., S. Kakade, K. Livescu, and K. Sridharan, “Multiview Clustering via Canonical Correlation Analysis,” Proc. 26th Ann. Int’l Conf. Machine Learning, pp. 129-136, 2009
[23]. Tsai C.-Y. and C.-C. Chiu, “Developing a Feature Weight Self-Adjustment Mechanism for a k-Means Clustering Algorithm,” Computational Statistics and Data Analysis, vol. 52, no. 10, pp. 4658- 4672, 2008.
[24]. Deng Z., K. Choi, F. Chung, and S. Wang, “Enhanced Soft Subspace Clustering Integrating Within-Cluster and Between- Cluster Information,” Pattern Recognition, vol. 43, no. 3, pp. 767- 781, 2010.
[25]. Parvin H, Minaei-Bidgoli B, Parvin S, Alinejad H (2012b) A New Classifier ensemble methodology based on subspace learning. J Exp Theor Artif Intell. doi:10.1080/0952813X.2012.715683
[26]. Parvin H, Minaei-Bidgoli B, Alinejad H (2013) Data weighing 1201 mechanisms for clustering ensembles. Comput Electr Eng. http:// 1202 dx.doi.org/10.1016/j.compeleceng.2013.02.004
[27]. Fred A, Jain AK (2002a) Data clustering using evidence accumulation. In: Proceedings of the 16th international conference on pattern recognition, pp. 276–280
[28]. Tzortzis G. and C. Likas, “Multiple View Clustering Using a Weighted Combination of Exemplar-Based Mixture Models,” IEEE Trans. Neural Networks, vol. 21, no. 12, pp. 1925-1938, Dec. 2010.
[29]. B. Long, P. Yu, and Z. Zhang, “A General Model for Multiple View Unsupervised Learning,” Proc. Eighth SIAM Int’l Conf. Data Mining (SDM ’08), 2008.
[30]. Greene D. and P. Cunningham, “A Matrix Factorization Approach for Integrating Multiple Data Views,” Proc. European Conf. Machine Learning and Knowledge Discovery in Databases, pp. 423-438, 2009.
[31]. Chen, X., Xu, X., Huang, J. Z., & Ye, Y. (2013). TW-k-means: automated two-level variable weighting clustering algorithm for multiview data. IEEE Transactions on Knowledge and Data Engineering, 25(4), 932-944.
[32]. S. Wang and K. Chen, “Ensemble Learning with Active Data Selection for Semi-Supervised Pattern Classification,” Proc. Int’l Joint Conf. Neural Networks, 2007.
[33]. W. Chen and S. Chang, “Motion Trajectory Matching of Video Objects,” Proc. SPIE/IS&T Conf. Storage and Retrieval for Media Database, 2000.
[34]. C. Faloutsos, M. Ranganathan, and Y. Manolopoulos, “Fast Subsequence Matching in Time-Series Databases,” Proc. ACM SIGMOD, pp. 419-429, 1994.
[35]. E. Keogh, Temporal Data Mining Benchmarks, http://www.cs. ucr.edu/~eamonn/time_series_data, 2010.
[36]. H. Kien, A. Hua, and K. Vu, “Constrained Locally Weighted Clustering,” Proc. ACM Int’l Conf. Very Large Data Bases (VLDB), pp. 90-101, 2008
[37]. Yang, Y., & Chen, K. (2011). Temporal data clustering via weighted clustering ensemble with different representations. IEEE Transactions on Knowledge and Data Engineering, 23(2), 307-320.