Study of Recurrent Neural Network Classification of Stress Types in Speech Identification
N.P. Dhole1 , S.N. Kale2
- Department of Electronics and Telecommunication Engineering, PRMIT&R Badnera, Amravati (Maharashtra), India.
- Department of Electronics and Telecommunication Engineering, PRMIT&R Badnera, Amravati (Maharashtra), India.
Section:Research Paper, Product Type: Journal Paper
Volume-6 ,
Issue-4 , Page no. 256-360, Apr-2018
CrossRef-DOI: https://doi.org/10.26438/ijcse/v6i4.256360
Online published on Apr 30, 2018
Copyright © N.P. Dhole, S.N. Kale . This is an open access article distributed under the Creative Commons Attribution License, which permits unrestricted use, distribution, and reproduction in any medium, provided the original work is properly cited.
View this paper at Google Scholar | DPI Digital Library
How to Cite this Paper
- IEEE Citation
- MLA Citation
- APA Citation
- BibTex Citation
- RIS Citation
IEEE Style Citation: N.P. Dhole, S.N. Kale, “Study of Recurrent Neural Network Classification of Stress Types in Speech Identification,” International Journal of Computer Sciences and Engineering, Vol.6, Issue.4, pp.256-360, 2018.
MLA Style Citation: N.P. Dhole, S.N. Kale "Study of Recurrent Neural Network Classification of Stress Types in Speech Identification." International Journal of Computer Sciences and Engineering 6.4 (2018): 256-360.
APA Style Citation: N.P. Dhole, S.N. Kale, (2018). Study of Recurrent Neural Network Classification of Stress Types in Speech Identification. International Journal of Computer Sciences and Engineering, 6(4), 256-360.
BibTex Style Citation:
@article{Dhole_2018,
author = {N.P. Dhole, S.N. Kale},
title = {Study of Recurrent Neural Network Classification of Stress Types in Speech Identification},
journal = {International Journal of Computer Sciences and Engineering},
issue_date = {4 2018},
volume = {6},
Issue = {4},
month = {4},
year = {2018},
issn = {2347-2693},
pages = {256-360},
url = {https://www.ijcseonline.org/full_paper_view.php?paper_id=1900},
doi = {https://doi.org/10.26438/ijcse/v6i4.256360}
publisher = {IJCSE, Indore, INDIA},
}
RIS Style Citation:
TY - JOUR
DO = {https://doi.org/10.26438/ijcse/v6i4.256360}
UR - https://www.ijcseonline.org/full_paper_view.php?paper_id=1900
TI - Study of Recurrent Neural Network Classification of Stress Types in Speech Identification
T2 - International Journal of Computer Sciences and Engineering
AU - N.P. Dhole, S.N. Kale
PY - 2018
DA - 2018/04/30
PB - IJCSE, Indore, INDIA
SP - 256-360
IS - 4
VL - 6
SN - 2347-2693
ER -
![]() |
![]() |
![]() |
415 | 342 downloads | 237 downloads |
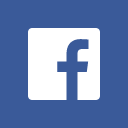
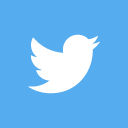
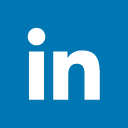
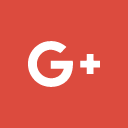
Abstract
Speech of human beings is the reflection of the state of mind. Proper evaluation of these speech signals into stress types is necessary in order to ensure that the person is in a healthy state of mind. More than a decade has passed since research on stress types in speech identification has become a new field of research in line with its ‘big brothers’ speech and speaker recognition. This article attempts to provide a short overview on where we are today, how we got there and what this can reveal us on where to go next and how we could arrive there. In this work we propose a Recurrent Neural Network classifier for speech stress classification algorithm, with sophisticated feature extraction techniques as Mel Frequency Cepstral Coefficients (MFCC). The algorithm assists the system to learn the speech patterns in real time and self-train itself in order to improve the classification accuracy of the overall system. The proposed system is suitable for real time speech and is language and word independent.
Key-Words / Index Term
RNN, MFCC, Stress Classification, Feature Selection
References
[1] Schuller, Bjorn, et al., “Recognising realistic emotions and affect in speech: State of the art and lessons learnt from the first Challenge”, Speech Communication 53.9, pp. 1062-1087, 2011.
[2] Anagnostopoulos, Christos-Nikolaos, Theodoros Iliou, and Ioannis Giannoukos, “Features and Classifiers for Emotion Recognition from Speech: A survey from 2000 to 2011”, Artificial Intelligence Review 43.2, pp.155-177, 2015.
[3] Dipti D. Joshi, M. B. Zalte, “Speech Emotion Recognition: A Review”, Journal of Electronics and Communication Engineering (IOSR-JECE) 4.4, pp.34-37, 2013.
[4] Ververidis, Dimitrios, and Constantine Kotropoulos, “Emotional Speech Recognition: Resources, Features, and Methods”, Speech Communication 48.9, pp.1162-1181, 2006.
[5] El Ayadi, Moataz, Mohamed S. Kamel, and Fakhri Karray, “Survey on Speech Emotion Recognition”, Features, classification schemes, and databases, Pattern Recognition 44.3 pp. 572-587,2011.
[6] Scherer, Klaus R., “Vocal Communication of Emotion: A review of research paradigms”, Speech communication 40.1, pp.227-256, 2003.
[7] Vogt, Thurid, Elisabeth Andre, and Johannes Wagner, “Automatic recognition of emotions from speech: a review of the literature and recommendations for practical realization, Affect and emotion in human-computer interaction”, Springer Berlin Heidelberg, pp. 75-91, 2008.
[8] Burkhardt, Felix, et al., “A Database of German Emotional Speech”, INTER-SPEECH, Lisbon, Portugal, vol. 5, pp.1-4, 2005.
[9] Kwon, Oh-Wook, et al, “Emotion Recognition by Speech Signals, INTER-SPEECH, pp.1-4, 2003.
[10] Campbell, N. “Recording and Storing of Speech Data”. In: Proceedings LREC, pp. 12-25, 2002.
[11] Cowie, R., Douglas-Cowie, E., Savvidou, S., McMahon, E., Sawey, M., Schroder, M. Feeltrace, “An Instrument for Recording Perceived Emotion in Real Time”, In: Proceedings of the ISCA Workshop on Speech and Emotion, pp.19-24, 2000.
[12] Devillers, L., Cowie, R., Martin, J.-C., Douglas-Cowie, E., Abrilian, S., McRorie, M.: “Real life emotions in French and English TV video clips: an integrated annotation protocol combining continuous and discrete approaches”, 5th International Conference on Language Resources and Evaluation LREC, Genoa, Italy.2006.
[13] Douglas-Cowie, E., Campbell, N., Cowie, R.P. “ Emotional speech: Towards a new generation of databases”. Speech Communication 40(1–2), pp.33-60, 2003.
[14] Douglas-Cowie, E., et al.: “The description of naturally occurring emotional speech”. In: Proceedings of 15th International Congress of Phonetic Sciences, Barcelona, 2003.
[15] http://audacity.sourceforge.net/download.
[16] A. J. Robinson, "An Application of Recurrent Nets to Phone Probability Estimation," IEEE Transactions on Neural Networks, vol. 5, no. 2, pp. 298-305, 1994.
[17] Oriol Vinyals, Suman Ravuri, and Daniel Povey, "Revisiting Recurrent Neural Networks for Robust ASR," in ICASSP, 2012.
[18] A. Maas, Q. Le, T. O Neil, O. Vinyals, P. Nguyen, and A. Ng, "Recurrent neural networks for noise reduction in robust asr," in INTERSPEECH, 2012.
[19] BOGERT, B. P.; HEALY, M. J. R.; TURKEY, J. W.: “The Quefrency Alanysis of Time Series for Echoes: Cepstrum, Pseudo Autocovariance, Cross-Cepstrum and Saphe Cracking”, Proceedings of the Symposium on Time Series Analysis, (M. Rosenblatt, Ed) Chapter 15, New York: Wiley, pp.209-243, 1963.