A Survey on Alleviating Cold – Start Problem in Lars* Using Hybrid System
Mili Mohan1 , Robert.S 2
Section:Survey Paper, Product Type: Journal Paper
Volume-3 ,
Issue-3 , Page no. 178-183, Mar-2015
Online published on Mar 31, 2015
Copyright © Mili Mohan , Robert.S . This is an open access article distributed under the Creative Commons Attribution License, which permits unrestricted use, distribution, and reproduction in any medium, provided the original work is properly cited.
View this paper at Google Scholar | DPI Digital Library
How to Cite this Paper
- IEEE Citation
- MLA Citation
- APA Citation
- BibTex Citation
- RIS Citation
IEEE Style Citation: Mili Mohan , Robert.S, “A Survey on Alleviating Cold – Start Problem in Lars* Using Hybrid System,” International Journal of Computer Sciences and Engineering, Vol.3, Issue.3, pp.178-183, 2015.
MLA Style Citation: Mili Mohan , Robert.S "A Survey on Alleviating Cold – Start Problem in Lars* Using Hybrid System." International Journal of Computer Sciences and Engineering 3.3 (2015): 178-183.
APA Style Citation: Mili Mohan , Robert.S, (2015). A Survey on Alleviating Cold – Start Problem in Lars* Using Hybrid System. International Journal of Computer Sciences and Engineering, 3(3), 178-183.
BibTex Style Citation:
@article{Mohan_2015,
author = {Mili Mohan , Robert.S},
title = {A Survey on Alleviating Cold – Start Problem in Lars* Using Hybrid System},
journal = {International Journal of Computer Sciences and Engineering},
issue_date = {3 2015},
volume = {3},
Issue = {3},
month = {3},
year = {2015},
issn = {2347-2693},
pages = {178-183},
url = {https://www.ijcseonline.org/full_paper_view.php?paper_id=444},
publisher = {IJCSE, Indore, INDIA},
}
RIS Style Citation:
TY - JOUR
UR - https://www.ijcseonline.org/full_paper_view.php?paper_id=444
TI - A Survey on Alleviating Cold – Start Problem in Lars* Using Hybrid System
T2 - International Journal of Computer Sciences and Engineering
AU - Mili Mohan , Robert.S
PY - 2015
DA - 2015/03/31
PB - IJCSE, Indore, INDIA
SP - 178-183
IS - 3
VL - 3
SN - 2347-2693
ER -
![]() |
![]() |
![]() |
2498 | 2346 downloads | 2526 downloads |
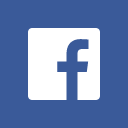
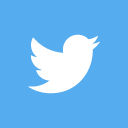
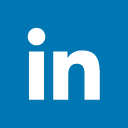
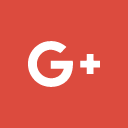
Abstract
Number of people who uses internet and websites for various purposes is increasing at an astonishing rate. More and more people rely on online sites for purchasing rented movies, songs, apparels, books etc. The competition between numbers of sites forced the web site owners to provide personalized services to their customers. So the recommender systems came into existence. LARS* is a location-aware recommender system that uses location based ratings to produce recommendations. LARS* supports a taxonomy of three novel classes of location-based ratings, namely, spatial ratings for non-spatial items, non-spatial ratings for spatial items, and spatial ratings for spatial items. The item based collaborative filtering used for generating recommendations in LARS* suffers from cold start problem. In cold start problem, the recommenders cannot draw inferences for users who are new to the system (new user problem) and for items which does not have sufficient ratings (new item problem). New user cold start problem can be resolved by utilizing the demographic data explicitly given by a user. Also the content based filtering does not suffer from new item cold start problem. From the survey carried out, a hybrid recommender system which exploits the demographic and content based filtering features can be used for alleviating cold start problem.
Key-Words / Index Term
Location Aware Recommender System, Collaborative filtering, cold-start problem, demographic filtering, content based filtering, Hybrid Systems
References
[1] Mohamed Sarwat, Justin J. Levandoski, Ahmed Eldawy, and Mohamed F. Mokbel, “LARS*: An Effcient and Scalable Location- Aware Recommender System, "IEEE Transactions On Knowledge And Data Engineering, Vol. 26, No. 6, June 2014
[2] G. Adomavicius and A. Tuzhilin, “Toward the next generation of recommender systems: A survey of the state-of-the-art and possible extensions," IEEE Trans. Knowl. Data Eng., vol. 17, no. 6, pp. 734-749, Jun. 2005.
[3] G. Linden, B. Smith, and J. York, “Amazon.com recommendations: Item-to-item collaborative filtering," IEEE Internet Comput., vol. 7, no. 1, pp. 76{80, Jan./Feb. 2003.
[4] B. Sarwar, G. Karypis, J. Konstan, and J. Riedl, “Item-based collaborative filtering recommendation algorithms,"in Proc. Int. Conf. WWW, Hong Kong, China, 2001.
[5] James Salter and Nick Antonopoulos,”CinemaScreen Recommender Agent: Combining Collaborative and Content-Based Filtering "in IEEE Computer Society, jan/feb ,2006.
[6] Eduardo Castillejo and Aitor Almeida and Diego Lopez-de-Ipina , “Social Network Analysis Applied to Recommendation Systems: Alleviating the Cold-User Problem," in Ubiquitous Computing and Ambient Intelligence,Lecture Notes in Computer Science Volume 7656, 2012, pp 306-313
[7] Pasquale Lops, Marco de Gemmis and Giovanni Semeraro, “Content-based Recommender Systems: State of the Art and Trends, "in Springer Science+Business Media, LLC 2011
[8] M. Pazzani, “A Framework for Collaborative, Content-Based, and Demographic Filtering,"Arti_cial Intelligence Rev., pp. 393-408, Dec. 1999.
[9] Laila Safoury and Akram Salah, “Exploiting User Demographic Attributes for Solving Cold- Start Problem in Recommender System," Lecture Notes on Software Engineering, Vol. 1, No. 3, August 2013
[10] Robin Burke, “Hybrid Recommender Systems: Survey and Experiments,"1997
[11] Jens Grivolla, Toni Badia,Diego Campo, Miquel Sonsona, Jose-Miguel Pulido, “A hybrid recommender combining user, item and interaction data,"European Union's Seventh Framework Programme managed by REA-Research Executive Agency http://ec.europa.eu/research/rea
[12] Chris Anderson, “Recommender systems for e-shops, "Business Mathematics and Informaticspaper,2011