A Survey on KASR for Big Data Applications
hakhy P S1 , Vidya K S2
Section:Survey Paper, Product Type: Journal Paper
Volume-3 ,
Issue-4 , Page no. 85-89, Apr-2015
Online published on May 04, 2015
Copyright © Shakhy P S , Vidya K S . This is an open access article distributed under the Creative Commons Attribution License, which permits unrestricted use, distribution, and reproduction in any medium, provided the original work is properly cited.
View this paper at Google Scholar | DPI Digital Library
How to Cite this Paper
- IEEE Citation
- MLA Citation
- APA Citation
- BibTex Citation
- RIS Citation
IEEE Style Citation: Shakhy P S , Vidya K S, “A Survey on KASR for Big Data Applications,” International Journal of Computer Sciences and Engineering, Vol.3, Issue.4, pp.85-89, 2015.
MLA Style Citation: Shakhy P S , Vidya K S "A Survey on KASR for Big Data Applications." International Journal of Computer Sciences and Engineering 3.4 (2015): 85-89.
APA Style Citation: Shakhy P S , Vidya K S, (2015). A Survey on KASR for Big Data Applications. International Journal of Computer Sciences and Engineering, 3(4), 85-89.
BibTex Style Citation:
@article{S_2015,
author = {Shakhy P S , Vidya K S},
title = {A Survey on KASR for Big Data Applications},
journal = {International Journal of Computer Sciences and Engineering},
issue_date = {4 2015},
volume = {3},
Issue = {4},
month = {4},
year = {2015},
issn = {2347-2693},
pages = {85-89},
url = {https://www.ijcseonline.org/full_paper_view.php?paper_id=467},
publisher = {IJCSE, Indore, INDIA},
}
RIS Style Citation:
TY - JOUR
UR - https://www.ijcseonline.org/full_paper_view.php?paper_id=467
TI - A Survey on KASR for Big Data Applications
T2 - International Journal of Computer Sciences and Engineering
AU - Shakhy P S , Vidya K S
PY - 2015
DA - 2015/05/04
PB - IJCSE, Indore, INDIA
SP - 85-89
IS - 4
VL - 3
SN - 2347-2693
ER -
![]() |
![]() |
![]() |
2534 | 2439 downloads | 2442 downloads |
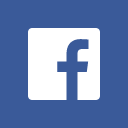
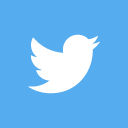
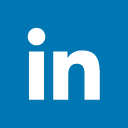
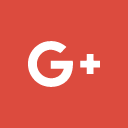
Abstract
Service recommender systems are valuable tools for providing appropriate recommendations to users. In the last decade the rapid growth of the number of customers, services and other online information yields service recommender systems in Big Data environment, some critical challenges .Traditional service recommender systems often suffer from scalability and inefficiency problems when processing or analyzing such large scale data. Moreover, most of the existing service recommender systems present the same ratings and rankings of services to different users without considering diverse users' preferences, and therefore fails to meet users' personalized requirements . KASR(Keyword Aware Service Recommendation System) aims at calculating a personalized rating of each candidate service for a user by extracting keywords from user reviews, and then presenting a personalized service recommendation list and recommending the most appropriate services to users. Various limitations of the current recommendation methods can be reduced by possible extensions that can provide better recommendation capabilities. These extensions include incorporation of the contextual information into the recommendation process. Designing and implementing scalable recommender systems in Big Data environment solve the scalability problem.
Key-Words / Index Term
Keyword Aware Service Recommendation System , Collaborative Filtering, BigData
References
[1] Shunmei Meng, Wanchun Dou, Xuyun Zhang, Jinjun Chen, “ KASR: A Keyword Aware Service Recommendation Method on MapReduce for Big Data Applications” IEEE Transactions on Parallel and Distributed Systems, TPDS-2013-12-1141.
[2] Gediminas Adomavicius, and Alexander Tuzhilin “Toward the Next Generation of Recommender Systems: A Survey of the State-of-the-Art and Possible Extensions” IEEE Transactions on Knowledge and Data Engineering, Vol. 17, No. 6, June 2005.
[3] G. Linden, B. Smith, and J. York, “Amazon.com Recommendations: Item-to-Item Collaborative Filtering,” IEEE Internet Computing, Vol. 7, No.1, pp. 76-80, 2003.
[4] K. Lakiotaki, N. F. Matsatsinis, and A. Tsoukis ,“Multi-Criteria User Modeling in Recommender Systems”, IEEE Intelligent Systems, Vol. 26, No. 2, pp. 64-76, 2011.
[5] J. Dean, and S. Ghemawat, “MapReduce: Simplified data processing on large clusters,” Communications of the ACM, Vol. 51, No.1, pp. 107-113, 2005.
[6] Daniar Asanov “Algorithms and Methods in Recommender Systems” Berlin Institute of Technology Berlin, Germany.
[7]Osman Khalid Muhammed Usman Shahid Khan, Samee U Khan , Albert Y Zomaya “Omnisuggest: A Ubiquitous Cloud Based Context Aware Recommendation System for Mobile Social Networks ” IEEE Transactions on Services Computing ,2013.
[8] Sang Hyun Choi, Young-Seon Jeong, and Myong K. Jeong “A Hybrid Recommendation Method with Reduced Data for Large-Scale Application” IEEE Transactions on systems, man, and cybernetics—Part C: Applications and Reviews, Vol. 40, No. 5, September 2010
[9] Building Recommendation Platforms with Hadoop, Jayant Shekhar O’Reilly Strata Conference Making Data Work.
[10] “Recommender Systems Handbook” Xavier Amatriain, Alejandro Jaimes, Nuria Oliver, and Josep M. Pujol Springer Science+Business Media, LLC 2011,pp 39-67.
[11] Recommender Systems , Daniel Rodriguez, University of Alcala ,Windsor ,August 5,2013