Beyond Volume: Enhancing Data Quality in Big Data Analytics through Frameworks and Metrics
Rajesh Remala1 , Divya Marupaka2 , Krishnamurty Raju Mudunuru3
- Independent Researcher, San Antonio, Texas, USA.
- Independent Researcher, Irvine, California, USA.
- Independent Researcher, San Antonio, Texas, USA.
Section:Research Paper, Product Type: Journal Paper
Volume-12 ,
Issue-4 , Page no. 39-46, Apr-2024
CrossRef-DOI: https://doi.org/10.26438/ijcse/v12i4.3946
Online published on Apr 30, 2024
Copyright © Rajesh Remala, Divya Marupaka, Krishnamurty Raju Mudunuru . This is an open access article distributed under the Creative Commons Attribution License, which permits unrestricted use, distribution, and reproduction in any medium, provided the original work is properly cited.
View this paper at Google Scholar | DPI Digital Library
How to Cite this Paper
- IEEE Citation
- MLA Citation
- APA Citation
- BibTex Citation
- RIS Citation
IEEE Style Citation: Rajesh Remala, Divya Marupaka, Krishnamurty Raju Mudunuru, “Beyond Volume: Enhancing Data Quality in Big Data Analytics through Frameworks and Metrics,” International Journal of Computer Sciences and Engineering, Vol.12, Issue.4, pp.39-46, 2024.
MLA Style Citation: Rajesh Remala, Divya Marupaka, Krishnamurty Raju Mudunuru "Beyond Volume: Enhancing Data Quality in Big Data Analytics through Frameworks and Metrics." International Journal of Computer Sciences and Engineering 12.4 (2024): 39-46.
APA Style Citation: Rajesh Remala, Divya Marupaka, Krishnamurty Raju Mudunuru, (2024). Beyond Volume: Enhancing Data Quality in Big Data Analytics through Frameworks and Metrics. International Journal of Computer Sciences and Engineering, 12(4), 39-46.
BibTex Style Citation:
@article{Remala_2024,
author = {Rajesh Remala, Divya Marupaka, Krishnamurty Raju Mudunuru},
title = {Beyond Volume: Enhancing Data Quality in Big Data Analytics through Frameworks and Metrics},
journal = {International Journal of Computer Sciences and Engineering},
issue_date = {4 2024},
volume = {12},
Issue = {4},
month = {4},
year = {2024},
issn = {2347-2693},
pages = {39-46},
url = {https://www.ijcseonline.org/full_paper_view.php?paper_id=5677},
doi = {https://doi.org/10.26438/ijcse/v12i4.3946}
publisher = {IJCSE, Indore, INDIA},
}
RIS Style Citation:
TY - JOUR
DO = {https://doi.org/10.26438/ijcse/v12i4.3946}
UR - https://www.ijcseonline.org/full_paper_view.php?paper_id=5677
TI - Beyond Volume: Enhancing Data Quality in Big Data Analytics through Frameworks and Metrics
T2 - International Journal of Computer Sciences and Engineering
AU - Rajesh Remala, Divya Marupaka, Krishnamurty Raju Mudunuru
PY - 2024
DA - 2024/04/30
PB - IJCSE, Indore, INDIA
SP - 39-46
IS - 4
VL - 12
SN - 2347-2693
ER -
![]() |
![]() |
![]() |
23 | 50 downloads | 8 downloads |
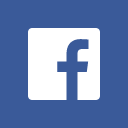
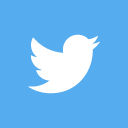
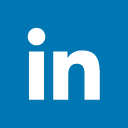
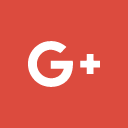
Abstract
The paper delves into various frameworks designed to address data quality concerns, highlighting their key components and methodologies. Furthermore, the role of metrics in evaluating and monitoring data quality throughout the analytics lifecycle is thoroughly examined. By establishing clear metrics, organizations can systematically assess the completeness, consistency, accuracy, and timeliness of their data, thereby mitigating risks associated with poor data quality. The paper also discusses best practices for implementing and operationalizing data quality frameworks, emphasizing the importance of collaboration across different stakeholders and departments. Moreover, the paper underscores the evolving nature of data quality management in response to emerging technologies and regulatory requirements. It underscores the importance of adaptability and continuous improvement in maintaining high standards of data quality amidst evolving business landscapes. Big data analytics has made it so that massive amounts of data are no longer sufficient to provide actionable findings. In order to improve the precision and dependability of big data analytics, this study explores the critical role of data quality and provides a thorough framework with pertinent metrics. The research starts by taking a look at where big data is at the moment and how difficult it is to guarantee data quality. Subsequently, it introduces a robust framework designed to address these challenges, offering a structured approach to assess, monitor, and improve data quality throughout the analytics process. Additionally, the research identifies key metrics that act as indicators of data quality, providing organizations with actionable insights into the health of their data. Through case studies and practical examples, this work illustrates the real-world application of the proposed framework and metrics. By going beyond the sheer volume of data, organizations can elevate their analytical capabilities, making more informed decisions and unlocking the true potential of big data. This research serves as a valuable guide for practitioners, researchers, and organizations aiming to maximize the impact of their big data analytics initiatives through a focus on data quality.
Key-Words / Index Term
Data Quality, Big Data Analytics, Frameworks, Metrics, Reliability, Accuracy.
References
[1]. Kimball R., & Ross M. The data warehouse toolkit: The definitive guide to dimensional modeling. John Wiley & Sons. 2013.
[2]. Lee, Y. W., Strong, D. M., Kahn, B. K., & Wang, R. Y. AIMQ: a methodology for information quality assessment. Information & Management, Vol.40, Issue.2, pp.133-146, 2002.
[3]. Loshin, D. Big data analytics: From strategic planning to enterprise integration with tools, techniques, NoSQL, and graph. Elsevier. 2013.
[4]. Redman, T. C. Data-driven: Creating a data culture. Harvard Business Press. 2008.
[5]. Wang, R. Y., & Strong, D. M. Beyond accuracy: What data quality means to data consumers. Journal of Management Information Systems, Vol.12, Issue.4, pp.5-33, 1996.
[6]. H. J. Watson and B. H. Wixom, "The Current State of Business Intelligence," in Computer, Vol.40, No.9, pp.96-99, Sept.2007.
[7]. Chen M, Mao S, Liu Y. Big data: A survey. Mobile Netw Appl. 19: pp.171–209, 2014.
[8]. Chiang F, Miller RJ. Discovering data quality rules. Proceed VLDB Endowment. Vol.1, Issue.1, pp.1166–1177, 2008.
[9]. P. Z. Yeh and C. A. Puri, "An Efficient and Robust Approach for Discovering Data Quality Rules," 2010 22nd IEEE International Conference on Tools with Artificial Intelligence, Arras, France, pp.248-255, 2010.
[10]. P. Ciancarini, F. Poggi and D. Russo, "Big Data Quality: A Roadmap for Open Data," 2016 IEEE Second International Conference on Big Data Computing Service and Applications (BigDataService), Oxford, UK, pp.210-215, 2016.
[11]. Firmani, D., Mecella, M., Scannapieco, M. et al. On the Meaningfulness of “Big Data Quality” (Invited Paper). Data Sci. Eng. 1, pp.6–20, 2016.
[12]. Rivas, B., Merino, J., Serrano, M., Caballero, I., Piattini, M., I8K|DQ-BigData: I8K Architecture Extension for Data Quality in Big Data, in: Advances in Conceptual Modeling, Lecture Notes in Computer Science. Presented at the International Conference on Conceptual Modeling, Springer, Cham, pp.164–172, 2015.
[13]. Manyika, J., Chui, M., Brown, B., Bughin, J., Dobbs, R., Roxburgh, C., Byers, A.H., Big data: The next frontier for innovation, competition, and productivity. McKinsey Global Institute pp.1–137, 2011.
[14]. Chen CP, Zhang C-Y. Data-intensive applications, challenges, techniques and technologies: A survey on Big Data. Inf Sci; 275: pp.314–47, 2014.
[15]. Hashem IAT, Yaqoob I, Anuar NB, Mokhtar S, Gani A, Ullah Khan S. The rise of “big data” on cloud computing: Review and open research issues. Inf Syst; 47: pp.98–115, 2015.
[16]. H. Hu, Y. Wen, T. -S. Chua and X. Li, "Toward Scalable Systems for Big Data Analytics: A Technology Tutorial," in IEEE Access, vol. 2, pp.652-687, 2014.
[17]. Wielki J. The Opportunities and Challenges Connected with Implementation of the Big Data Concept. In: Mach-Król M, Olszak CM, Pe?ech-Pilichowski T, editors. Advances in ICT for Business. Springer International Publishing: Industry and Public Sector, Studies in Computational Intelligence; pp.171–89, 2015.
[18]. M. A. -u. -d. Khan, M. F. Uddin and N. Gupta, "Seven V`s of Big Data understanding Big Data to extract value," Proceedings of the 2014 Zone 1 Conference of the American Society for Engineering Education, Bridgeport, CT, USA, pp.1-5, 2014.