Performance Evaluation on Real-time object detection using DL techniques
B. Sai Jyothi1 , Chavali Saathvika Durga Abhinaya2 , Bellamkonda Lahari3 , Chinta Devika Priya4 , Devarapalli Anjali5 , Bathula Sri Navya6
- Dept. of Information Technology, Vasireddy Venkatadri Institute of Technology, Guntur, AP, India.
- Dept. of Information Technology, Vasireddy Venkatadri Institute of Technology, Guntur, AP, India.
- Dept. of Information Technology, Vasireddy Venkatadri Institute of Technology, Guntur, AP, India.
- Dept. of Information Technology, Vasireddy Venkatadri Institute of Technology, Guntur, AP, India.
- Dept. of Information Technology, Vasireddy Venkatadri Institute of Technology, Guntur, AP, India.
- Dept. of Information Technology, Vasireddy Venkatadri Institute of Technology, Guntur, AP, India.
Section:Research Paper, Product Type: Journal Paper
Volume-12 ,
Issue-4 , Page no. 75-80, Apr-2024
CrossRef-DOI: https://doi.org/10.26438/ijcse/v12i4.7580
Online published on Apr 30, 2024
Copyright © B. Sai Jyothi, Chavali Saathvika Durga Abhinaya, Bellamkonda Lahari, Chinta Devika Priya, Devarapalli Anjali, Bathula Sri Navya . This is an open access article distributed under the Creative Commons Attribution License, which permits unrestricted use, distribution, and reproduction in any medium, provided the original work is properly cited.
View this paper at Google Scholar | DPI Digital Library
How to Cite this Paper
- IEEE Citation
- MLA Citation
- APA Citation
- BibTex Citation
- RIS Citation
IEEE Style Citation: B. Sai Jyothi, Chavali Saathvika Durga Abhinaya, Bellamkonda Lahari, Chinta Devika Priya, Devarapalli Anjali, Bathula Sri Navya, “Performance Evaluation on Real-time object detection using DL techniques,” International Journal of Computer Sciences and Engineering, Vol.12, Issue.4, pp.75-80, 2024.
MLA Style Citation: B. Sai Jyothi, Chavali Saathvika Durga Abhinaya, Bellamkonda Lahari, Chinta Devika Priya, Devarapalli Anjali, Bathula Sri Navya "Performance Evaluation on Real-time object detection using DL techniques." International Journal of Computer Sciences and Engineering 12.4 (2024): 75-80.
APA Style Citation: B. Sai Jyothi, Chavali Saathvika Durga Abhinaya, Bellamkonda Lahari, Chinta Devika Priya, Devarapalli Anjali, Bathula Sri Navya, (2024). Performance Evaluation on Real-time object detection using DL techniques. International Journal of Computer Sciences and Engineering, 12(4), 75-80.
BibTex Style Citation:
@article{Jyothi_2024,
author = {B. Sai Jyothi, Chavali Saathvika Durga Abhinaya, Bellamkonda Lahari, Chinta Devika Priya, Devarapalli Anjali, Bathula Sri Navya},
title = {Performance Evaluation on Real-time object detection using DL techniques},
journal = {International Journal of Computer Sciences and Engineering},
issue_date = {4 2024},
volume = {12},
Issue = {4},
month = {4},
year = {2024},
issn = {2347-2693},
pages = {75-80},
url = {https://www.ijcseonline.org/full_paper_view.php?paper_id=5682},
doi = {https://doi.org/10.26438/ijcse/v12i4.7580}
publisher = {IJCSE, Indore, INDIA},
}
RIS Style Citation:
TY - JOUR
DO = {https://doi.org/10.26438/ijcse/v12i4.7580}
UR - https://www.ijcseonline.org/full_paper_view.php?paper_id=5682
TI - Performance Evaluation on Real-time object detection using DL techniques
T2 - International Journal of Computer Sciences and Engineering
AU - B. Sai Jyothi, Chavali Saathvika Durga Abhinaya, Bellamkonda Lahari, Chinta Devika Priya, Devarapalli Anjali, Bathula Sri Navya
PY - 2024
DA - 2024/04/30
PB - IJCSE, Indore, INDIA
SP - 75-80
IS - 4
VL - 12
SN - 2347-2693
ER -
![]() |
![]() |
![]() |
9 | 17 downloads | 5 downloads |
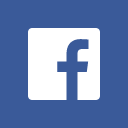
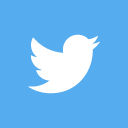
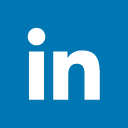
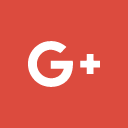
Abstract
Objects are located by drawing a bounding box around the detected object. One of computer vision`s specialties is object detection, which finds things in an image or video. Techniques for object detection are the foundation of the area of artificial intelligence. Typically, object detection uses deep learning and machine learning to yield accurate and significant findings. It is essentially made up of localization and classification. The state-of-the-art techniques utilized for real-time object detection have advanced recently. This study paper compares state-of-the-art techniques, such as faster region convolutional neural networks (Faster R-CNN) and you only look once V8 (YOLOV8). These algorithms are deep neural network representations, or neural networks with numerous hidden layers. Although each of these algorithms is notable for its own distinctiveness, they are compared to see which is superior. This study focuses on determining which of these algorithms is more practical to employ despite sharing a common core, namely CNNs.
Key-Words / Index Term
YOLOV8(You only look once), Faster region convolutional neural network (faster R-CNN), object detection, deep learning, deep neural networks, and convolutional neural networks.
References
[1] S. Ren, K. He, R. Girshick, and J. Sun, “Faster R-CNN: Towards Real-Time Object Detection with Region Proposal Networks.” arXiv, Jan. 06, 2016. Accessed: Jun. 17, 2023. [Online]. Available: http://arxiv.org/abs/1506.01497.
[2] S. J. Redmon and A. Farhadi, “YOLOv3: An Incremental Improvement.” arXiv, apr. 08, 2018. Accessed: Sep. 25, 2022. [Online]. Available: http://arxiv.org/abs/1804.0276.
[3] A.M.A.ghani Abdulghani and G.G. Menekse Dalveren, “Moving Object Detection in Video with Algorithms YOLO and Faster R-CNN in Different Conditions,” European Journal of Science and Technology, Jan. 2022, DOI: 10.31590/ejosat.1013049.
[4] H. Jiang and E. Learned-Miller, Face Detection with the Faster R-CNN.” arXiv, Jun. 10, 2016. Accessed: Sep. 25, 2022. [Online].Available: http://arxiv.org/abs/1606.03473.
[5] Chandana, R. K., & Ramachandra, A. C. Real time object detection system with YOLO and CNN 740 models: A review. arXiv preprint arXiv:2208.00773, 2022.
[6] JiayiFan; JangHyeon, Lee; InSuJung; YongKeunLee, “Improvement of Object Detection Based on Faster R-CNN and YOLO”, International Technical Conference on Circuits/Systems, Computers and Communications (ITC-CSCC) June, pp.27-30 2021. DOI: 10.1109/ITC-CSCC52171.2021.9501480.
[7] J. Kim, J.-Y. Sung, and S. Park, “Comparison of Faster-RCNN, YOLO, and SSD for Real-Time Vehicle Type Recognition,” in 2020 IEEE International Conference on Consumer Electronics - Asia (ICCE-Asia), Seoul, Korea (South): IEEE, Nov. pp.1–4, 2020.
DOI: 10.1109/ICCE-Asia49877.2020.9277040.
[8] F. Miao, Y. Tian, and L. Jin, “Vehicle Direction Detection Based on YOLOv3,” in 2019 11th International Conference on Intelligent Human-Machine Systems and Cybernetics (IHMSC), Hangzhou, China: IEEE, Aug., pp.268–271, 2019.
DOI: 10.1109/IHMSC.2019.10157.
[9] Rohan, A., Rabah, M., & Kim, S. H. Convolutional neural network-based real-time object detection and tracking for parrot AR drone 2. IEEE access, 7, pp.69575- 69584, 2019.
[10] Younis, A., Shixin, L., Jn, S., & Hai, Z. (January). Real-time object detection using pre- trained deep learning models MobileNet-SSD. In Proceedings of 2020 6th International Conference on Computing and Data Engineering, pp.44-48, 2020.
[11] Nguyen, N. D., Do, T., Ngo, T. D., & Le, D. D. An evaluation of deep learning methods for small object detection. Journal of electrical and computer engineering, 2020, pp.1-18, 2020.
[12] Hossain, S., & Lee, D. J. Deep learning-based real- time multiple-object detection and tracking from aerial imagery via a flying robot with GPU-based embedded devices. Sensors, Vol.19, Issue.15, pp.33-71, 2019.
[13] Pal, S. K., Pramanik, A., Maiti, J., & Mitra, P. Deep learning in multi-object detection and tracking: state of the art. Applied Intelligence, 51, pp.6400-6429, 2021.
[14] J. Du, Understanding of Object Detection Based on CNN Family and YOLO,” J. Phys.: Conf. Ser., Apr., Vol.1004, pp.12-29, 2018. DOI: 10.1088/1742-6596/1004/1/012029.
[15] S. Ren, K. He, R. Girshick, and J. Sun, Faster R-CNN: Towards Real-Time Object Detection with Region Proposal Networks,” IEEE Trans. Pattern Anal. Mach. Intell., Jun., Vol.39, No.6, pp.1137–1149, 2017, DOI: 10.1109/TPAMI.2016.2577031.