Face Matching for Similar Faces Evaluation from Videos Using Low Level Facial Geometries
Devendra Sakharkar1 , Sonali Bodkhe2
Section:Research Paper, Product Type: Journal Paper
Volume-3 ,
Issue-8 , Page no. 85-89, Aug-2015
Online published on Aug 31, 2015
Copyright © Devendra Sakharkar , Sonali Bodkhe . This is an open access article distributed under the Creative Commons Attribution License, which permits unrestricted use, distribution, and reproduction in any medium, provided the original work is properly cited.
View this paper at Google Scholar | DPI Digital Library
How to Cite this Paper
- IEEE Citation
- MLA Citation
- APA Citation
- BibTex Citation
- RIS Citation
IEEE Style Citation: Devendra Sakharkar , Sonali Bodkhe, “Face Matching for Similar Faces Evaluation from Videos Using Low Level Facial Geometries,” International Journal of Computer Sciences and Engineering, Vol.3, Issue.8, pp.85-89, 2015.
MLA Style Citation: Devendra Sakharkar , Sonali Bodkhe "Face Matching for Similar Faces Evaluation from Videos Using Low Level Facial Geometries." International Journal of Computer Sciences and Engineering 3.8 (2015): 85-89.
APA Style Citation: Devendra Sakharkar , Sonali Bodkhe, (2015). Face Matching for Similar Faces Evaluation from Videos Using Low Level Facial Geometries. International Journal of Computer Sciences and Engineering, 3(8), 85-89.
BibTex Style Citation:
@article{Sakharkar_2015,
author = {Devendra Sakharkar , Sonali Bodkhe},
title = {Face Matching for Similar Faces Evaluation from Videos Using Low Level Facial Geometries},
journal = {International Journal of Computer Sciences and Engineering},
issue_date = {8 2015},
volume = {3},
Issue = {8},
month = {8},
year = {2015},
issn = {2347-2693},
pages = {85-89},
url = {https://www.ijcseonline.org/full_paper_view.php?paper_id=614},
publisher = {IJCSE, Indore, INDIA},
}
RIS Style Citation:
TY - JOUR
UR - https://www.ijcseonline.org/full_paper_view.php?paper_id=614
TI - Face Matching for Similar Faces Evaluation from Videos Using Low Level Facial Geometries
T2 - International Journal of Computer Sciences and Engineering
AU - Devendra Sakharkar , Sonali Bodkhe
PY - 2015
DA - 2015/08/31
PB - IJCSE, Indore, INDIA
SP - 85-89
IS - 8
VL - 3
SN - 2347-2693
ER -
![]() |
![]() |
![]() |
2447 | 2378 downloads | 2326 downloads |
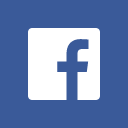
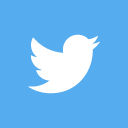
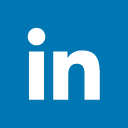
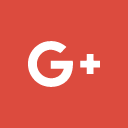
Abstract
The enhancement of digital devices and the popularity of social networking sites like Facebook, twitter, Instagram etc. The large numbers of peoples are shearing their images and videos by different social networking sites. The users are very much interested in uploading the images or videos on the internet in which most of the photos and videos contain faces. Thus with the rapidly growing photos and videos on the internet the large scale content base face image retrieval is a facilitating technology for many prominent applications. In this project, our aim is to detect a human face image which is present in the video frame and retrieving the similar human face images from the large scale database. By using human attributes in a systematic and scalable framework. The attribute-enhanced sparse coding is used to improve the performance of face retrieval in the offline stage. With this method the performance improvement to greater extent. Experimenting on public photo and video datasets, the result shows that the implementation of above method by using video.
Key-Words / Index Term
Face image, human attributes, content-based image retrieval, Face image retrieval, Face occurrences in videos
References
[1] D. Wang, S. C. Hoi, Y. He, and J. Zhu, “Retrieval-based face annotation by weak label regularized local coordinate coding,” ACM Multimedia, 2011.
[2] U. Park and A. K. Jain, “Face matching and retrieval using soft biometrics,” IEEE Transactions on Information Forensics and Security,2010.
[3] B.-C. Chen, Y.-H. Kuo, Y.-Y. Chen, K.-Y. Chu, and W. Hsu, “Semi-supervised face image retrieval using sparse coding with identity con-straint,” ACM Multimedia, 2011.
[4] M. Douze and A. Ramisa and C. Schmid, “Combining Attributes and Fisher Vectors for Efficient Image Retrieval,” IEEE Conference onComputer Vision and Pattern Recognition, 2011.
[5] N. Kumar, A. C. Berg, P. N. Belhumeur, and S. K. Nayar, “Describable visual attributes for face verification and image search,” in IEEE Transactions on Pattern Analysis and Machine Intelligence (PAMI), Special Issue on Real-World Face Recognition, Oct 2011.
[6] Y. Freund, R.E. Schapire, “Experiments with a New Boosting Algorithm”,In Proc. of the IEEE International Conference on Machine Learning (ICML), pp. 148–156, Bari, Italy, 1996.
[7] P. Viola, M. Jones, “Rapid Object Detection using a Boosted Cascade of Simple Features”, IEEE Conference on Computer Vision and Pattern Recognition (CVPR), 2001, pp. 511–518.
[8] J. Zobel and A. Moffat, “Inverted files for text search engines,” ACMComputing Surveys, 2006.
[9] A. Gionis, P. Indyk, and R. Motwani, “Similarity search in high dimensions via hashing,” VLDB, 1999.
[10] J. Sivic and A. Zisserman, “Video google: A text retrieval approach to object matching in videos,” International Conference on Computer Vision, 2003.
[11] D. Lowe, “Distinctive image features from scale-invariant keypoints,” International Journal of Computer Vision, 2003.
[12] L. Wu, S. C. H. Hoi, and N. Yu, “Semantics-preserving bag-of-words models and applications,” Journal of IEEE Transactions on image processing, 2010.
[13] Y.-H. Kuo, H.-T. Lin, W.-H. Cheng, Y.-H. Yang, and W. H. Hsu, “Unsupervised auxiliary visual words discovery for large-scale image object retrieval,” IEEE Conference on Computer Vision and PatternRecognition, 2011.
[14] N. Kumar, A. C. Berg, P. N. Belhumeur, and S. K. Nayar, “Describable visual attributes for face verification and image search,” in IEEE Transactions on Pattern Analysis and Machine Intelligence (PAMI), Special Issue on Real-World Face Recognition, Oct 2011.
[15] V. Blanz, S. Romdhani, and T. Vetter, “Face Identification across Different Poses and Illuminations with a 3D Morphable Model,” Proc. IEEE Int’l Conf. Automatic Face and Gesture Recognition, 2002.
[16] A.S. Georghiades, P.N. Belhumeur, and D.J. Kriegman, “From Few to Many: Illumination Cone Models for Face Recognition under Variable Lighting and Pose,” IEEE Trans. Pattern Analysis and Machine Intelligence, vol. 23, no. 6, pp. 643-660, June 2001.
[17] R. Gross, J. Shi, and J. Cohn, “Quo Vadis Face Recognition?” Proc. Workshop Empirical Evaluation Methods in Computer Vision, Dec.2001.
[18] B. Siddiquie, R. S. Feris, and L. S. Davis, “Image ranking and retrieval based on multi-attribute queries,” IEEE Conference on Computer Vision and Pattern Recognition, 2011.
[19] W. Scheirer, N. Kumar, K. Ricanek, T. E. Boult, and P. N. Belhumeur, “Fusing with context: a bayesian approach to combining descriptive attributes,” International Joint Conference on Biometrics, 2011.
[20] W. Scheirer and N. Kumar and P. Belhumeur and T. Boult, “Multi-Attribute Spaces: Calibration for Attribute Fusion and Similarity Search,” IEEE Conference on Computer Vision and Pattern Recognition, 2012.
[21] W. J. Scheirer, A. Rocha, R.Michaels, and T. E. Boult. Meta-Recognition: The Theory and Practice of Recognition Score Analysis. IEEE TPAMI, 33(8):1689–1695, August 2011.
[22] W. J. Scheirer, A. Rocha, R. Micheals, and T. E. Boult. Robust Fusion: Extreme Value Theory for Recognition Score Normalization. In ECCV, September 2010
[23] Z. Wu, Q. Ke, J. Sun, and H.-Y. Shum, “Scalable face image retrieval with identity-based quantization and multi-reference re-ranking,” IEEE Conference on Computer Vision and Pattern Recognition, 2010.
[24] H. Jegou, M. Douze, and C. Schmid, “Hamming embedding and weak geometric consistency for large scale image search,” European Conference on Computer Vision, 2008.
[25] J. Mairal, F. Bach, J. Ponce, and G. Sapiro, “Online dictionary learning for sparse coding,” ICML, 2009.