Inventing Rising Topics in Social Networks through Link-Anomaly Detection
A Sasikanth1 , S Venkata Ramana2
Section:Review Paper, Product Type: Journal Paper
Volume-3 ,
Issue-9 , Page no. 65-70, Sep-2015
Online published on Oct 01, 2015
Copyright © A Sasikanth , S Venkata Ramana . This is an open access article distributed under the Creative Commons Attribution License, which permits unrestricted use, distribution, and reproduction in any medium, provided the original work is properly cited.
View this paper at Google Scholar | DPI Digital Library
How to Cite this Paper
- IEEE Citation
- MLA Citation
- APA Citation
- BibTex Citation
- RIS Citation
IEEE Style Citation: A Sasikanth , S Venkata Ramana, “Inventing Rising Topics in Social Networks through Link-Anomaly Detection,” International Journal of Computer Sciences and Engineering, Vol.3, Issue.9, pp.65-70, 2015.
MLA Style Citation: A Sasikanth , S Venkata Ramana "Inventing Rising Topics in Social Networks through Link-Anomaly Detection." International Journal of Computer Sciences and Engineering 3.9 (2015): 65-70.
APA Style Citation: A Sasikanth , S Venkata Ramana, (2015). Inventing Rising Topics in Social Networks through Link-Anomaly Detection. International Journal of Computer Sciences and Engineering, 3(9), 65-70.
BibTex Style Citation:
@article{Sasikanth_2015,
author = {A Sasikanth , S Venkata Ramana},
title = {Inventing Rising Topics in Social Networks through Link-Anomaly Detection},
journal = {International Journal of Computer Sciences and Engineering},
issue_date = {9 2015},
volume = {3},
Issue = {9},
month = {9},
year = {2015},
issn = {2347-2693},
pages = {65-70},
url = {https://www.ijcseonline.org/full_paper_view.php?paper_id=642},
publisher = {IJCSE, Indore, INDIA},
}
RIS Style Citation:
TY - JOUR
UR - https://www.ijcseonline.org/full_paper_view.php?paper_id=642
TI - Inventing Rising Topics in Social Networks through Link-Anomaly Detection
T2 - International Journal of Computer Sciences and Engineering
AU - A Sasikanth , S Venkata Ramana
PY - 2015
DA - 2015/10/01
PB - IJCSE, Indore, INDIA
SP - 65-70
IS - 9
VL - 3
SN - 2347-2693
ER -
![]() |
![]() |
![]() |
2404 | 2305 downloads | 2337 downloads |
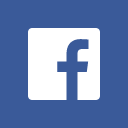
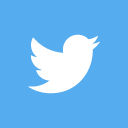
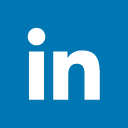
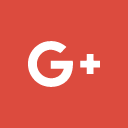
Abstract
Detection of rising topics is currently receiving revived interest impressed by the rapid climb of social networks. during this context, Conventional-term-frequency-based approaches might not be acceptable, as a result of the data changed in social-network posts embody not solely text however conjointly pictures, URLs, and videos. We have a tendency to specialize in emergence of topics signaled by social aspects of those networks. Specifically, we have a tendency to specialize in mentions of user links between users that are generated dynamically (intentionally or unintentionally) through replies, mentions, and retweets. we have a tendency to propose to notice the emergence of a replacement topic from the anomalies measured through the model and propose a chance model of the mentioning behavior of a social network user, and aggregating anomaly scores from many users, we have a tendency to show that we will notice rising topics solely supported the reply/mention relationships in social-network posts. we have a tendency to gathered from Twitter and incontestable the technique in many real knowledge sets. The experiments show that the projected mention-anomaly-based approaches will notice new topics a minimum of as early as text-anomaly-based approaches, and in some cases abundant earlier once the subject is poorly known by the matter contents in posts.
Key-Words / Index Term
TDT, Anomaly, SDNML, DTO
References
[1] J. Allan et al., “Topic Detection and Tracking Pilot Study: Final Report,” Proc. DARPA Broadcast News Transcription and UnderstandingWorkshop, 1998.
[2] J. Kleinberg, “Bursty and Hierarchical Structure in Streams,” DataMining Knowledge Discovery, vol. 7, no. 4, pp. 373-397, 2003.
[3] Y. Urabe, K. Yamanishi, R. Tomioka, and H. Iwai, “Real-Time Change-Point Detection Using Sequentially Discounting Normalized Maximum Likelihood Coding,” Proc. 15th Pacific-Asia Conf.Advances in Knowledge Discovery and Data Mining (PAKDD’ 11),2011.
[4] S. Morinaga and K. Yamanishi, “Tracking Dynamics of Topic Trends Using a Finite Mixture Model,” Proc. 10th ACM SIGKDD Int’l Conf. Knowledge Discovery and Data Mining, pp. 811-816, 2004.
[5] Q. Mei and C. Zhai, “Discovering Evolutionary Theme Patterns from Text: An Exploration of Temporal Text Mining,” Proc. 11th ACM SIGKDD Int’l Conf. Knowledge Discovery in Data Mining,pp. 198-207, 2005.
[6] A. Krause, J. Leskovec, and C. Guestrin, “Data Association for Topic Intensity Tracking,” Proc. 23rd Int’l Conf. Machine Learning(ICML’ 06), pp. 497-504, 2006.
[7] R Shiva Shankar, P.Neelima, V. Priyadarshini and D. Ravibabu “An Object Oriented Approach for Evaluating the Error Correction Coding ,” International Journal of Engineering Research & Technology, (IJERT), ISSN No. 2278-0181, Vol.3,Issue No. 4, pp.1322-1327, 2014.
[8] D. He and D.S. Parker, “Topic Dynamics: An Alternative Model of Bursts in Streams of Topics,” Proc. 16th ACM SIGKDD Int’l Conf. Knowledge Discovery and Data Mining, pp. 443-452, 2010.
[9] H. Small, “Visualizing Science by Citation Mapping,” J. Am. Soc. Information Science, vol. 50, no. 9, pp. 799-813, 1999.
[10] D. Aldous, “Exchangeability and Related Topics,” _ Ecole d’ _ Ete´ de Probabilite´s de Saint-Flour XIII—1983, pp. 1-198, Springer, 1985.
[11] Y. Teh, M. Jordan, M. Beal, and D. Blei, “Hierarchical Dirichlet Processes,” J. Am. Statistical Assoc., vol. 101, no. 476, pp. 1566-1581, 2006.
[12] M. Chilakarao, D. Ravibabu and R Shiva Shankar, and “A Realistic And Efficient Information Gathering In Tree Based Wireless Sensor Networks ,” nternational Journal of Advanced Research in Computer Science, (IJARCS), ISSN No. 0976-5697, Vol.5, No. 2, pp.53-57, 2014.
[13] J. Rissanen, “Strong Optimality of the Normalized ML Models as Universal Codes and Information in Data,” IEEE Trans. Information Theory, vol. 47, no. 5, pp. 1712-1717, July 2001.
[14] T. Roos and J. Rissanen, “On Sequentially Normalized Maximum Likelihood Models,” Proc. Workshop Information Theoretic Methods in Science and Eng., 2008.
[15] J. Rissanen, T. Roos, and P. Myllyma¨ki, “Model Selection by Sequentially Normalized Least Squares,” J. Multivariate Analysis, vol. 101, no. 4, pp. 839-849, 2010.
[16] C. Giurc_aneanu, S. Razavi, and A. Liski, “Variable Selection in Linear Regression: Several Approaches Based on Normalized Maximum Likelihood,” Signal Processing, vol. 91, pp. 1671-1692, 2011.