Comparative Analysis of Linked Unsupervised based Feature Selection Framework for Social Media Data
Pradeepa. T1 , Shanmugapriya.B 2
Section:Research Paper, Product Type: Journal Paper
Volume-3 ,
Issue-11 , Page no. 39-44, Nov-2015
Online published on Nov 30, 2015
Copyright © Pradeepa. T , Shanmugapriya.B . This is an open access article distributed under the Creative Commons Attribution License, which permits unrestricted use, distribution, and reproduction in any medium, provided the original work is properly cited.
View this paper at Google Scholar | DPI Digital Library
How to Cite this Paper
- IEEE Citation
- MLA Citation
- APA Citation
- BibTex Citation
- RIS Citation
IEEE Style Citation: Pradeepa. T , Shanmugapriya.B, “Comparative Analysis of Linked Unsupervised based Feature Selection Framework for Social Media Data,” International Journal of Computer Sciences and Engineering, Vol.3, Issue.11, pp.39-44, 2015.
MLA Style Citation: Pradeepa. T , Shanmugapriya.B "Comparative Analysis of Linked Unsupervised based Feature Selection Framework for Social Media Data." International Journal of Computer Sciences and Engineering 3.11 (2015): 39-44.
APA Style Citation: Pradeepa. T , Shanmugapriya.B, (2015). Comparative Analysis of Linked Unsupervised based Feature Selection Framework for Social Media Data. International Journal of Computer Sciences and Engineering, 3(11), 39-44.
BibTex Style Citation:
@article{T_2015,
author = {Pradeepa. T , Shanmugapriya.B},
title = {Comparative Analysis of Linked Unsupervised based Feature Selection Framework for Social Media Data},
journal = {International Journal of Computer Sciences and Engineering},
issue_date = {11 2015},
volume = {3},
Issue = {11},
month = {11},
year = {2015},
issn = {2347-2693},
pages = {39-44},
url = {https://www.ijcseonline.org/full_paper_view.php?paper_id=723},
publisher = {IJCSE, Indore, INDIA},
}
RIS Style Citation:
TY - JOUR
UR - https://www.ijcseonline.org/full_paper_view.php?paper_id=723
TI - Comparative Analysis of Linked Unsupervised based Feature Selection Framework for Social Media Data
T2 - International Journal of Computer Sciences and Engineering
AU - Pradeepa. T , Shanmugapriya.B
PY - 2015
DA - 2015/11/30
PB - IJCSE, Indore, INDIA
SP - 39-44
IS - 11
VL - 3
SN - 2347-2693
ER -
![]() |
![]() |
![]() |
2650 | 2442 downloads | 2382 downloads |
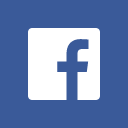
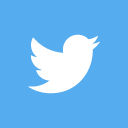
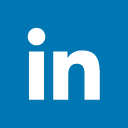
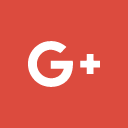
Abstract
The explosive usage of social media produces massive amount of unlabeled and high- dimensional data. Feature selection has been proven to be effective in dealing with high-dimensional data for efficient learning and data mining. Unsupervised learning has been proven to be a powerful technique in unsupervised feature selection, which allows embedding feature selection into the classification (or regression) problem. In literature several numbers of feature selection methods such as supervised feature selection algorithms and unsupervised feature selection methods have been proposed to select dimensional feature in the social network. When compare to supervised methods ,unsupervised feature selection methods performs well since it perform operation without label information .But unsupervised feature selection is particularly difficult due to the definition of relevancy of features becomes unclear. To solve this problem , in this paper study a unsupervised feature selection algorithm the concept of pseudo-class labels to guide extracting constraints from link information and attribute- value information, resulting in a new Linked Unsupervised based feature selection framework (LUFS), for linked social media data. LUFS examine the differences between social media data and traditional attribute value data; investigate how the relations extracted from linked data can be exploited to help select relevant features for linked social media data. Furthermore, social theories are developed by sociologists to explain the formation of links in social media. Experimental results on various social media datasets demonstrate the effectiveness of the proposed framework LUFS is compared with existing schemas in terms of accuracy and Normalized Mutual Information (NMI). Design and conduct systemic experiments to evaluate the proposed framework on data sets from real-world social media websites.
Key-Words / Index Term
Unsupervised Feature Selection, Linked Data, Social Media, Pseudo Labels, Social Dimension Regularization.
References
[1]. Tang, J., & Liu, H. (2014). An unsupervised feature selection framework for social media data. Knowledge and Data Engineering, IEEE Transactions on, 26(12), 2914-2927.
[2]. H. Liu and H. Motoda,(2008) Computational Methods of Feature Selection. London, U.K. Chapman & Hall
[3]. H. Liu and L. Yu, (Apr. 2005) “Toward integrating feature selection algorithms for classification and clustering,” IEEE Trans. Knowl. Data Eng., vol. 17, no. 4, pp. 491–502.
[4]. Huan Liu and Hiroshi Motoda. (2007) Computational methods of feature selection. CRC Press.
[5]. Zheng Zhao, Lei Wang, and Huan Liu. (July 11-15, 2010) Efficient spectral feature selection with minimum redundancy. In Proceedings of the Twenty-Fourth AAAI Conference on Artificial Intelligence, AAAI 2010, Atlanta, Georgia, USA.
[6]. Jiliang Tang, Salem Alelyani, and Huan Liu(2014) Feature selection for classification: A review. In Data Classification: Algorithms and Applications.
[7]. Jiliang Tang and Huan Liu.(2012) Unsupervised feature selection for linked social media data. In Proceedings of the 18th ACM SIGKDD international conference on Knowledge discovery and data mining, pages 904–912. ACM,
[8]. Salem Alelyani, Jiliang Tang, and Huan Liu.(2013) Feature selection for clustering: A review. In Data Clustering: Algorithms and Applications, pages 29–60. CRC Press.
[9]. Mingjie Qian and Chengxiang Zhai. Robust unsupervised feature selection. In Proceedings of the Twenty-Third international joint conference on Artificial Intelligence, pages 1621–1627. AAAI Press.
[10]. Zheng Zhao and Huan Liu(2013) Spectral feature selection for supervised and unsupervised learning. In Proceedings of the 24th international conference on Machine learning, pages 1151–1157. ACM.
[11]. Deng Cai, Chiyuan Zhang, and Xiaofei(2010) The Unsupervised feature selection for multi-cluster data. In Proceedings of the 16th ACM SIGKDD international conference on Knowledge discovery and data mining, pages 333–342. ACM.
[12]. X. He, D. Cai, and P. Niyogi, “Laplacian score for feature selection,” in Proc. Adv. Neural Inf. Process. Syst, pp. 507–514.
[13]. J. Dy and C. Brodley,(2006) “Feature selection for unsupervised learning,” J. Mach. Learn. Res., vol. 5, pp. 845–889, 2004.
[14]. Z. Zhao and H. Liu,(2007) “Spectral feature selection for supervised and unsupervised learning,” in Proc. 24th Int. Conf. Mach. Learn., pp. 1151–1157.
[15]. Constantinopoulos, C., Titsias, M. K., & Likas, A. (2006). Bayesian feature and model selection for Gaussian mixture models. IEEE Transactions on Pattern Analysis and Machine Intelligence, 28(6), 1013-1018.
[16]. Y. Yang, H. Shen, Z. Ma, Z. Huang, and X. Zhou, (2011)“L21-norm regularized discriminative feature selection for unsupervised learning,” in Proc. 22nd Int. Joint Conf. Artif. Intell., pp. 1589–1594.
[17]. J. Tang and H. Liu,(2012) “Feature selection with linked data in social media,” in Proc. 13th SIAM Int. Conf. Data Mining, pp. 118– 128.
[18]. P. Marsden and N. Friedkin, (1993) “Network studies of social influence,” Sociol. Methods Res., vol. 22, no. 1, pp. 127–151.
[19]. M. McPherson, L. S. Lovin, and J. M. Cook,(2001) “Birds of a feather: Homophily in social networks,” Annu. Rev. Sociol., vol. 27, pp. 415–444.
[20]. Tang, J., & Liu, H. (2014). An unsupervised feature selection framework for social media data. Knowledge and Data Engineering, IEEE Transactions on,26(12), 2914-2927.