A Study on Benchmarking Parameters for Intelligent Systems
Rajesh Misir1 , Malay Mitra2 , R. K. Samanta3
Section:Research Paper, Product Type: Conference Paper
Volume-03 ,
Issue-01 , Page no. 10-17, Feb-2015
Online published on Feb 18, 2015
Copyright © Rajesh Misir , Malay Mitra , R. K. Samanta . This is an open access article distributed under the Creative Commons Attribution License, which permits unrestricted use, distribution, and reproduction in any medium, provided the original work is properly cited.
View this paper at Google Scholar | DPI Digital Library
How to Cite this Paper
- IEEE Citation
- MLA Citation
- APA Citation
- BibTex Citation
- RIS Citation
IEEE Style Citation: Rajesh Misir , Malay Mitra , R. K. Samanta, “A Study on Benchmarking Parameters for Intelligent Systems,” International Journal of Computer Sciences and Engineering, Vol.03, Issue.01, pp.10-17, 2015.
MLA Style Citation: Rajesh Misir , Malay Mitra , R. K. Samanta "A Study on Benchmarking Parameters for Intelligent Systems." International Journal of Computer Sciences and Engineering 03.01 (2015): 10-17.
APA Style Citation: Rajesh Misir , Malay Mitra , R. K. Samanta, (2015). A Study on Benchmarking Parameters for Intelligent Systems. International Journal of Computer Sciences and Engineering, 03(01), 10-17.
BibTex Style Citation:
@article{Misir_2015,
author = {Rajesh Misir , Malay Mitra , R. K. Samanta},
title = {A Study on Benchmarking Parameters for Intelligent Systems},
journal = {International Journal of Computer Sciences and Engineering},
issue_date = {2 2015},
volume = {03},
Issue = {01},
month = {2},
year = {2015},
issn = {2347-2693},
pages = {10-17},
url = {https://www.ijcseonline.org/full_spl_paper_view.php?paper_id=2},
publisher = {IJCSE, Indore, INDIA},
}
RIS Style Citation:
TY - JOUR
UR - https://www.ijcseonline.org/full_spl_paper_view.php?paper_id=2
TI - A Study on Benchmarking Parameters for Intelligent Systems
T2 - International Journal of Computer Sciences and Engineering
AU - Rajesh Misir , Malay Mitra , R. K. Samanta
PY - 2015
DA - 2015/02/18
PB - IJCSE, Indore, INDIA
SP - 10-17
IS - 01
VL - 03
SN - 2347-2693
ER -
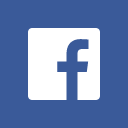
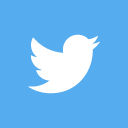
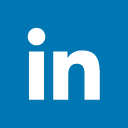
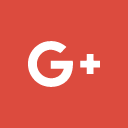
Abstract
Intelligent automated decision support systems are now found to be very much useful in various fields. In bioinformatics and machine learning in general, there is a large variation in the predictive measures that are used to evaluate intelligent systems. If we do not assess the accuracy of model's prediction, a vital step in model development, its application will have little merit. This work critically discusses different approaches to assess predictive performance and various test statistics. Choice of assessing strategy or validation for a specific application helps in determining the suitability of the model and in comparing the performances of different modeling techniques. The purpose of this paper is to serve as an introduction to various important benchmarking parameters and as a guide for using them in research.
Key-Words / Index Term
Predictive performance, Confusion Matrix, Receiver operating characteristic (ROC), Akaike information criteria (AIC), Kappa statistic, Lift, Cumulative gain, Probability Threshold
References
[1] Z. Lu et al., Predicting Subcellular Localization of Proteins using Machine Learned Classifiers, Bioinformatics, vol 20, issue 4, pp. 547-556, 2004.
[2] R. Eisner et al., Improving Protein Function Prediction using the Hierarchical Structure of the Gene Ontology, IEEE Symposium on Computational Intelligence in Bioinformatics and Computational Biology, Nov-2005. --
[3] J. A. Swets, Measuring the accuracy of diagnostic systems. Science, 240, pp. 1285–1293,1988.
[4] Xiao-Hua Zhou and Jaroslaw Harezlak, Comparison of bandwidth selection methods for kernel smoothing of ROC curves, Statist. Med.; 21:2045–2055, 2002.
[5] Tom Fawcett, An introduction to ROC analysis , Pattern Recognition Letters, vol 27, pp. 861-874, 2006.
[6] Jacob Cohen, A coefficient of agreement for nominal scales, Educational and Psychological Measurement, 20 (1), pp. 37–46, 1960.
[7] J.R. Landis., G.G. Koch, The measurement of observer agreement for categorical data, Biometrics, vol 33, no. 1, pp 159-174, 1977.
[8] H. Akaike, Information Theory and An Extension of The Maximum Likelihood Principle. In B.N. Petrov and F. Caske (eds.), Second International Symposium on Information Theory. Budapest: Akademiai Kiado, 267-281, 1973.
[9] H. Akaike, A Bayesian Analysis of The Minimum AIC procedure, Ann. Inst. Statist. Math., vol 30, no. 1, pp. 9-14, 1978.
[10] G. Schwarz, Estimating the Dimension of a Model, The Annals of Statistics, Vol. 6, no. 2, pp. 461-464, 1978.
[11] R. E. Kass and L. Wasserman, A Reference Bayesian Test for Nested Hypotheses and its Relationship to the Schwarz Criterion, J. American Stat. Association, Vol. 90, no. 431, pp. 928-934, 1995.
[12] Tariq Jaffery, Shirley X. Liu, Measuring Campaign Performance by Using Cumulative Gain Lift Chart, SAS Global Forum 2009, Paper 196, 2009.
[13] S. J. Philips et al., Maximum entropy modeling of species geographic distributions, Ecological Modelling, Vol. 190, pp. 231–259, 2006.
[14] C. Liu et al, Selecting thresholds of occurrence in the prediction of species distributions. Ecography 28, pp. 385-393, 2005.
[15] R. G. Pearson, Species' Distribution Modeling for Conservation Educators and Practitioners, Synthesis. American Museum of Natural History, 2007. Available at http://ncep.amnh.org.
[16] S. Manel et al., Comparing discriminant analysis ,neural networks and logistic regression for prediction species distributions: a case study with a Himalayan river bird. Ecological Modelling, 120, 337-347, 1999.
[17] M. P. Robertson et al., A PCA-based modeling technique for predicting environmental suitability for organisms from presence records, Diversity and Distributions 7, 15-27, 2001.
[18] R. G. Pearson et al., . Model based uncertainty in species' range prediction, Journal of Biogeography, 33, pp. 1704-1711, 2006.
[19] S. J. Phillips et al., Maximum entropy modeling of species geographic distributions, Ecological Modelling , Vol. 190, pp. 231-259, 2006.
[20] R. G. Pearson et al., . Modelling species distributions in britain: A hierarchical integration of climate and land-cover data, Ecography, 27, 285-298, 2004.
[21] S. Manel et al., Evaluating presences absence models in ecology: the need to account for prevalence, Journal of Applied Ecology, Vol. 38, pp.,921-931, 2001.
[22] B. Huntley et al., Modelling present and potential future ranges of some European higher plants using climate response surfaces. Journal of Biogeography, Vol. 22, pp.967-1001, 1995.
[23] J. Elith et al., Novel methods improve prediction of species' distributions from occurrence data, Ecography, Vol. 29, pp. 129-151, 2006.
[24] J. S. Cramer,. Logit models: from economics and other fields. Cambridge University Press, 2003.
J. Pearce et al., Evaluating the predictive performance of habitat models developed using logistic regression, Ecological Modelling, Vol. 133, pp. 225–245, 2000.