Generalized Anxiety Disorder : Prediction using ANN
Dilip Roy Chowdhury1 , Arpita Das2
Section:Research Paper, Product Type: Conference Paper
Volume-04 ,
Issue-01 , Page no. 48-56, Feb-2016
Online published on Feb 26, 2016
Copyright © Dilip Roy Chowdhury, Arpita Das . This is an open access article distributed under the Creative Commons Attribution License, which permits unrestricted use, distribution, and reproduction in any medium, provided the original work is properly cited.
View this paper at Google Scholar | DPI Digital Library
How to Cite this Paper
- IEEE Citation
- MLA Citation
- APA Citation
- BibTex Citation
- RIS Citation
IEEE Style Citation: Dilip Roy Chowdhury, Arpita Das, “Generalized Anxiety Disorder : Prediction using ANN,” International Journal of Computer Sciences and Engineering, Vol.04, Issue.01, pp.48-56, 2016.
MLA Style Citation: Dilip Roy Chowdhury, Arpita Das "Generalized Anxiety Disorder : Prediction using ANN." International Journal of Computer Sciences and Engineering 04.01 (2016): 48-56.
APA Style Citation: Dilip Roy Chowdhury, Arpita Das, (2016). Generalized Anxiety Disorder : Prediction using ANN. International Journal of Computer Sciences and Engineering, 04(01), 48-56.
BibTex Style Citation:
@article{Chowdhury_2016,
author = {Dilip Roy Chowdhury, Arpita Das},
title = {Generalized Anxiety Disorder : Prediction using ANN},
journal = {International Journal of Computer Sciences and Engineering},
issue_date = {2 2016},
volume = {04},
Issue = {01},
month = {2},
year = {2016},
issn = {2347-2693},
pages = {48-56},
url = {https://www.ijcseonline.org/full_spl_paper_view.php?paper_id=32},
publisher = {IJCSE, Indore, INDIA},
}
RIS Style Citation:
TY - JOUR
UR - https://www.ijcseonline.org/full_spl_paper_view.php?paper_id=32
TI - Generalized Anxiety Disorder : Prediction using ANN
T2 - International Journal of Computer Sciences and Engineering
AU - Dilip Roy Chowdhury, Arpita Das
PY - 2016
DA - 2016/02/26
PB - IJCSE, Indore, INDIA
SP - 48-56
IS - 01
VL - 04
SN - 2347-2693
ER -
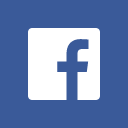
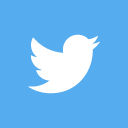
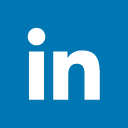
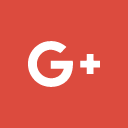
Abstract
Artificial Neural Network plays an important role in medical diagnostics field and used by medical practitioners and domain specialists for diagnosis and treatment with ultimate accuracy. In this paper, a medical diagnosis system is proposed for predicting Generalized Anxiety Disorder (GAD). In today’s world of computational Intelligence, Swarm Intelligence technique is one of the successive ways to solve hard medical problems. Particle Swarm Optimization (PSO) imitates the behavior of a swarm of insects or a group of fish or birds. In this paper the relative advantages of genetic algorithm, Particle Swarm Optimization and Artificial Neural Network (ANN) are combined to achieve the desired accuracy. ANN’s are often used as a powerful discriminating classifier for tasks in medical diagnosis for early detection of diseases. The data set on this study is composed of 200 patients with various sign symptoms. The objective of this paper is to determine the weights of the neural network using genetic algorithm in less number of iterations and PSO algorithm for feature Reduction. For training the network Quasi-newtonalgorithm is used in this study using various training algorithm parameters. The accuracy obtained using this approach is 98.56%.
Key-Words / Index Term
Artificial Neural Network (ANN); Genetic Algorithm (GA); Particle Swarm Optimization (PSO); Generalized Anxiety Disorder (GAD)
References
[1] Anxiety Dsorders Association of America Available: http://www.adaa.org
[2] ”Generalized Anxiety Disorder: When Worry Gets Out of Control”-national institute of mental Health U.S. Department of Health and Human Services national institutes of Health
[3] Singh Y, and Chauhan, A.S. “Neural Networks in Data mining”, Journal of Theoretical and Applied Information Technology, Vol. 5, No. 1, Pp. 37-42,2009
[4] Roy ChowdhuryDilip, BhattacharjeeDipanwita, "Swarm Intelligence and ANN Techniques for predicting Neonatal Disease" NCRTCSA-2014
[5] Gupta preeti,KaurBikrampal, “Accuracy Enhancement of Heart Disease Diagnosis System Using Neural Network and Genetic Algorithm”International Journal of Advanced Research in Computer Science and Software Engineering 4(8), August - 2014, pp. 160-166
[6] Sivagowry.S ,Dr.Durairaj.M “PSO - An Intellectual Technique for Feature Reduction on Heart Malady Anticipation Data”Volume 4, Issue 9, September 2014 ISSN: 2277 128X International Journal of Advanced Research in Computer Science and Software Engineering
[7] Valavanis, I.K.,School Of Electrical And Computer Engineering, National Technical University Of Athens, 9 heroonPolytechneiou Str,15780 Zographou, Geece, Mougiakakou, S.G; Grimaldi, Ka Nikita Ks,”. Analysis Of Postprandial Lipemia As A Cardiovascular Disease Risk factor Using Genetic And Clinical Information: An Artificial Neural network Perspective”, Engineering In Medicine And Biology Society 2008.Embs 2008.30th Annual International IEEE Embs Conference Vancouver, British Columbia, Canada, August 20-24, 2008 Pages 4609-4612.
[8] Goldberg E., “Genetic algorithms in search, optimization, and machine learning” , London, Addison-Wesley, 1989.
[9] KaregowdaGowda, Manjunath A.S. and JayaramM.A.,”Application of Genetic Agorithm optimized Neural Network connection weights for Medical Diagnosis of PIMA Indians Diabets”,International Journal on Soft Computing(IJSC), Vol.2,No.2,Pp. 15-23,2011
[10] Parimala M., Sumalatha G. and N. Zareena, “A heuristic Approach to the CHILLI Expert System using PSO Algorithm”, Int. J of Advanced research in Computer Science and Software Engineering(IJARCSSE),Vol.3,Issue 7, Pp. 1126-1130, ISSN: 2277 128X, July 2013
[11] Alperunler, Alper Murat and RatnaBabuChinnam, “m2PSO: A maximum relevance minimum redundancy feature selection method based on Swarm Intellignce for SVM Classification”, Elsevier, 2011, pp 4625-4641.
[12] Shi Y. and Eberhart R., “Parameter Selection in Particle Swarm Optimization”, proceedings of the Seventh Annual Conference on Evolutionary Programming,Pp.591-601,1998.
[13] D. R. Chowdhury, R.K Samanta and M. Chatterjee. "Artificial Neural Network Model for Neonatal Disease Diagnosis", International Journal of Artificial Intelligence and Expert Systems (IJAE), Volume (2) : Issue (3), 2011
[14] International Classification of Diseases(ICD)”. World Health Organization. Retrieved 23 November 2010.
[15] “Neuro Intelligence using Alyuda”, http://www.alyuda.com