The Enhancement of Character and Non-Character images using Extensive segmentation Techniques
S. Gopinathan1
Section:Research Paper, Product Type: Journal Paper
Volume-06 ,
Issue-04 , Page no. 8-13, May-2018
Online published on May 31, 2018
Copyright © S. Gopinathan . This is an open access article distributed under the Creative Commons Attribution License, which permits unrestricted use, distribution, and reproduction in any medium, provided the original work is properly cited.
View this paper at Google Scholar | DPI Digital Library
How to Cite this Paper
- IEEE Citation
- MLA Citation
- APA Citation
- BibTex Citation
- RIS Citation
IEEE Style Citation: S. Gopinathan, “The Enhancement of Character and Non-Character images using Extensive segmentation Techniques,” International Journal of Computer Sciences and Engineering, Vol.06, Issue.04, pp.8-13, 2018.
MLA Style Citation: S. Gopinathan "The Enhancement of Character and Non-Character images using Extensive segmentation Techniques." International Journal of Computer Sciences and Engineering 06.04 (2018): 8-13.
APA Style Citation: S. Gopinathan, (2018). The Enhancement of Character and Non-Character images using Extensive segmentation Techniques. International Journal of Computer Sciences and Engineering, 06(04), 8-13.
BibTex Style Citation:
@article{Gopinathan_2018,
author = {S. Gopinathan},
title = {The Enhancement of Character and Non-Character images using Extensive segmentation Techniques},
journal = {International Journal of Computer Sciences and Engineering},
issue_date = {5 2018},
volume = {06},
Issue = {04},
month = {5},
year = {2018},
issn = {2347-2693},
pages = {8-13},
url = {https://www.ijcseonline.org/full_spl_paper_view.php?paper_id=350},
publisher = {IJCSE, Indore, INDIA},
}
RIS Style Citation:
TY - JOUR
UR - https://www.ijcseonline.org/full_spl_paper_view.php?paper_id=350
TI - The Enhancement of Character and Non-Character images using Extensive segmentation Techniques
T2 - International Journal of Computer Sciences and Engineering
AU - S. Gopinathan
PY - 2018
DA - 2018/05/31
PB - IJCSE, Indore, INDIA
SP - 8-13
IS - 04
VL - 06
SN - 2347-2693
ER -
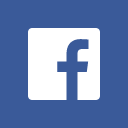
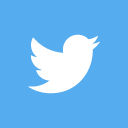
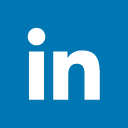
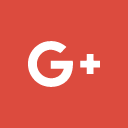
Abstract
The research work presents detection of different types of text in scene images based on extensive segmentation (proposed method) to generate character candidate regions. We usually consider many connected regions as candidates, which aim to capture character regions as many as possible. Key feature of exhaustive segmentation technique, which exactly segments character candidate region in scene images from non-character candidate region also. First, we detect candidate text regions using Maximally Stable External Region (MSER method) where the scene image has been converted to gray image and to find the text region. Second, the geometric properties of text on the image are used to filter out non-text regions using simple thresholds. Third, we remove the rest Non-Text region based on Stroke Width Variation (SWV). Finally, we merge the entire text region from the detection of Text and thus recognise the Detected Text in the scene image. We use public dataset, namely, the Street View Text dataset and some other language (Tamil, Hindi and Chinese) images to evaluate the performance of our(extensive segmentation) method. The experimental results are shown that our(extensive segmentation) method achieves excellent improvement in the detection of text though the images being blurred, low-resolution and small in size from the existing method(Yuenwang et al.). We also achieve considerable rate of recall with the executed images.
Key-Words / Index Term
digitization, Exhaustive segmentation, stroke Width Variation
References
[1] Yuanwang Wei, Zhijiang Zhang, Wei Shen, Dan Zeng,, Mei Fang, Shifu Zhou, “Text detection in scene images based on exhaustive segmentation”,2017, Signal Processing: Image communication, 50, pg 1-8.
[2] L. Neumann, J. Matas, Real-time scene text localization and recognition, in: 2012 IEEE Conference on Computer Vision and Pattern Recognition (CVPR), IEEE, Providence, RI USA, 2012, pp. 3538–3545.
[3] Lei Sun, QiangHuo, WeiJia, KaiChen, A robust approach for text detection from natural scene images, in: 2015 Pattern recognition, 48, pp. 2906-2920.
[4] Qixiang Ye, Qingming Huang, Wen Gao, Debin Zhao, Fast and Robust text detection in images and video frames, in :2005, Image and vision computing, 23, pp. 565 – 576.
[5] Juhua Liu, HaiSu, YaohuaYi, WenbinHu, Robust text detection via multi-degree of sharpening and blurring, in: 2016, Signal Processing, 124, pp. 259-265.
[6] B. Epshtein, E. Ofek, Y. Wexler, Detecting text in natural scenes with stroke width transform, in: 2010 IEEE Conference on Computer Vision and Pattern Recognition(CVPR), IEEE, San Francisco, California, USA, 2010, pp. 2963–2970.
[7] T. Wang, D.J. Wu, A. Coates, A.Y. Ng, End-to-end text recognition with convolutional neural networks, in: 2012 21st International Conference on Pattern Recognition (ICPR), IEEE, Tsukuba, 2012, pp. 3304–3308.
[8] K. Wang, B. Babenko, S. Belongie, End-to-end scene text recognition, in: 2011 IEEE International Conference on Computer Vision (ICCV), IEEE, Barcelona, Spain, 2011, pp. 1457–1464.
[9] K.I. Kim, K. Jung, J.H. Kim, Texture-based approach for text detection in images using support vector machines and continuously adaptive mean shift
[10] algorithm, IEEE Trans. Pattern Anal. Mach. Intell. 25 (12) (2003) 1631–1639.
[11] J. Gllavata, R. Ewerth, B. Freisleben, Text detection in images based on unsupervised classification of high-frequency wavelet coefficients, in: Proceedings of the 17th International Conference on Pattern Recognition, 2004. ICPR 2004, vol. 1, IEEE, Cambridge UK, 2004, pp. 425–428.
[12] L. Neumann, J. Matas, Text localization in real-world images using efficiently pruned exhaustive search, in: 2011 International Conference on Document Analysis and Recognition (ICDAR), IEEE, Beijing, China, 2011, pp. 687–691.
[13] T. Wang, D.J. Wu, A. Coates, A.Y. Ng, End-to-end text recognition with convolutional neural networks, in: 2012 21st International Conference on Pattern Recognition (ICPR), IEEE, Tsukuba, 2012, pp. 3304–3308.