Supervised Learning Architecture for Solving Double Dummy Bridge Problem
Dharmalingam Muthusamy1
Section:Research Paper, Product Type: Journal Paper
Volume-06 ,
Issue-04 , Page no. 30-37, May-2018
Online published on May 31, 2018
Copyright © Dharmalingam Muthusamy . This is an open access article distributed under the Creative Commons Attribution License, which permits unrestricted use, distribution, and reproduction in any medium, provided the original work is properly cited.
View this paper at Google Scholar | DPI Digital Library
How to Cite this Paper
- IEEE Citation
- MLA Citation
- APA Citation
- BibTex Citation
- RIS Citation
IEEE Style Citation: Dharmalingam Muthusamy, “Supervised Learning Architecture for Solving Double Dummy Bridge Problem,” International Journal of Computer Sciences and Engineering, Vol.06, Issue.04, pp.30-37, 2018.
MLA Style Citation: Dharmalingam Muthusamy "Supervised Learning Architecture for Solving Double Dummy Bridge Problem." International Journal of Computer Sciences and Engineering 06.04 (2018): 30-37.
APA Style Citation: Dharmalingam Muthusamy, (2018). Supervised Learning Architecture for Solving Double Dummy Bridge Problem. International Journal of Computer Sciences and Engineering, 06(04), 30-37.
BibTex Style Citation:
@article{Muthusamy_2018,
author = {Dharmalingam Muthusamy},
title = {Supervised Learning Architecture for Solving Double Dummy Bridge Problem},
journal = {International Journal of Computer Sciences and Engineering},
issue_date = {5 2018},
volume = {06},
Issue = {04},
month = {5},
year = {2018},
issn = {2347-2693},
pages = {30-37},
url = {https://www.ijcseonline.org/full_spl_paper_view.php?paper_id=354},
publisher = {IJCSE, Indore, INDIA},
}
RIS Style Citation:
TY - JOUR
UR - https://www.ijcseonline.org/full_spl_paper_view.php?paper_id=354
TI - Supervised Learning Architecture for Solving Double Dummy Bridge Problem
T2 - International Journal of Computer Sciences and Engineering
AU - Dharmalingam Muthusamy
PY - 2018
DA - 2018/05/31
PB - IJCSE, Indore, INDIA
SP - 30-37
IS - 04
VL - 06
SN - 2347-2693
ER -
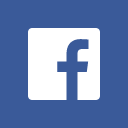
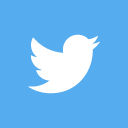
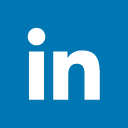
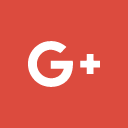
Abstract
The bridge game is one of the most generally known card games comprising many mesmerizing aspects, such as bidding, playing and winning the trick including estimation of human hand strength. The harmonizing input data based on the human knowledge of the game to improvement the quality of tricks. The bridge game classification under a game of imperfect information is to be equally well-defined. The decision made on any stage of the game is simply based on the assessment that was made on the immediate preceding stage. The intelligent game of bridge incompleteness of information, the real spirit of the card game in proceeding further deals of the game are taking into many forms especially during the distribution of cards for the next deal. The cascade correlation neural network architecture with supervised learning implemented in resilient back - propagation algorithm to train data and therefore to test data it is together along with the bamberger point count method and work point count methods.
Key-Words / Index Term
Cascade-correlation neural network, Resilient back-propagation algorithm, Bridge game, Double dummy bridge problem, Bamberger point count method, Work point count method
References
[1] B.Yegnanarayana, D.Khemani, M.Sarkar, “Neural networks for contract bridge bidding”, Sadhana, Vol.21, Issue.3, pp.395-413, 1996.
[2] A.Amit, S.Markovitch, “Learning to bid in bridge. Machine Learning”, Vol.63, Issue.3, pp.287-327, 2006.
[3] J.Mandziuk, K.Mossakowski, “Looking Inside Neural Networks Trained to Solve Double-Dummy Bridge Problems”, In the 5th Game-on International Conference on Computer Games: Artificial Intelligence, Design and Education (CGAIDE 2004),U.K, 2004.
[4] J.Mandziuk, K.Mossakowski, “Neural networks compete with expert human players in solving the Double Dummy Bridge Problem”, In the Proceedings of the 2009 IEEE symposium on computational intelligence and games (CIG 2009), Italy, pp. 117-124, 2009.
[5] K.Mossakowski, J.Mandziuk, “Artificial neural networks for solving double dummy bridge problems”, Lecture Notes in Computer Science (LNAI), Springer, Heidelberg, Vol. 3070, pp. 915-921, 2004.
[6] M.Sarkar, B.Yegnanarayana, D.Khemani, “Application of neural network in contract bridge bidding”, In the Proceedings of the 1995 national conference on neural networks and fuzzy methods, India, pp.144-151,1995.
[7] M.Dharmalingam, R.Amalraj, “Neural Network Architectures for Solving the Double Dummy Bridge Problem in Contract Bridge”, In the Proceedings of the 2013 PSG-ACM national conference on intelligent computing, India, pp. 31-37, 2013.
[8] M.Dharmalingam, R.Amalraj, “Artificial Neural Network Architecture for Solving the Double Dummy Bridge Problem in Contract Bridge”, Advanced Research in Computer and Communication Engineering, Vol.2, Issue.12, pp.4683-4691, 2013.
[9] H.Francis, A.Truscott, D.Francis, “The Official Encyclopedia of Bridge”, 6th ed. Memphis, American Contract Bridge League, 2001.
[10] K.Mossakowski, J.Mandziuk, “Learning without human expertise: A case study of Double Dummy Bridge Problem”, IEEE Transactions on Neural Networks. Vol.20, Issue.2, pp.278-299, 2009.
[11] B.Seifert, “Encyclopedia of Bridge”, Polish Scientific Publishers PWN, Warsaw, 1996.
[12] S.Haykin, “Neural Networks: A Comprehensive Foundation”, Prentice Hall, New York, 1998.
[13] K.Mossakowski, J.Mandziuk, “Neural networks and the estimation of hand’s strength in contract bridge”, Lecture Notes in Computer Science (LNAI), Springer, Heidelberg, Vol.4029, pp. 1189-1198, 2006.
[14] S.N.Sivanandam, S.N.Deepa, “Principles of Soft Computing”, Wiley, New Delhi, 2007.
[15] B.Yegnanarayana, “Artificial neural networks”, Prentice Hall, New Delhi, 2010.
[16] J.Mandziuk, “Computational intelligence in mind games”, In the Studies in computational intelligence, Springer, Heidelberg, 2007.
[17] J.Mandziuk, “Some thoughts on using computational intelligence methods in classical mind board games”, In the Proceedings of the 2008 International joint conference on neural networks (IJCNN 2008), China, pp. 4001-4007, 2008.
[18] J.Mandziuk, “Knowledge-free and learning- based methods in intelligent game playing”, Springer, Berlin, Heidelberg, 2010.
[19] M.Dharmalingam, R.Amalraj, “Back-Propagation Neural Network Architecture for Solving the Double Dummy Bridge Problem in Contract Bridge”, In the Proceedings of the 2014 IEEE international conference on intelligent computing applications (ICICA 2014), India, pp. 454-461,2014.
[20] M.Dharmalingam, R.Amalraj, “A Solution to the Double Dummy Bridge Problem in Contract Bridge Influenced by Supervised learning module Adapted by Artificial Neural Network”, In the Special Issue on distributed intelligent method and applications, Vol.5, Issue.1, pp.836- 843, 2014.
[21] M.Dharmalingam, R.Amalraj, “Artificial Neural Network Architectures: Work Point Count System Coupled with Back-Propagation and Resilient Back - Propagation Algorithm for Solving Double Dummy Bridge Problem in Contract Bridge”, International Journal of Innovative Science, Engineering and Technology, Vol.2, Issue.8, pp.142-157, 2015.
[22] M.Dharmalingam, R.Amalraj, “Artificial Neural Network Architectures for solving Double Dummy Bridge Problem in Imperfect Information Game”, International Journal of Computational Intelligence and Informatics, Vol.5, Issue.3, pp.188-197, 2015.
[23] M.Dharmalingam, “Point Count Systems in Imperfect Information Game”, International Research Journal of Engineering and Technology, Vol.3, Issue.1, pp.362-370, 2016.
[24] M.Dharmalingam, “Artificial Neural Network Architectures for Solving the Contract Bridge”, International Journal of Advanced Information and Communication Technology, Vol.4, Issue.3, pp.01-09, 2017.
[25] W.H. Root, “The ABCs of Bridge”, Three Rivers Press, 1998.
[26] M.Dharmalingam, R.Amalraj, “Supervised Learning in Imperfect Information Game”, Advanced Research in Computer Science, Vol.4, Issue.2, pp.195-200, 2013.
[27] T.Ando, N.Kobayashi, T.Uehara, “Cooperation and competition of agents in the auction of Computer Bridge”, Electronics and Communications in Japan, Part 3, Vol.86, Issue.12, pp.76-86, 2003.
[28] T.Ando, T.Uehara, “Reasoning by agents in computer bridge bidding”, In the Computers and Games, Lecture Notes in Computer Science, Springer, Berlin, Vol. 2063, pp. 346-364, 2001.
[29] I.Frank, D.A.Basin, “Optimal play against best Defence: Complexity and Heuristics”, Lecture Notes in Computer Science, Springer-Verlag, Germany, Vol.1558, pp.50-73, 1999.
[30] T.Ando, Y. Sekiya, T.Uehara, “Partnership bidding for Computer Bridge”, Systems and Computers, Japan, Vol.31, Issue.2, pp.72-82, 2000.
[31] I.Frank, D.A.Basin, “Strategies explained”, In the Proceedings of the 5th Game programming workshop, Japan, pp1-8, 1999.
[32] I.Frank, D.A.Basin, “A Theoretical and Empirical Investigation of Search in Imperfect Information Game”, Theory of Computer Science, Vol.252, Issue.1, pp.217-256, 2001.
[33] W.Jamroga, “Modeling artificial intelligence on a case of bridge card play bidding”, In the Proceedings of the 1999 international workshop on intelligent information method, pp.276-277, 1999.
[34] D.N.Levy, “The million pound bridge program”, In the Heuristic Programming in artificial intelligence - First Computer Olymiad, pp.95-103, 1989.
[35] J.Mandziuk, K.Mossakowski, “Example-based estimation of hand’s strength in the game of bridge with or without using explicit human knowledge”, In the Proceedings of the 2007 IEEE symposium on computational intelligence in data mining (CIDM 2007), Los Alamitos, pp. 413-420, 2007.
[36] S.J.J. Smith, D.S.Nau, T.A.Throop, “Computer bridge - a big win for AI planning”, Artificial intelligence, Vol.19, Issue.2, pp.93-106, 1998.
[37] S.J.J. Smith, D.S.Nau, T.A.Throop, “Success in Spades: Using AI Planning Techniques to Win the World Championship of Computer Bridge”, In the Proceedings of the 1998 national conference on artificial intelligence, pp.1079-1086, 1998.
[38] S.E.Fahlman, V.Lebiere, “The cascade-correlation learning architecture”, In the Advances in neural information processing methods, pp.524-532, 1990.
[39] M.Riedmiller, H.Braun, “A direct adaptive method for faster back propagation: the RPROP algorithm”, In the Proceedings of the 1993 IEEE international conference on neural networks (ICNN 1993), pp. 586- 591, 1993.
[40] R.Amalraj, M.Dharmalingam, “A work point count method coupled
[41] with back-propagation for solving double dummy bridge problem”, Neurocomputing, Vol.168, pp.160-178, 2015.
[42] M.L.Ginsberg, “GIB:Imperfect information in a computationally challenging game”, Artificial intelligence research, Vol.14, pp.303-358, 2001.