Role of Feature Extraction Techniques : PCA and LDA for Appearance Based Gait Recognition
K. Annbuselvi1 , N. Santhi2 , S. Sivakumar3
Section:Research Paper, Product Type: Journal Paper
Volume-06 ,
Issue-04 , Page no. 38-44, May-2018
Online published on May 31, 2018
Copyright © K. Annbuselvi, N. Santhi, S. Sivakumar . This is an open access article distributed under the Creative Commons Attribution License, which permits unrestricted use, distribution, and reproduction in any medium, provided the original work is properly cited.
View this paper at Google Scholar | DPI Digital Library
How to Cite this Paper
- IEEE Citation
- MLA Citation
- APA Citation
- BibTex Citation
- RIS Citation
IEEE Style Citation: K. Annbuselvi, N. Santhi, S. Sivakumar, “Role of Feature Extraction Techniques : PCA and LDA for Appearance Based Gait Recognition,” International Journal of Computer Sciences and Engineering, Vol.06, Issue.04, pp.38-44, 2018.
MLA Style Citation: K. Annbuselvi, N. Santhi, S. Sivakumar "Role of Feature Extraction Techniques : PCA and LDA for Appearance Based Gait Recognition." International Journal of Computer Sciences and Engineering 06.04 (2018): 38-44.
APA Style Citation: K. Annbuselvi, N. Santhi, S. Sivakumar, (2018). Role of Feature Extraction Techniques : PCA and LDA for Appearance Based Gait Recognition. International Journal of Computer Sciences and Engineering, 06(04), 38-44.
BibTex Style Citation:
@article{Annbuselvi_2018,
author = {K. Annbuselvi, N. Santhi, S. Sivakumar},
title = {Role of Feature Extraction Techniques : PCA and LDA for Appearance Based Gait Recognition},
journal = {International Journal of Computer Sciences and Engineering},
issue_date = {5 2018},
volume = {06},
Issue = {04},
month = {5},
year = {2018},
issn = {2347-2693},
pages = {38-44},
url = {https://www.ijcseonline.org/full_spl_paper_view.php?paper_id=355},
publisher = {IJCSE, Indore, INDIA},
}
RIS Style Citation:
TY - JOUR
UR - https://www.ijcseonline.org/full_spl_paper_view.php?paper_id=355
TI - Role of Feature Extraction Techniques : PCA and LDA for Appearance Based Gait Recognition
T2 - International Journal of Computer Sciences and Engineering
AU - K. Annbuselvi, N. Santhi, S. Sivakumar
PY - 2018
DA - 2018/05/31
PB - IJCSE, Indore, INDIA
SP - 38-44
IS - 04
VL - 06
SN - 2347-2693
ER -
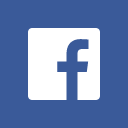
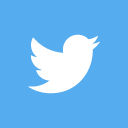
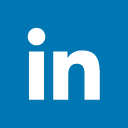
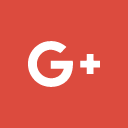
Abstract
Feature extraction is one of the most important step in image pattern recognition. Some sources of difficulty are the presence of irrelevant information and the relativity of a feature set to a particular application. Feature extraction and description are essential components of various computer vision applications. The concept of feature extraction and description refers to the process of identifying points in an image (interested points) that can be used to describe the image’s contents. The One major goal of feature extraction is to increase the accuracy of learned models by compactly extracting prominent features from the input data, while also possibly removing noise and redundancy from the input. Additional objectives include low-dimensional representations for data imagining and compression for the purpose of reducing data storage requirements as well as increasing training and implication speed. The aim of this paper is to report an descriptive study of most popular feature extraction methods PCA and LDA which are generally used in pattern recognition and the role of PCA and LDA in gait feature extraction.
Key-Words / Index Term
Feature extraction, PCA, LDA, Gait Feature Extraction
References
[1] Gaurav Kumar, Pradeep Kumar Bhatia, A Detailed Review of Feature Extraction in Image Processing Systems, 2014 Fourth International Conference on Advanced Computing & Communication Technologies.
[2] Turk M, Pentland A. Eigenfaces for recognition. J Cognitive Neurosci. 1991; 3(1):71–86.
[3] Lindsay I Smith, A tutorial on Principal Components Analysis, 2002.
[4] M. Murali, Principal Component Analysis based Feature Vector Extraction, Indian Journal of Science and Technology, Vol 8(35), 2015.
[5] Alaa Tharwat, Tarek Gaber, Abdelhameed Ibrahim, Aboul Ella Hassanien, Linear discriminant analysis: A detailed Tutorial, AI Communications, 2017.
[6] Han Su, Zhi-Wu Liao, Guo-Yue Chen, A gait recognition method using L1-PCA and LDA, IEEE, International Conference on Machine Learning and Cybernetics, 2009.
[7] Pranjit Das, Sarat Saharia, Human Gait Recognition Based on Principal Component Analysis, International Journal of Computer Sciences and Engineering, Volume-4, Special Issue-7, Dec 2016.
[8] Qiong Cheng, Bo Fu, and Hui Chen, Gait Recognition Based on PCA and LDA, Proceedings of the Second Symposium International Computer Science and Computational Technology(ISCSCT ’09), 2009.
[9] Gait recognition based on gait energy image and linear discriminant analysis, IEEE International conference on signal processing, communications and computing, 2015.
[10] Liang Wang, Tieniu Tan, Huazhong Ning, and Weiming Hu, Silhouette Analysis-Based Gait Recognition for Human Identification, IEEE Trans. on Pattern analysis and machine intelligence, vol. 25, no. 12, december 2003.
[11] T. Daoliang, H. Kaiqi, Y. Shiqi, and T. Tieniu, "Orthogonal Diagonal Projections for Gait Recognition," in 2007 IEEE International Conference on Image Processing, 2007, pp. I - 337-I - 340.
[12] P.N. Belhumeur, J. P. Hespanha, and D. J. Kriegman, "Eigenfaces vs. Fisherfaces: recognition using class specific linear projection," IEEE Transactions on Pattern Analysis and Machine Intelligence, vol. 19, pp. 711-720, 1997.
[13] T. Dacheng, L. Xuelong, W. Xindong, and S. J. Maybank, "General Tensor Discriminant Analysis and Gabor Features for Gait Recognition," IEEE Transactions on Pattern Analysis and Machine Intelligence, vol. 29, pp. 1700-1715, 2007.
[14] Y. Mu and D. Tao, "Biologically inspired feature manifold for gait recognition," Neurocomputing, vol. 73, pp. 895-902,2010.
[15] R. Hu, W. Shen, and H. Wang, "Recursive spatio temporal subspace learning for gait recognition," Neuro computing,vol. 73, pp. 1892-1899, 2010.
[16] E. Zhang, Y. Zhao, and W. Xiong, "Active energy imageplus 2DLPP for gait recognition," Signal Processing, vol.90, pp. 2295-2302, 2010.
[17] G. Baofeng and M. S. Nixon, "Gait Feature Subset Selectionby Mutual Information," IEEE Transactions on Systems,Man and Cybernetics, Part A: Systems and Humans vol. 39, pp. 36-46, 2009.
[18] G. Baofeng and M. S. Nixon, "Gait Feature Subset Selection by Mutual Information," in First IEEE International Conference on Biometrics: Theory, Applications, and Systems, 2007, pp. 1-6.
[19] Jinyan Chen Jiansheng Li, Average Gait Differential Image Based Human Recognition, Scientific World Journal. 2014. 27.
[20] Yang J, Zhang D, Frangi AF, Yang J-Y. Two-dimensional PCA: a new approach to appearance based face representation and recognition. IEEE Transactions on Pattern Analysis and Machine Intelligence. 2004;26(1):131–137.
[21] Worapan Kusakunniran, Qiang Wu, Hongdong Li, Jian Zhang, Multiple views gait recognition using View Transformation Model based on optimized Gait Energy Image, IEEE 12th International Conference on Computer Vision Workshops (ICCV Workshops), 2009
[22] Deng Cai, Xiao fei He, Jia wei Han.“Training Linear Discriminant Analysis in Linear Time,” IEEE International Conference on Data Engineering, 2008(24):pp. 209-217.
[23] Fradkin, Dmitriy1,Madigan, David2.“Experiments with random projections for machine learning,” IEEE 9th ACM SIGKDD International Conference on Knowledge Discovery and Data Mining, KDD`03. 2003(8):pp 517-522.