HM-SPCA: Hybrid Method for Automatic Detection of MA Using MinIMas with Sparse PCA in Diabetic Retinopathy
D. Ashok Kumar1 , A. Sankari2
Section:Research Paper, Product Type: Journal Paper
Volume-06 ,
Issue-04 , Page no. 71-80, May-2018
Online published on May 31, 2018
Copyright © D. Ashok Kumar, A. Sankari . This is an open access article distributed under the Creative Commons Attribution License, which permits unrestricted use, distribution, and reproduction in any medium, provided the original work is properly cited.
View this paper at Google Scholar | DPI Digital Library
How to Cite this Paper
- IEEE Citation
- MLA Citation
- APA Citation
- BibTex Citation
- RIS Citation
IEEE Style Citation: D. Ashok Kumar, A. Sankari, “HM-SPCA: Hybrid Method for Automatic Detection of MA Using MinIMas with Sparse PCA in Diabetic Retinopathy,” International Journal of Computer Sciences and Engineering, Vol.06, Issue.04, pp.71-80, 2018.
MLA Style Citation: D. Ashok Kumar, A. Sankari "HM-SPCA: Hybrid Method for Automatic Detection of MA Using MinIMas with Sparse PCA in Diabetic Retinopathy." International Journal of Computer Sciences and Engineering 06.04 (2018): 71-80.
APA Style Citation: D. Ashok Kumar, A. Sankari, (2018). HM-SPCA: Hybrid Method for Automatic Detection of MA Using MinIMas with Sparse PCA in Diabetic Retinopathy. International Journal of Computer Sciences and Engineering, 06(04), 71-80.
BibTex Style Citation:
@article{Kumar_2018,
author = {D. Ashok Kumar, A. Sankari},
title = {HM-SPCA: Hybrid Method for Automatic Detection of MA Using MinIMas with Sparse PCA in Diabetic Retinopathy},
journal = {International Journal of Computer Sciences and Engineering},
issue_date = {5 2018},
volume = {06},
Issue = {04},
month = {5},
year = {2018},
issn = {2347-2693},
pages = {71-80},
url = {https://www.ijcseonline.org/full_spl_paper_view.php?paper_id=360},
publisher = {IJCSE, Indore, INDIA},
}
RIS Style Citation:
TY - JOUR
UR - https://www.ijcseonline.org/full_spl_paper_view.php?paper_id=360
TI - HM-SPCA: Hybrid Method for Automatic Detection of MA Using MinIMas with Sparse PCA in Diabetic Retinopathy
T2 - International Journal of Computer Sciences and Engineering
AU - D. Ashok Kumar, A. Sankari
PY - 2018
DA - 2018/05/31
PB - IJCSE, Indore, INDIA
SP - 71-80
IS - 04
VL - 06
SN - 2347-2693
ER -
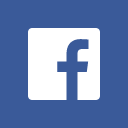
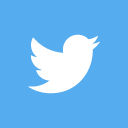
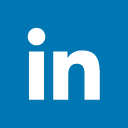
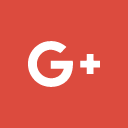
Abstract
Microaneurysms (MAs) is an earliest lesions in DR detection, its plays a challenging role in diabetic retinopathy (DR) diagnosis. It has been an active research in medical image processing and so many machine learning algorithms has been developed for MA detection. The First Stage of detection is consisting of clearer segmentation of optical disc area in retina using a new Minimum Intensity Maximum Solidity (MinIMas) algorithm on fundus dataset, then extract bright lesion and red lesion using Gaussian mixture models. Set of feature extracted that the second stage of the system, finally machine learning approach is a0pplied for lesion classification. In this paper, a hybrid of segmentation and unsupervised classification of sparse PCA (HM-SPCA) for MA detection is proposed so that enhanced output is obtained. This proposed algorithm achieves great analysis in lesion segmentation with minimum false alarm. Furthermore, effective features can be extracted due to sparse properties of PCA (Principle Component Analysis) which merge the elastic net penalty with PCA together. Thus, the projected DR detection system enhanced its performance by reducing false positives compared with existing algorithms in lesion classification, and hence this approach can be applied to improve the beneficence in earlier vision detection of patients for diabetic retinopathy.
Key-Words / Index Term
Diabetic Retinopathy, MA, Optic disk, Blood vessel, Classification, Sparse, PCA
References
[1] B. Antal, A. Hajdu, “An ensemble-based system for microaneurysm detection and diabetic retinopathy grading,” IEEE transactions on bio-medical engineering, vol. 59, no. 6, pp. 1720-6, 2012.
[2] D. B. Mukamel, G. H. Bresnick, J. C. Dickinson, D. R. Cole, “ A screening approach to the surveillance of patients with diabetes for the presence of vision-threatening retinopathy,” Ophthalmology, vol. 107, no. 1, pp. 19-24, 2000.
[3] M. Abràmoff, M. Garvin, M. Sonka, “Retinal imaging and image analysis,” IEEE Reviews in Biomedical Engineering, vol. 3, pp. 169–208, 2010.
[4] B. Antal, A. Hajdu, “Improving microaneurysm detection using an optimally selected subset of candidate extractors and preprocessing methods,” Pattern Recognition, vol. 45, no. 1, pp. 264-270, 2012.
[5] C. Sinthanayothin, J. F. Boyce, H. L. Cook, and T. H.Williamson,“Automated localisation of the optic disk, fovea, and retinal blood vessels from digital colour fundus images,” Br. J. phthalmol,, vol. 83, no. 8, pp. 902–910, 1999.
[6] T. Kauppi, V. Kalesnykiene, J.-K. Kmrinen, L. Lensu, I. Sorr, A. Raninen, R. Voutilainen, H. Uusitalo, H. Klviinen, and J. Pietil, “Diaretdb1 diabetic retinopathy database and evaluation protocol,” Proc. of the 11th Conf. on Medical Image Understanding and Analysis (MIUA2007), pp. 61–65, 2007.
[7] A.-H. Abdel-Razik Youssif, A. Ghalwash, and A. Abdel- Rahman Ghoneim, “Optic disc detection from normalized digital fundus images by means of a vessels’ direction matched filter,” IEEE Transactions on Medical Imaging, vol. 27, no. 1, pp. 11 –18, jan. 2008.
[8] J. Staal, M. Abramoff, M. Niemeijer, M. Viergever, and B. van Ginneken, “Ridge based vessel segmentation in color images of the retina,” IEEE Transactions on Medical Imaging, vol. 23, pp. 501–509, 2004.
[9] A. Hoover, and M. Goldbaum, “Locating the optic nerve in retinal image using the fuzzy convergence of bood vessels,” IEEE Transactions on Medical Imaging, vol. 22, August 2003.
[10] F. ter Haar, “Automatic localization of the optic disc in digital colour images of the human retina,” M.S. Thesis in Computer Science, UtrechtUniversity, Utrecht, 2005.
[11] M. Niemeijer, G. B. Van, J. Staal, M. S. Suttorp-Schulten, M. D. Abràmoff, “Automatic detection of red lesions in digital color fundus photographs,” IEEE Transactions on Medical Imaging, vol. 24, no. 5, pp. 584-92, 2005.
[12] C. I. Sánchez, “Mixture model-based clustering and logistic regression for automatic detection of microaneurysms in retinal images,” Proceedings of SPIE - The International Society for Optical Engineering, pp. 7260: 72601M-72601M-8, 2009.
[13] B. Zhang, F. Karray, Q. Li, L. Zhang, “Sparse representation classifier for microaneurysm detection and retinal blood vessel extraction,” Information Sciences, vol. 200, no. (1), pp.78-90, 2012.
[14] G. G. Gardner, D. Keating, T. H. Williamson, A. T. Elliott, “Automatic detection of diabetic retinopathy using an artificial neural network: a screening tool,” British Journal of Ophthalmology, vol. 80, no. 11, pp. 940-4, 1996.
[15] T. Walter, P. Massin, A. Erginay, R. Ordonez, C. Jeulin, J.C. Klein, “Automatic detection of microaneurysms in color fundus images,” Medical Image Analysis, vol. 11, no. 6, pp. 555-566, 2007.
[16] G. Quellec, M. Lamard, P. M. Josselin, G. Cazuguel, B. Cochener, C. Roux, “Optimal wavelet transform for the detection of microaneurysms in retina photographs,” IEEE Transactions on Medical Imaging, vol. 27, no. 9, pp. 1230-41, 2008.
[17] K. Zuiderveld, “Contrast Limited Adaptive Histogram Equalization,” Graphics Gems, pp. 474-485, 1994.
[18] S. Roychowdhury, D. D. Koozekanani, and K. K. Parhi, “Screening fundus images for diabetic retinopathy,” in 2012 Conference Record of the Forty Sixth Asilomar Conference on Signals, systems and Computers (ASILOMAR), 2012, pp. 1641–1645.
[19] A. Frame, P. Undrill, M. Cree, J. Olson, K. McHardy, P. Sharp, and J. Forrester, “A comparison of computer based classication methods applied to the detection of microaneurysms in ophthalmic uorescein angiograms,” Comput. Biol. Med., vol. 28, pp. 225–238, 1998.
[20] B. C. Moore, “Principal component analysis in linear systems: controllability, observability, and model reduction,” Automatic Control IEEE Transactions on, vol. 26, no. 1, pp. 17-32, 1981.
[21] S. Valle, A. Weihua Li, S. J. Qin, “Selection of the number of principal components: the variance of the reconstruction error criterion with a comparison to other methods†,” Industrial & Engineering Chemistry Research, vol. 38, no. 11, pp. 653-658, 1999.
[22] I. T. Jolliffe, N. T. Trendafilov, M. Uddin, “A modified principal component technique based on the lasso,” Journal of Computational & Graphical Statistics, vol. 12, no. 3, pp. 531-547, 2003.
[23] B. Efron, T. Hastie, I. Johnstone, R. Tibshirani, “Least angle regression,” Mathematic, vol. 32, no. 2, pp. 2004, 2004.
[24] A. Bhalerao, A. Patanaik, S. Anand, and P. Saravanan, “Robust detection of microaneurysms for sight threatening retinopathy screening,” in Sixth Indian Conference on Computer Vision, Graphics Image Processing, 2008. ICVGIP ’08, Dec. 2008, pp. 520 –527.
[25] Sohini Roychowdhury, Dara D. Koozekanani and Keshab K.,’DREAM: Diabetic Retinopathy Analysis using Machine Learning’, IEEE Journal of Biomedical and Health Informatics 2013.
[26] A. Sopharak, B. Uyyanonvara, S. Barman, and T. H. Williamson, “Automatic detection of diabetic retinopathy exudates from non-dilated retinal images using mathematical morphology methods,” Computerized Medical Imaging and Graphics, vol. 32, no. 8, pp. 720 – 727, 2008.
[27] T. Walter, J.-C. Klein, P. Massin, and A. Erginay, “A contribution of image processing to the diagnosis of diabetic retinopathy-detection of exudates in color fundus images of the human retina,” IEEE Transactions on Medical Imaging, vol. 21, no. 10, pp. 1236 –1243, Oct. 2002.
[28] D. Welfer, J. Scharcanski, and D. R. Marinho, “A coarse-to-fine strategy for automatically detecting exudates in color eye fundus images,” Computerized Medical Imaging and Graphics, vol. 34, no. 3, pp. 228 – 235, 2010.
[29] Ragav Venkatesan, Parag Chandakkar, Baoxin,’’Classification of Diabetic Retinopathy Images Using Multi-Class Multiple-Instance Learning Based on Color Correlogram features”,IEEE Engineering in Medicine and Biology Society (EMBC), 2012 Annual International Conference of the IEEE 2012.
[30] Mohamed Omar,Fouad Khelifi and Muhammad Atif Tahir,’’Detection and Classification of Retinal Fundus Images Exudates using Region based Multiscale LBP Texture Approach”,IEEE, International Conference on Control, Decision and Information Technologies (CoDIT), 2016.