Credit Risk Management through Big Data Analytics
Deepika Sharma1
Section:Research Paper, Product Type: Journal Paper
Volume-06 ,
Issue-05 , Page no. 14-18, Jun-2018
Online published on Jun 30, 2018
Copyright © Deepika Sharma . This is an open access article distributed under the Creative Commons Attribution License, which permits unrestricted use, distribution, and reproduction in any medium, provided the original work is properly cited.
View this paper at Google Scholar | DPI Digital Library
How to Cite this Paper
- IEEE Citation
- MLA Citation
- APA Citation
- BibTex Citation
- RIS Citation
IEEE Style Citation: Deepika Sharma , “Credit Risk Management through Big Data Analytics,” International Journal of Computer Sciences and Engineering, Vol.06, Issue.05, pp.14-18, 2018.
MLA Style Citation: Deepika Sharma "Credit Risk Management through Big Data Analytics." International Journal of Computer Sciences and Engineering 06.05 (2018): 14-18.
APA Style Citation: Deepika Sharma , (2018). Credit Risk Management through Big Data Analytics. International Journal of Computer Sciences and Engineering, 06(05), 14-18.
BibTex Style Citation:
@article{Sharma_2018,
author = {Deepika Sharma },
title = {Credit Risk Management through Big Data Analytics},
journal = {International Journal of Computer Sciences and Engineering},
issue_date = {6 2018},
volume = {06},
Issue = {05},
month = {6},
year = {2018},
issn = {2347-2693},
pages = {14-18},
url = {https://www.ijcseonline.org/full_spl_paper_view.php?paper_id=413},
publisher = {IJCSE, Indore, INDIA},
}
RIS Style Citation:
TY - JOUR
UR - https://www.ijcseonline.org/full_spl_paper_view.php?paper_id=413
TI - Credit Risk Management through Big Data Analytics
T2 - International Journal of Computer Sciences and Engineering
AU - Deepika Sharma
PY - 2018
DA - 2018/06/30
PB - IJCSE, Indore, INDIA
SP - 14-18
IS - 05
VL - 06
SN - 2347-2693
ER -
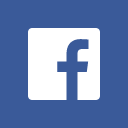
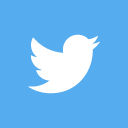
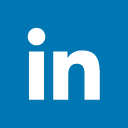
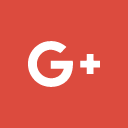
Abstract
Credit risk remains till date one of the biggest and most challenging issue in the lending financial institutions. Credit risk refers to the probability of default which may occur if the liabilities are not fulfilled under the terms of the contract, resulting into the loss of the financial institution or banks (the creditor). Difficulties in credit risk management arise because the credit default occurs mostly, unexpectedly. The databases of the banks around the world have accumulated large quantities of information about clients and their financial and credit history. These databases can be used for the credit risk assessment, but they are generally high dimensional and traditional data analytics may not be able to handle such large volume of high dimensional data. How to develop a high-performance platform to efficiently analyze the Big Data Analytics (BDA) that can lead to better and more informed credit decisions? This study seeks to answer this question by discussing a macroscopic view of emerging Big Data techniques for addressing the vital issues of credit risk across the various sectors of finance and aim to identify the suitable BDA tools for the purpose of managing Credit Risk.
Key-Words / Index Term
Credit, Big, Risk, Hadoop, Finance
References
[1] Baliga, J., Ayre, R.W., Hinton, K. and Tucker, R.S., 2011. Green cloud computing: Balancing energy in processing, storage, and transport. Proceedings of the IEEE, 99(1), pp.149-167.
[2] Begoli, E., & Horey, J. (2012). Design principles for effective knowledge discovery from big data. Software architecture (WICSA) and european conference on software architecture (ECSA), 2012 joint working IEEE/IFIP conference on (pp. 215-218). IEEE.
[3] Begoli, E., & Horey, J. (2012). Design principles for effective knowledge discovery from big data. Software architecture (WICSA) and european conference on software architecture (ECSA), 2012 joint working IEEE/IFIP conference on (pp. 215-218). IEEE..
[4] Chen, Y., Chen, H., Gorkhali, A., Lu, Y., Ma, Y. and Li, L., 2016. Big data analytics and big data science: a survey. Journal of Management Analytics, 3(1), pp.1-42.
[5] Dragosavac,(2015)”Big Data Analytics for LendersandCreditors” https://www.kdnuggets.com/2015/10/big-data-analytics-lenders-creditors.
[6] Eccles, R., Herz, R., Keegan, M. and Phillips, D. (2001), “The risk of risk”, Balance Sheet, Vol. 9No. 3, pp. 28-33.energy in processing, storage, and transport, Proc. IEEE 99 (1) (2011) 149–167.
[7] Fatemi, A. and Fooladi, I. (2006), “Credit risk management: a survey of practices”, Managerial Finance, Vol. 32 No. 3, pp. 227-233.
[8] Flood, M.D., Jagadish, H.V. and Raschid, L., 2016. Big data challenges and opportunities in financial stability monitoring. Banque de France, Financial Stability Review, 20.
[9] Katal, A., Wazid, M. and Goudar, R.H., 2013, August. Big data: issues, challenges, tools and good practices. In Contemporary Computing (IC3), 2013 Sixth International Conference on (pp. 404-409). IEEE.
[10] Klieštik, T. and Cúg, J., 2015. Comparison of selected models of credit risk. Procedia Economics and Finance, 23, pp.356-361.
[11] Lee, T.S., Chiu, C.C., Lu, C.J. and Chen, I.F., 2002. Credit scoring using the hybrid neural discriminant technique. Expert Systems with applications, 23(3), pp.245-254.
[12] Li, Y., Chen, W., Wang, Y., & Zhang, Z. L. (2013). Influence diffusion dynamics and influence maximization in social networks with friend and foe relationships. In Proceedings of the sixth ACM international conference on Web search and data mining (pp. 657–666). ACM.
[13] Lin Z, Whinston AB, Fan S (2015) Harnessing Internet finance with innovative cyber credit management. Financial Innovation, 1(1), p.5.
[14] Lin, J. and Dyer, C., 2010. Data-intensive text processing with MapReduce. Synthesis Lectures on Human Language Technologies, 3(1), pp.1-177.
[15] Mashanovich,(2017)”Using Big Data and Predictive Analytics for Credit Scoring” https://dzone.com/articles/using-big-data-and-predictive-analytics-for-credit.
[16] Najafabadi, M.M., Villanustre, F., Khoshgoftaar, T.M., Seliya, N., Wald R.,Muharemagic, E., 2015a. Deep learning applications and challenges in big data analytics. Journal of Big Data, 2(1), p.1.
[17] Oreski, S., Oreski, D. and Oreski, G., 2012. Hybrid system with genetic algorithm and artificial neural networks and its application to retail credit risk assessment. Expert systems with applications, 39(16), pp.12605-12617.
[18] Oussous, A., Benjelloun, F.Z., Lahcen, A.A. and Belfkih, S., 2017. Big Data technologies: A survey. Journal of King Saud University-Computer and Information Sciences.
[19] Prekopcsak, Z., Makrai, G., Henk, T. and Gaspar-Papanek, C., 2011, June. Radoop: Analyzing big data with rapidminer and hadoop. In Proceedings of the 2nd RapidMiner community meeting and conference (RCOMM 2011) (pp. 1-12).
[20] Shvachko, K., Kuang, H., Radia, S. and Chansler, R., 2010, May. The hadoop distributed file system. In Mass storage systems and technologies (MSST), 2010 IEEE 26th symposium on (pp. 1-10). IEEE.
[21] Siddiqa, A., Hashem, I.A.T., Yaqoob, I., Marjani, M., Shamshirband, S., Gani, A. and Nasaruddin, F., 2016. A survey of big data management: Taxonomy and state-of-the-art. Journal of Network and Computer Applications, 71, pp.151-166.
[22] Singh, D. and Reddy, C.K., 2015. A survey on platforms for big data analytics. Journal of Big Data, 2(1), p.8.
[23] Spuchľaková, E., & Cúg, J. (2014). Lost Given Default and the Credit Risk. Proceedings of ICMEBIS 2014 International Conference on Management, Education, Business, and Information Science, Shanghai, China, EDUGait Press, Canada (pp. 12-15).
[24] Srinivasan, S. and Kamalakannan, T., 2017. Multi Criteria Decision Making in Financial Risk Management with a Multi-objective Genetic Algorithm. Computational Economics, pp.1-15.
[25] Terzi, D.S., Terzi, R. and Sagiroglu, S., 2015, December. A survey on security and privacy issues in big data. In Internet Technology and Secured Transactions (ICITST), 2015 10th International Conference for (pp. 202-207). IEEE.
[26] Thusoo, A., Sarma, J. S., Jain, N., Shao, Z., Chakka, P., Anthony, S., et al. (2009). Hive:a warehousing solution over a map-reduce framework. Proceedings of the VLDBEndowment, 2(2), 1626–1629.
[27] Uddin, M.F. and Gupta, N., 2014, April. Seven V`s of Big Data understanding Big Data to extract value. In American Society for Engineering Education (ASEE Zone 1), 2014 Zone 1 Conference of the (pp. 1-5). IEEE .Vol. 25 Issue: 4, pp.422-434.
[28] White, T., 2009. Hadoop: the definitive guide: the definitive guide:“O’Reilly Media, Inc.”.