Programmed Face Learning To Name Discriminative Fondness Matrices From Weakly Labeled Images
Dr Naghabhushan1 , Dr Aravinda T V2 , Arpitha H S3
Section:Review Paper, Product Type: Conference Paper
Volume-04 ,
Issue-03 , Page no. 58-61, May-2016
Online published on Jun 07, 2016
Copyright © Dr Naghabhushan , Dr Aravinda T V , Arpitha H S . This is an open access article distributed under the Creative Commons Attribution License, which permits unrestricted use, distribution, and reproduction in any medium, provided the original work is properly cited.
View this paper at Google Scholar | DPI Digital Library
How to Cite this Paper
- IEEE Citation
- MLA Citation
- APA Citation
- BibTex Citation
- RIS Citation
IEEE Style Citation: Dr Naghabhushan , Dr Aravinda T V , Arpitha H S , “Programmed Face Learning To Name Discriminative Fondness Matrices From Weakly Labeled Images,” International Journal of Computer Sciences and Engineering, Vol.04, Issue.03, pp.58-61, 2016.
MLA Style Citation: Dr Naghabhushan , Dr Aravinda T V , Arpitha H S "Programmed Face Learning To Name Discriminative Fondness Matrices From Weakly Labeled Images." International Journal of Computer Sciences and Engineering 04.03 (2016): 58-61.
APA Style Citation: Dr Naghabhushan , Dr Aravinda T V , Arpitha H S , (2016). Programmed Face Learning To Name Discriminative Fondness Matrices From Weakly Labeled Images. International Journal of Computer Sciences and Engineering, 04(03), 58-61.
BibTex Style Citation:
@article{Naghabhushan_2016,
author = {Dr Naghabhushan , Dr Aravinda T V , Arpitha H S },
title = {Programmed Face Learning To Name Discriminative Fondness Matrices From Weakly Labeled Images},
journal = {International Journal of Computer Sciences and Engineering},
issue_date = {5 2016},
volume = {04},
Issue = {03},
month = {5},
year = {2016},
issn = {2347-2693},
pages = {58-61},
url = {https://www.ijcseonline.org/full_spl_paper_view.php?paper_id=63},
publisher = {IJCSE, Indore, INDIA},
}
RIS Style Citation:
TY - JOUR
UR - https://www.ijcseonline.org/full_spl_paper_view.php?paper_id=63
TI - Programmed Face Learning To Name Discriminative Fondness Matrices From Weakly Labeled Images
T2 - International Journal of Computer Sciences and Engineering
AU - Dr Naghabhushan , Dr Aravinda T V , Arpitha H S
PY - 2016
DA - 2016/06/07
PB - IJCSE, Indore, INDIA
SP - 58-61
IS - 03
VL - 04
SN - 2347-2693
ER -
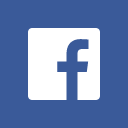
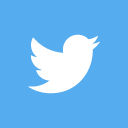
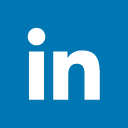
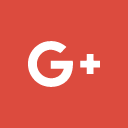
Abstract
In video or image so many faces will be present. Each name is associated with some names in the corresponding caption. The goal of this project is naming the faces with the correct names. This application used in Face book, Flicker and some news websites like NDTV,TV9 etc…To generate these type of application earlier they using a method like detect the face first and give label to it give name to it. Here dataset are more. To solve this problem here proposing two new methods by learning two discriminative affinity matrices from these Weakly labeled images. First method is regularized low-rank representation by effectively utilizing Weakly supervised information to learn a low-rank reconstruction coefficient matrix while exploring multiple subspace structures of the data. In this method they reducing dataset by taking a training images and converted into affinity matrices. After generating affinity matrices they are using low rank representation method. After generating this low rank representation they provide labeling for the images by using subspace structures. After creating subspace structures generate a affinity matrices. Second method is called ambiguously supervised structural metric learning by using weakly supervised information to seek a discriminative distance metric. This method is used to calculate the distances between the pixels in the image by using mahalanobis distances of data. After calculating the distances it going to create some of the clusters. It is used to create a boundary and also give the features of the faces. These faces will be get in matrix form. From this face we recognizing the correct name for it.
Key-Words / Index Term
matrix, caption-based face naming, distance metric learning, low-rank representation(LRR
References
[1] P. Viola and M. J. Jones, “Robust real-time face detection,” Int.J. Comput. Vis., vol. 57, no. 2, pp. 137–154, 2004.
[2] G. Liu, Z. Lin, and Y. Yu, “Robust subspace segmentation by low-rank representation,” in Proc. 27th Int. Conf. Mach. Learn., Haifa, Israel, Jun. 2010, pp. 663–670.
[3] T. L. Berg et al., “Names and faces in the news,” in Proc. 17th IEEE Comput. Soc. Conf. Comput. Vis. Pattern Recognit., Washington, DC, USA, Jun./Jul. 2004, pp. II-848–II-854.
[4] D. Ozkan and P. Duygulu, “A graph based approach for naming faces in news photos,” in Proc. 19th IEEE Comput. Soc. Conf. Comput. Vis. Pattern Recognit., New York, NY, USA, Jun. 2006, pp. 1477–1482.
[5] M. Everingham, J. Sivic, and A. Zisserman, “Hello! My name is... Buffy—Automatic naming of characters in TV video,” in Proc. 17th Brit Mach. Vis. Conf., Edinburgh, U.K., Sep. 2006, pp. 899–908.
[6] Z. Zeng et al., “Learning by associating ambiguously labeled images,”in Proc. 26th IEEE Conf. Comput. Vis. Pattern Recognit., Portland, OR,USA, Jun. 2013, pp. 708–715.